## 视频
<video src="https://dev-media.amazoncloud.cn/30-LibaiGenerate/31-LiBaiRebrandingVideo/IMP204-Mental_health_crisis_intervention_based_on_analytics_and_ML-LBrebrandingWCaptionCN.mp4" class="bytemdVideo" controls="controls"></video>
## 导读
准确及时的洞察力可以挽救生命、提高公平性,甚至有助于增加非营利组织所依赖的关键捐款。在本论坛中,向非营利组织学习如何使用[机器学习](https://aws.amazon.com/cn/machine-learning/?trk=cndc-detail)算法、自然语言处理和实体提取,构建自杀前行为的模型,揭示有助于大规模拯救生命的新见解。此外,请听一家非营利媒体组织介绍,如何在湖畔小屋中整合数据源,并使用分析和 ML 帮助他们分析收视率趋势,以更好地服务社区,并为捐助者建立有针对性的活动。一位亚马逊云科技专家深入探讨了这些有影响力的解决方案背后的原因。
## 演讲精华
<font color = "grey">以下是小编为您整理的本次演讲的精华,共1800字,阅读时间大约是9分钟。如果您想进一步了解演讲内容或者观看演讲全文,请观看演讲完整视频或者下面的演讲原文。</font>
这个富有洞见的视频探讨了两个非营利组织——PBS和Stop Soldier Suicide——如何运用亚马逊云科技的分析与[机器学习](https://aws.amazon.com/cn/machine-learning/?trk=cndc-detail)服务来产生影响力,从而推动数字化变革。他们的成功案例为非营利组织如何实现数据驱动并扩大影响提供了范例。
首先,我们听取了PBS高级产品经理Haneef的分享。他解释说,PBS通过提供教育、信息和启发性内容,已为美国公众服务了50多年。与商业媒体不同,PBS是一个非营利会员组织,为美国的330个会员台服务,覆盖美国人口的96%。
尽管PBS的核心使命多年来一直保持稳定,但数字技术已极大地改变了媒体环境。为了适应这些变化,PBS意识到他们需要采用数字化转型策略,并利用数据分析。他们已经与亚马逊云科技展开合作,建立一个基于云端的数据平台,提供更丰富的个性化体验,降低运营成本,并挖掘数据驱动的洞察。
PBS正在使用亚马逊S3、亚马逊云科技Lake Formation和[Amazon AppFlow](https://aws.amazon.com/cn/appflow/?trk=cndc-detail)等服务,将来自各种来源的内容和客户数据整合到中央数据湖中。这使得他们将零散的数据源整合成统一的单一来源成为可能。
PBS还在使用亚马逊Glue ETL作业来转换和编目数据,以便更易于进行查询。亚马逊Glue数据目录为一个描述S3中数据的一致化元数据存储提供了支持。
在分析方面,PBS正在使用亚马逊Athena对其数据湖中的结构化数据进行查询。这使他们能够分析观众行为,从而发掘有价值的见解。
PBS还在使用亚马逊Personalize构建一个视频推荐原型。Personalize的[机器学习](https://aws.amazon.com/cn/machine-learning/?trk=cndc-detail)功能使得PBS能够分析用户的观看历史,并为每位用户提供定制的视频集。
此外,PBS的创新团队还在尝试使用亚马逊Kendra和[Amazon Textract](https://aws.amazon.com/cn/textract/?trk=cndc-detail)等亚马逊云科技服务,以探索自动化生成视频字幕和标题的使用场景。
然而,PBS在早期数据处理过程中也遭遇了一定的挑战。首先,客户数据分散在多个独立的系统中,导致他们对观众的认知不全面。由于缺乏统一的真实来源,不同的团队可能会使用过时的Excel报告作为决策依据。
Secondly, early data projects were designed for single, narrow purposes, leading to limited reuse and repetitive work when other teams wanted to use the data for new initiatives. Thirdly, launching new data projects was expensive, as attention was focused on simply integrating data from different sources rather than solving business problems. Data teams spent more time integrating data than extracting value from it. Lastly, without appropriate data governance, employees found it difficult to locate, access, and share data throughout the organization.
To address these issues, PBS adopted a five-stage data maturity model to guide its analytical journey:
1. Manual Data Triage - Dependent on Excel attachments and manual processes
2. Trapped by Dashboards - Struggling with excessive visualizations and real-time information sources
3. Data Integration - Adopting a more controlled approach with consistent information
4. Emerging Intelligence - Beginning to leverage AI and ML technologies
5. AI/ML Becomes Reality - Building a strong data foundation to support advanced analysis
When they began their journey, PBS realized that they were stuck between the first and third stages. Their goal was to enter the fourth and fifth stages by building a unified and managed data lakehouse on Amazon Web Services. This data lakehouse would integrate their various data sources into a flexible, centralized repository. They hoped this would help them discover new insights, achieve cross-departmental data access democratization, and improve their overall data maturity.
