{"value":"The International Conference on Acoustics, Speech, and Signal Processing ([ICASSP](https://www.amazon.science/conferences-and-events/icassp-2021)) starts next week, and as Alexa principal research scientist Ariya Rastrow [explained last year](https://www.amazon.science/blog/icassp-what-signal-processing-has-come-to-mean), it casts a wide net. The topics of the 36 Amazon research papers at this year’s ICASSP range from the classic signal-processing problems of noise and echo cancellation to such far-flung problems as separating song vocals from instrumental tracks and regulating translation length.\n\nA plurality of the papers, however, concentrate on the core technology of **automatic speech recognition** (ASR), or converting an acoustic speech signal into text:\n\n- [**ASR n-best fusion nets**](https://www.amazon.science/publications/asr-n-best-fusion-nets)\n[Xinyue Liu](https://www.amazon.science/author/xinyue-liu), Mingda Li, [Luoxin Chen](https://www.amazon.science/author/luoxin-chen), [Prashan Wanigasekara](https://www.amazon.science/author/prashan-wanigasekara), [Weitong Ruan](https://www.amazon.science/author/wael-hamza), [Haidar Khan](https://www.amazon.science/author/haidar-khan), [Wael Hamza](https://www.amazon.science/author/wael-hamza), [Chengwei Su](https://www.amazon.science/author/chengwei-su)\n\n- [**Bifocal neural ASR: Exploiting keyword spotting for inference optimization**](https://www.amazon.science/publications/bifocal-neural-asr-exploiting-keyword-spotting-for-inference-optimization)\nJon Macoskey, [Grant P. Strimel](https://www.amazon.science/author/grant-p-strimel), [Ariya Rastrow](https://www.amazon.science/author/ariya-rastrow)\n\n- [**Domain-aware neural language models for speech recognition**](https://www.amazon.science/publications/domain-aware-neural-language-models-for-speech-recognition)\n[Linda Liu](https://www.amazon.science/author/linda-liu), [Yile Gu](https://www.amazon.science/author/yile-gu), [Aditya Gourav](https://www.amazon.science/author/aditya-gourav), [Ankur Gandhe](https://www.amazon.science/author/ankur-gandhe), [Shashank Kalmane](https://www.amazon.science/author/shashank-kalmane), [Denis Filimonov](https://www.amazon.science/author/denis-filiminov), [Ariya Rastrow](https://www.amazon.science/author/ariya-rastrow), [Ivan Bulyko](https://www.amazon.science/author/ivan-bulyko)\n\n- [**End-to-end multi-channel transformer for speech recognition**](https://www.amazon.science/publications/end-to-end-multi-channel-transformer-for-speech-recognition)\n[Feng-Ju Chang](https://www.amazon.science/author/feng-ju-chang), [Martin Radfar](https://www.amazon.science/author/martin-radfar), [Athanasios Mouchtaris](https://www.amazon.science/author/athanasios-mouchtaris), [Brian King](https://www.amazon.science/author/brian-king), [Siegfried Kunzmann](https://www.amazon.science/author/siegfried-kunzmann)\n\n- [**Improved robustness to disfluencies in RNN-transducer-based speech recognition**](https://www.amazon.science/publications/improved-robustness-to-disfluencies-in-rnn-transducer-based-speech-recognition)\n[Valentin Mendelev](https://www.amazon.science/author/valentin-mendelev), Tina Raissi, Guglielmo Camporese, [Manuel Giollo](https://www.amazon.science/author/manuel-giollo)\n\n- [**Personalization strategies for end-to-end speech