PBS has already started to realize tangible benefits from their early data lakehouse initiatives:
- PBS Recommendation Engine: This prototype uses [Amazon Personalize](https://aws.amazon.com/cn/personalize/?trk=cndc-detail) to provide audiences with personalized content collections. Compared to manually curated lists, it has increased weekly engagement by 158%.
- Generative AI Exploration: PBS's innovation team is attempting to use OpenAI's generative AI models to create metadata, such as subtitles and titles. This saves substantial time compared to manual processes. PBS plans to achieve this through working alongside services like [Amazon Kendra](https://aws.amazon.com/cn/kendra/?trk=cndc-detail).
- Data Democratization: The data lakehouse is helping PBS share data with local radio stations for various initiatives, ranging from donor analysis to personalized audience experiences. As their iterations progress, they will be able to invest more effectively and become a more data-driven organization.
根据他们的经验,PBS为刚开始数据分析之旅的组织分享了三个最重要的经验教训:
1. 获得组织上的承诺——提高数据成熟度需要时间,因此要与利益相关者设定现实的目标。
2. 有明确的“为什么”——制定与业务目标一致的数据策略。考虑是否一个单一的使用场景值得建立一个数据集市而不是完整的湖仓。
3. 关注数据质量、治理和隐私——好的数据可以训练模型,但治理使得责任使用得以实现。
接下来,我们听取了Glenn,Stop Soldier Suicide创始人兼CTO的介绍。Stop Soldier Suicide为军人和服务退伍军人提供支持和危机干预资源。截至目前,他们已经服务了超过1400个客户,提供了超过700小时的照顾,并且年度需求同比增长了23%。然而,他们服务的需求仍在不断增加。大多数客户是通过数字营销和在线广告获得的。这使Stop Soldier Suicide开始探索如何利用数据和技术来推进其使命。
Black Box项目始于2019年,目的是揭示新的防止退伍军人自杀的方法。Glenn注意到他的自杀朋友中的一个模式——在他们去世之前,他们经常在社交媒体上发布令人担忧的消息。这表明他们的设备中包含有关他们行为和心态的有价值见解。
在几个月内,Black Box团队从68个因自杀失去亲人的家庭那里获得了78部手机和其他设备。他们与亚马逊的[机器学习](https://aws.amazon.com/cn/machine-learning/?trk=cndc-detail)专家合作分析这些设备上的数据。
具体来说,Black Box项目使用亚马逊云科技的服务,如[Amazon S3](https://aws.amazon.com/cn/s3/?trk=cndc-detail)和Amazon Cloud Glue,从移动设备中摄取和处理数据。[Amazon Comprehend](https://aws.amazon.com/cn/comprehend/?trk=cndc-detail)用于对文本消息和社交媒体帖子进行情感分析,以将“好天”与“坏天”进行分类。[Amazon Kendra](https://aws.amazon.com/cn/kendra/?trk=cndc-detail)提供语义搜索功能,以识别与自杀念头相关的关键短语和模式。该团队使用亚马逊 SageMaker构建自定义[机器学习](https://aws.amazon.com/cn/machine-learning/?trk=cndc-detail)模型,以分析诸如基于电话使用和应用程序活动的睡眠安排等因素。
该项目已经发现了一些重要发现:
- 对文本消息的情感分析,将“好天”和“坏天”根据表达的情绪进行分类。坏天看到愤怒、恐惧和悲伤的增加。
语义搜索工具提取了与自杀观念、寻求帮助、药物滥用和经济困难有关的关键短语。通过利用手机使用数据,定制算法在自杀前识别出了睡眠剥夺趋势。通常而言,在糟糕的日子里,受试者平均比在好日子少睡约3个小时。将这些信号结合起来发现,在死亡之前,孤立、恐惧、愤怒、睡眠剥夺和自杀风险都在上升。掌握这些行为洞察后,阻止士兵自杀计划改善了对高风险退伍军的定位策略。他们的下一步包括:将所学知识应用于呼叫中心以监控客户和员工,开发一个SDK来标记第三方应用程序中的高风险个人,以及创建一个实时追踪客户健康状况的个性化应用。这将持续监测警告迹象并触发警报。他们将继续提高预测能力,因为数据越多,算法就越准确。黑盒项目大量依赖于亚马逊云科技服务,如用于情感分析的[Amazon Comprehend](https://aws.amazon.com/cn/comprehend/?trk=cndc-detail)、用于语义搜索的[Amazon Kendra](https://aws.amazon.com/cn/kendra/?trk=cndc-detail)以及用于构建自定义[机器学习](https://aws.amazon.com/cn/machine-learning/?trk=cndc-detail)模型的[Amazon SageMaker](https://aws.amazon.com/cn/sagemaker/?trk=cndc-detail)。Glenn强调,没有亚马逊云科技的合作和支持,这个项目就不可能存在。他们与亚马逊云科技的非营利组织、亚马逊ML解决方案实验室和ProServe等团队的合作伙伴关系对他们的成功至关重要。在未来的部分中,亚马逊云科技的演讲者将探讨如何将PBS和阻止士兵自杀的经验应用于亚马逊云科技的数据分析和[机器学习](https://aws.amazon.com/cn/machine-learning/?trk=cndc-detail)路线图。她提供一个常见挑战的例子:评估程序有效性时,数据位于隔离源中。这可能包括一个包含参与者基本信息的项目数据库和一个单独的收集反馈的调查工具。通过将这些来源整合到[Amazon S3](https://aws.amazon.com/cn/s3/?trk=cndc-detail)上的数据湖中,使得分析变得更加简单。演讲者概述了一个高级别的现代数据分析管道视图,该管道利用亚马逊云科技:
1. 将数据从各种来源导入至[Amazon S3](https://aws.amazon.com/cn/s3/?trk=cndc-detail),这是一个灵活且持久的数据湖。可选方法包括AppFlow、数据库迁移服务、Kinesis和数据交换。
2. 使用亚马逊云科技Glue进行ETL处理和转换数据,清理并组织它。使用亚马逊云科技Glue数据目录对数据进行编目。
3. Utilizing Athena for data analysis and querying or using [Amazon QuickSight](https://aws.amazon.com/cn/quicksight/?trk=cndc-detail) for visualization. AI/ML capabilities can be applied through services like Comprehend.