recognition systems**](https://www.amazon.science/publications/personalization-strategies-for-end-to-end-speech-recognition-systems)\n[Aditya Gourav](https://www.amazon.science/author/aditya-gourav), [Linda Liu](https://www.amazon.science/author/linda-liu), [Ankur Gandhe](https://www.amazon.science/author/ankur-gandhe), [Yile Gu](https://www.amazon.science/author/yile-gu), [Guitang Lan](https://www.amazon.science/author/guitang-lan), [Xiangyang Huang](https://www.amazon.science/author/xiangyang-huang), [Shashank Kalmane](https://www.amazon.science/author/shashank-kalmane), [Gautam Tiwari](https://www.amazon.science/author/gautam-tiwari), [Denis Filimonov](https://www.amazon.science/author/denis-filiminov), [Ariya Rastrow](https://www.amazon.science/author/ariya-rastrow), [Andreas Stolcke](https://www.amazon.science/author/andreas-stolcke), [Ivan Bulyko](https://www.amazon.science/author/ivan-bulyko) \n\n- [**reDAT: Accent-invariant representation for end-to-end ASR by domain adversarial training with relabeling**](https://www.amazon.science/publications/redat-accent-invariant-representation-for-end-to-end-asr-by-domain-adversarial-training-with-relabeling)\nHu Hu, [Xuesong Yang](https://www.amazon.science/author/xuesong-yang), [Zeynab Raeesy](https://www.amazon.science/author/zeynab-raeesy), [Jinxi Guo](https://www.amazon.science/author/jinxi-guo), [Gokce Keskin](https://www.amazon.science/author/gokce-keskin), [Harish Arsikere](https://www.amazon.science/author/harish-arsikere), [Ariya Rastrow](https://www.amazon.science/author/ariya-rastrow), [Andreas Stolcke](https://www.amazon.science/author/andreas-stolcke), [Roland Maas](https://www.amazon.science/author/roland-maas) \n\n- [**Sparsification via compressed sensing for automatic speech recognition**](https://www.amazon.science/publications/sparsification-via-compressed-sensing-for-automatic-speech-recognition)\nKai Zhen, [Hieu Duy Nguyen](https://www.amazon.science/author/hieu-duy-nguyen), [Feng-Ju Chang](https://www.amazon.science/author/feng-ju-chang), [Athanasios Mouchtaris](https://www.amazon.science/author/athanasios-mouchtaris), [Ariya Rastrow](https://www.amazon.science/author/ariya-rastrow)\n\n- [**Streaming multi-speaker ASR with RNN-T**](https://www.amazon.science/publications/streaming-multi-speaker-asr-with-rnn-t)\n[Ilya Sklyar](https://www.amazon.science/author/ilya-sklyar), [Anna Piunova](https://www.amazon.science/author/anna-piunova), [Yulan Liu](https://www.amazon.science/author/yulan-liu) \n\n- [**Using synthetic audio to improve the recognition of out-of-vocabulary words in end-to-end ASR systems**](https://www.amazon.science/publications/using-synthetic-audio-to-improve-the-recognition-of-out-of-vocabulary-words-in-end-to-end-asr-systems)\nXianrui Zheng, [Yulan Liu](https://www.amazon.science/author/yulan-liu), [Deniz Gunceler](https://www.amazon.science/author/deniz-gunceler), [Daniel Willett](https://www.amazon.science/author/daniel-willett)\n\n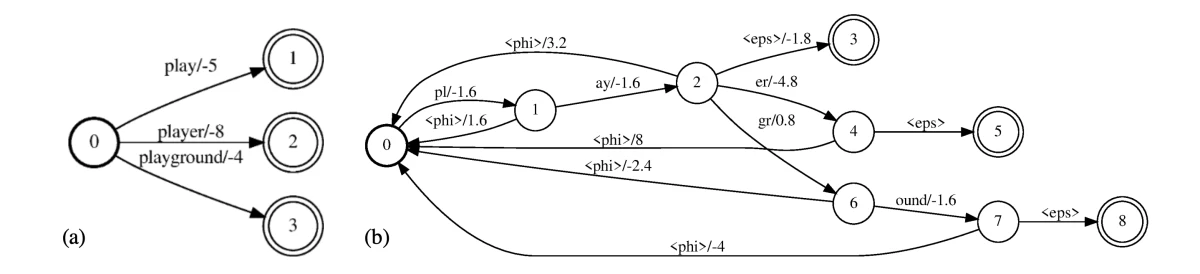\n\nTo enable personalization of end-to-end automatic-speech-recognition systems, Linda Liu, Aditya Gourav and their colleagues use a word-level biasing finite state transducer, or FST (left). A subword-level FST preserves the weights of the word-level FST. For instance, the weight between state 0 and 5 of the subword-level FST (representing the word “player”) is (-1.6) +(- 1.6)+(-4.8) = -8.