4. Sharing insights within the organization using Amazon Web Services (亚马逊云科技) Data Exchange.
This pipeline has been intentionally designed to be flexible. As business needs change, organizations can add new data sources, analytical capabilities, and consumption channels.
Initially, speakers recommend following a five-step approach:
1. Identify an important but non-emergency business issue to address. Seek a "quick win" to demonstrate value.
2. Determine the data sources necessary to solve this issue. Gradually populate the data lake.
3. Run analyses to generate insights that address the issue. Leverage services such as Athena, QuickSight, and SageMaker.
4. Take action based on these insights.
5. Measure the consistency of results with the original objectives. Adjust and iterate as needed.
Starting with targeted use cases avoids overwhelm. As the data team gains experience with the process, they can scale to more complex and critical tasks.
Speakers emphasize that Amazon Web Services provides numerous resources to help organizations succeed in data analytics, including:
- Architecture design meetings
- Strategic planning workshops
- Hands-on assistance with concept validation
- Training and knowledge transfer
Ultimately, it is crucial to engage with your Amazon Web Services account manager to start a conversation and unlock the potential of AWS as a strategic data partner.
In summary, this insightful video showcases how PBS and Stop Soldier Suicide utilize 亚马逊云科技's analytics and machine learning services to achieve digital transformation and impact. Their story provides a blueprint for non-profit organizations to become data-driven entities on 亚马逊云科技. While the technology is powerful, success relies on selecting targeted business issues, embracing continuous improvement, and leveraging guidance from AWS's experienced team. Any organization can begin their data maturity journey and unlock new levels of intelligence and influence through this approach.
**下面是一些演讲现场的精彩瞬间:**
美国公共广播公司(PBS),作为一家非营利性的会员电台,已为全美民众提供了超过50年的教育、信息和启发内容。
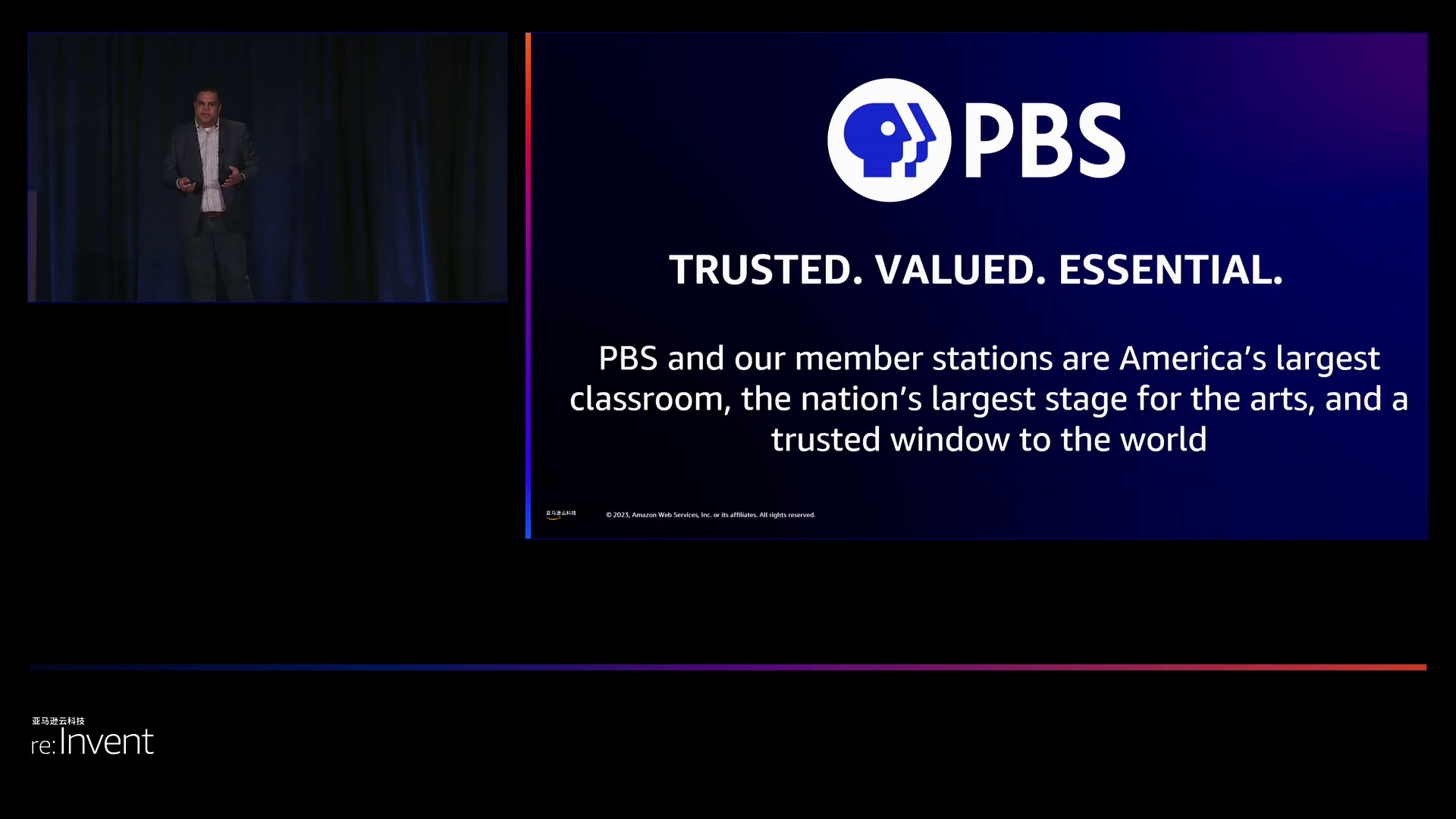
黑盒项目的目标在于研究网络攻击发生前的关键时刻,以揭示潜在的安全漏洞。
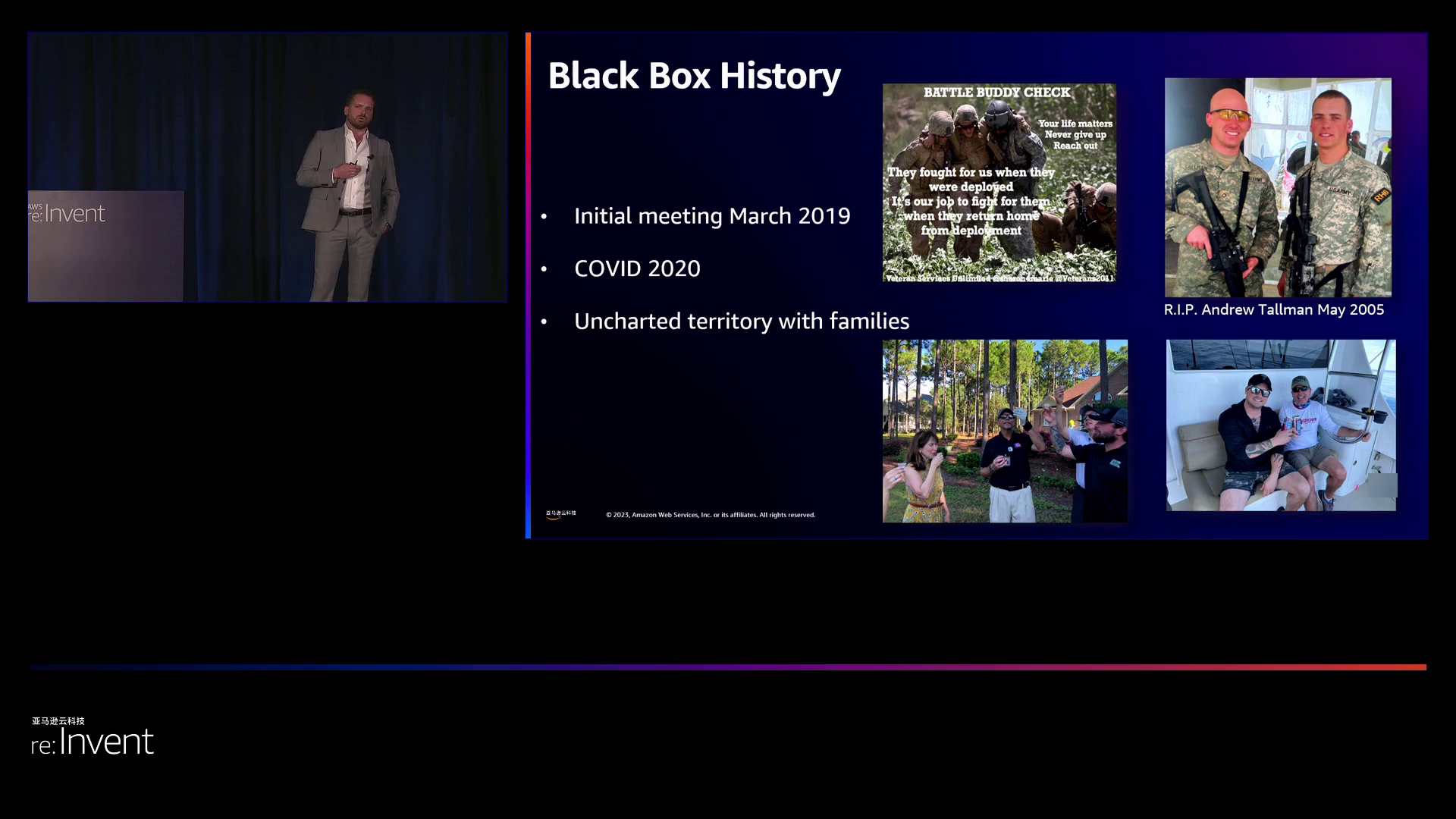
这种方式类似于航空事故中使用的飞行记录器,用于分析关键信息。
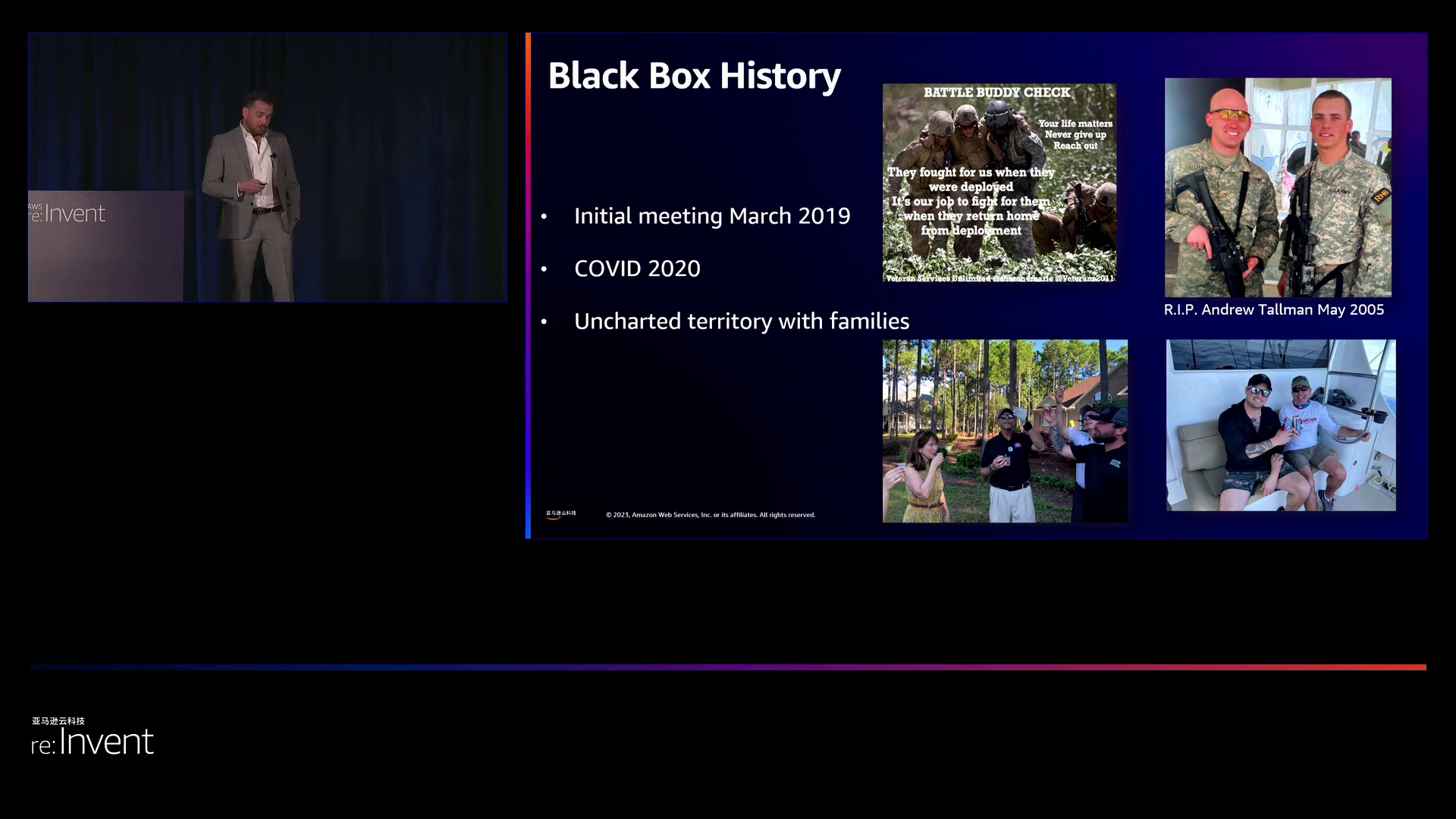
领导者们与社区成员保持着紧密的合作关系,他们提供各种设备,共同创造一个预防自杀的系统,就像一个家庭一样。
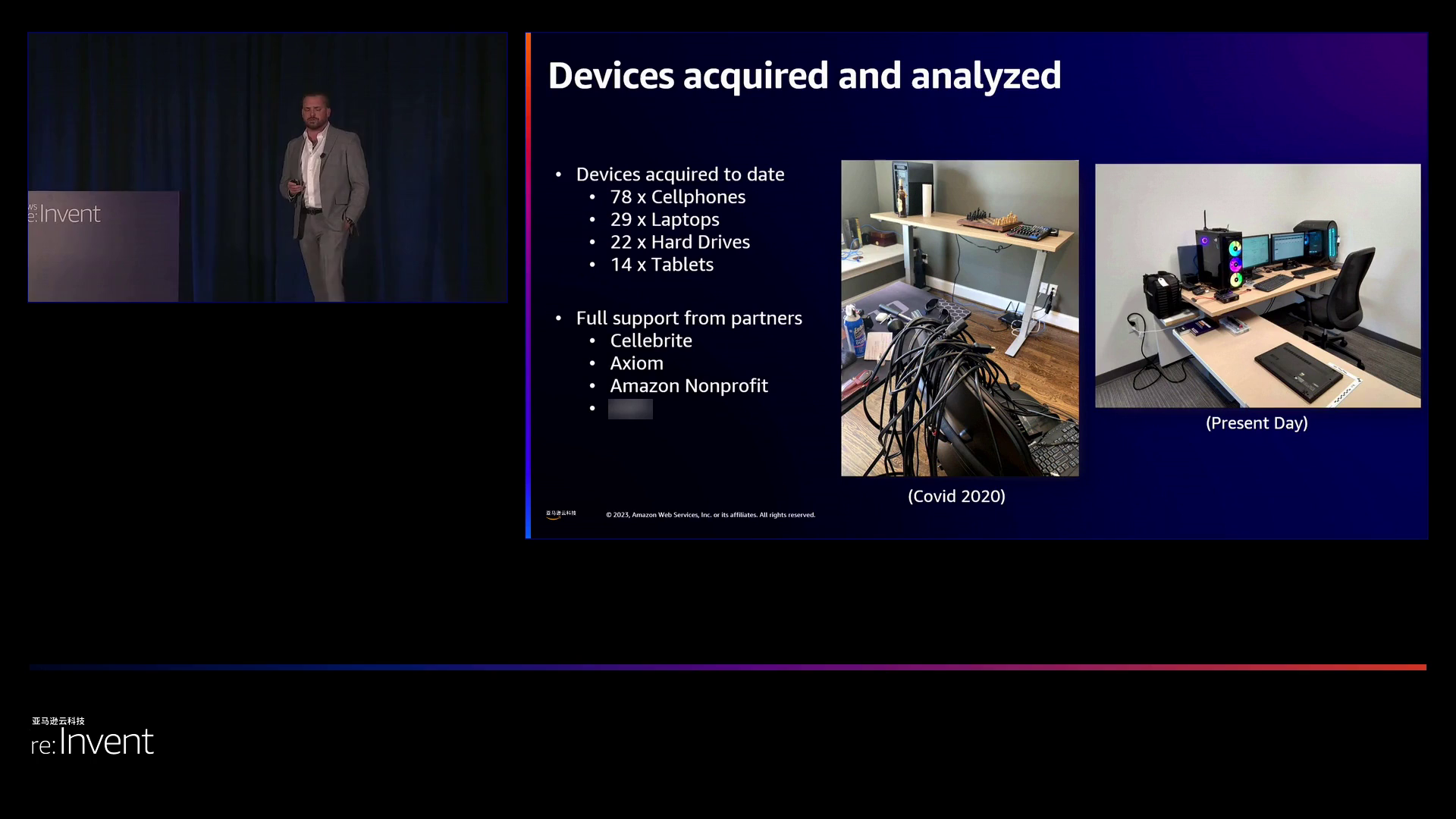
亚马逊云科技慷慨地捐赠了软件和资源,以协助建立一座实验室内测犯罪侦查设备的可行性。
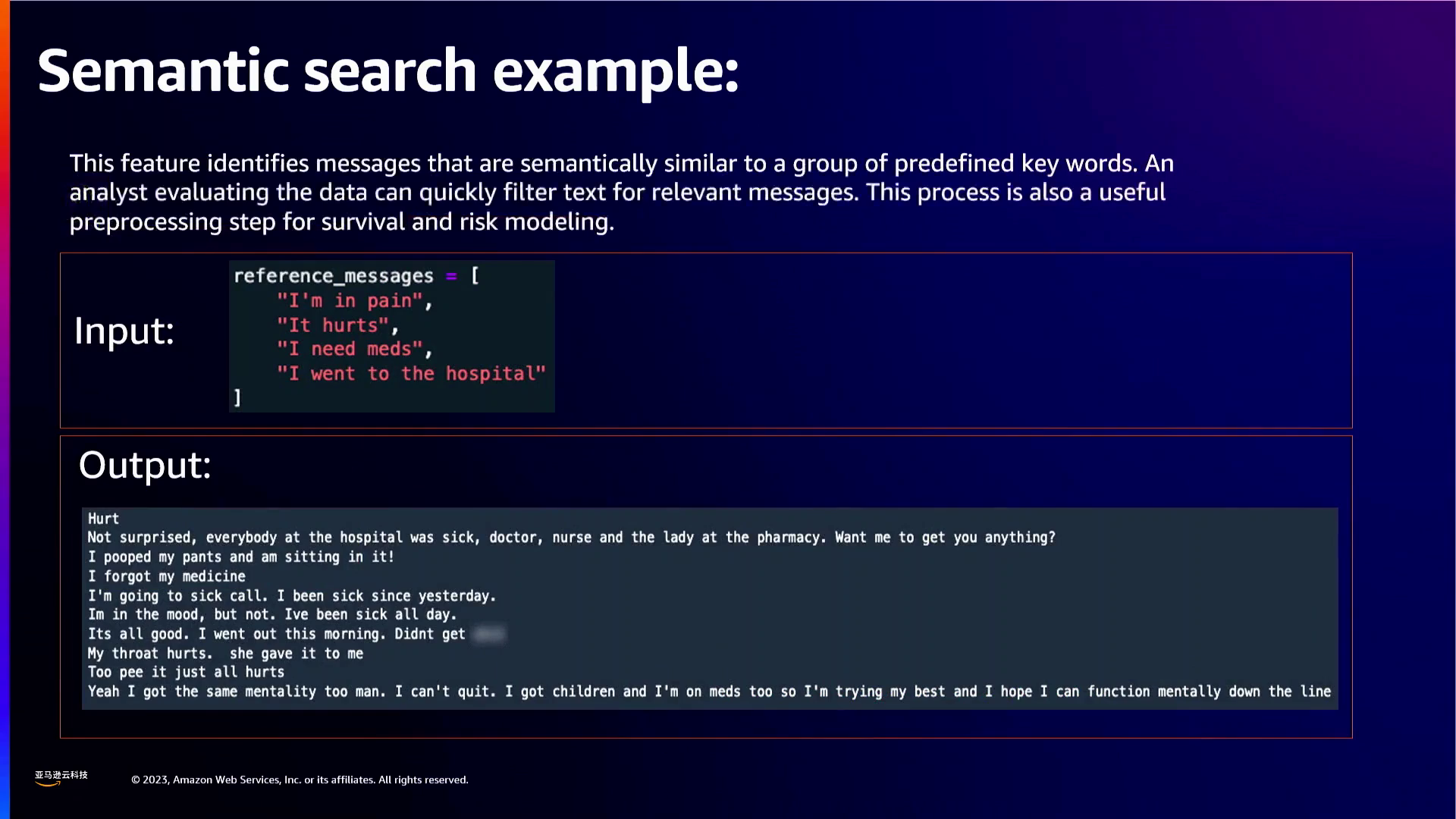
领导者们展示了如何运用亚马逊Comprehend技术分析文本,从而识别出痛苦的症状,以便及时给予帮助。
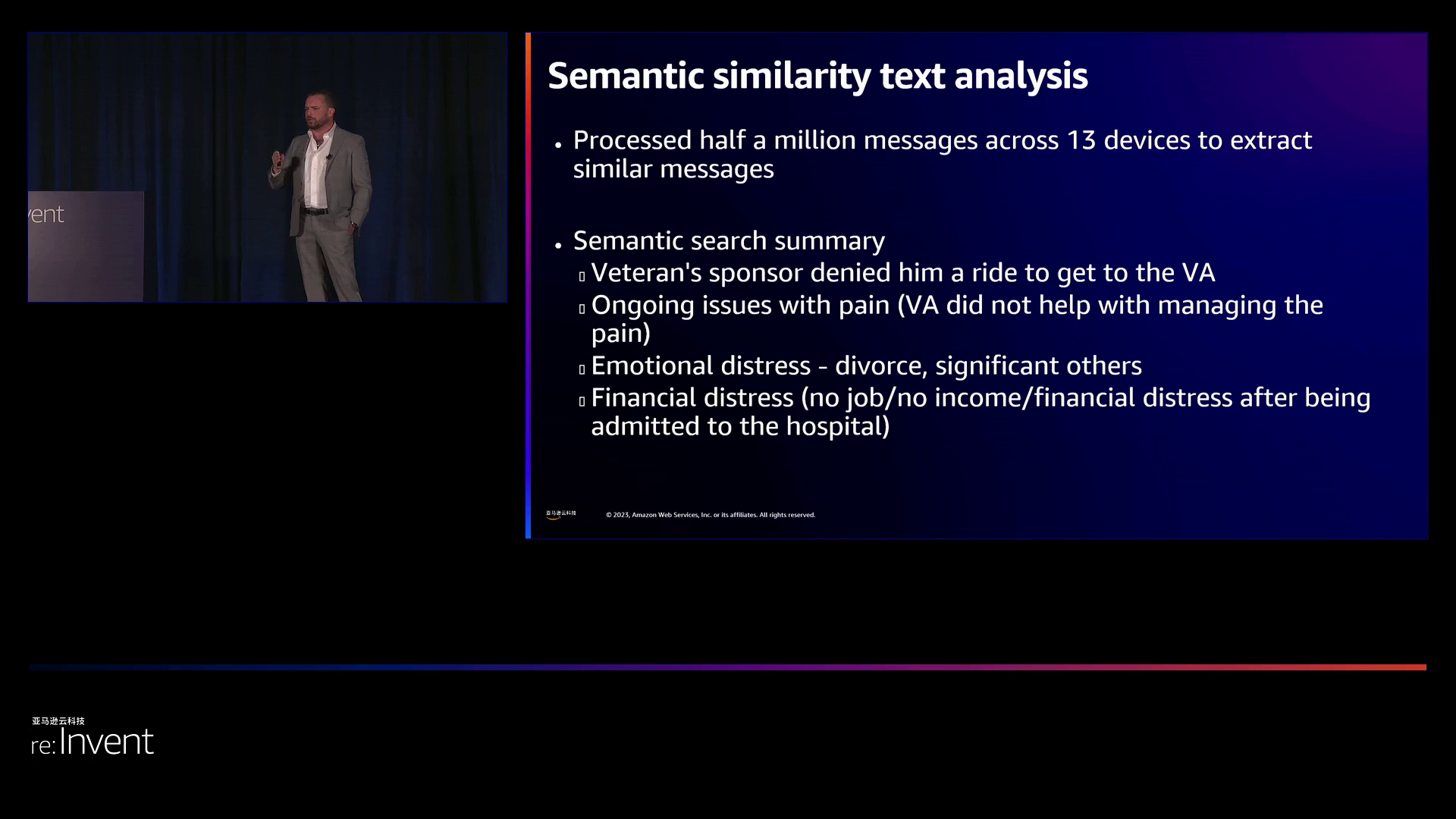
## 总结
The video discusses how PBS has leveraged Amazon Web Services' cloud and data analytics capabilities to transform from a broadcast organization to a multi-platform entity.
Facing data challenges including isolated systems and multiple information sources, resulting in incoherent audience perspectives, PBS sought to create a unified data lake on Amazon Web Services by integrating diverse data sources to uncover actionable insights. This data lake improved access to data across the entire organization and enabled use cases such as personalized recommendations.
PBS also began experimenting with AI/ML, using OpenAI for brand voice writing and action prompts in mobile applications. This created metadata and saved time. While specific use cases demonstrated value, the lake unlocked immense potential on a broader scale.
Key lessons include obtaining organizational commitment due to the time required to establish data infrastructure; having a clear "why" that aligns with business goals; and focusing on data quality, governance, and privacy.
The presentation continues with the Black Box Project, which aims to analyze digital forensic data of deceased veterans over the past year to prevent soldier suicides. Using their devices and AI/ML, the goal is to uncover patterns leading to suicide for predictive and preventive purposes. Key findings so far relate to sleep deprivation, emotional factors, isolation, and other factors.
Collaborating with Amazon Web Services, the project applies learning outcomes to suicide prevention applications like call center monitoring, SDKs for mental health apps, and personal support apps. This innovative data-driven approach aims to provide accountability and save lives.
In summary, through strategic data planning, PBS and the Black Box Project demonstrate how human-centered organizations can leverage cloud and AI capabilities for digital transformation and social impact.
## 演讲原文
## 想了解更多精彩完整内容吗?立即访问re:Invent 官网中文网站!
[2023亚马逊云科技re:Invent全球大会 - 官方网站](https://webinar.amazoncloud.cn/reInvent2023/?s=8739&smid=19458 "2023亚马逊云科技re:Invent全球大会 - 官方网站")
[点击此处](https://aws.amazon.com/cn/new/?trk=6dd7cc20-6afa-4abf-9359-2d6976ff9600&trk=cndc-detail "点击此处"),一键获取亚马逊云科技全球最新产品/服务资讯!
[点击此处](https://www.amazonaws.cn/new/?trk=2ab098aa-0793-48b1-85e6-a9d261bd8cd4&trk=cndc-detail "点击此处"),一键获取亚马逊云科技中国区最新产品/服务资讯!
## 即刻注册亚马逊云科技账户,开启云端之旅!
[【免费】亚马逊云科技“100 余种核心云服务产品免费试用”](https://aws.amazon.com/cn/campaigns/freecenter/?trk=f079813d-3a13-4a50-b67b-e31d930f36a4&sc_channel=el&trk=cndc-detail "【免费】亚马逊云科技“100 余种核心云服务产品免费试用“")
[【免费】亚马逊云科技中国区“40 余种核心云服务产品免费试用”](https://www.amazonaws.cn/campaign/CloudService/?trk=2cdb6245-f491-42bc-b931-c1693fe92be1&sc_channel=el&trk=cndc-detail "【免费】亚马逊云科技中国区“40 余种核心云服务产品免费试用“")