\n\nFROM [\\"PERSONALIZATION STRATEGIES FOR END-TO-END SPEECH RECOGNITION SYSTEMS\\"](https://www.amazon.science/publications/personalization-strategies-for-end-to-end-speech-recognition-systems)\n\nTwo of the papers address **language (or code) switching**, a more complicated version of ASR in which the speech recognizer must also determine which of several possible languages is being spoken: \n\n- [**Joint ASR and language identification using RNN-T: An efficent approach to dynamic language switching**](https://www.amazon.science/publications/joint-asr-and-language-identification-using-rnn-t-an-efficent-approach-to-dynamic-language-switching)\n[Surabhi Punjabi](https://www.amazon.science/author/surabhi-punjabi), [Harish Arsikere](https://www.amazon.science/author/harish-arsikere), [Zeynab Raeesy](https://www.amazon.science/author/zeynab-raeesy), [Chander Chandak](https://www.amazon.science/author/chander-chandak), [Nikhil Bhave](https://www.amazon.science/author/nikhil-bhave), [Markus Mueller](https://www.amazon.science/author/markus-mueller), [Sergio Murillo](https://www.amazon.science/author/sergio-murillo), [Ariya Rastrow](https://www.amazon.science/author/ariya-rastrow), [Andreas Stolcke](https://www.amazon.science/author/andreas-stolcke), [Jasha Droppo](https://www.amazon.science/author/jasha-droppo), [Sri Garimella](https://www.amazon.science/author/sri-garimella), [Roland Maas](https://www.amazon.science/author/roland-maas), [Mat Hans](https://www.amazon.science/author/mat-hans), [Athanasios Mouchtaris](https://www.amazon.science/author/athanasios-mouchtaris), [Siegfried Kunzmann](https://www.amazon.science/author/siegfried-kunzmann)\n\n- [**Transformer-transducers for code-switched speech recognition**](https://www.amazon.science/publications/transformer-transducers-for-code-switched-speech-recognition)\nSiddharth Dalmia, [Yuzong Liu](https://www.amazon.science/author/yuzong-liu), [Srikanth Ronanki](https://www.amazon.science/author/srikanth-ronanki), [Katrin Kirchhoff ](https://www.amazon.science/author/katrin-kirchhoff)\n\nThe acoustic speech signal contains more information than just the speaker’s words; how the words are said can change their meaning. Such **paralinguistic signals** can be useful for a voice agent trying to determine how to interpret the raw text. Two of Amazon’s ICASSP papers focus on such signals:\n\n- [**Contrastive unsupervised learning for speech emotion recognition**](https://www.amazon.science/publications/contrastive-unsupervised-learning-for-speech-emotion-recognition)\nMao Li, [Bo Yang](https://www.amazon.science/author/bo-yang), [Joshua Levy](https://www.amazon.science/author/joshua-levy), [Andreas Stolcke](https://www.amazon.science/author/andreas-stolcke), [Viktor Rozgic](https://www.amazon.science/author/viktor-rozgic), [Spyros Matsoukas](https://www.amazon.science/author/spyros-matsoukas), [Constantinos Papayiannis](https://www.amazon.science/author/constantinos-papayiannis), [Daniel Bone](https://www.amazon.science/author/daniel-bone), [Chao Wang](https://www.amazon.science/author/chao-wang) \n\n- [**Disentanglement for audiovisual emotion recognition using multitask setup**](https://www.amazon.science/publications/disentanglement-for-audiovisual-emotion-recognition-using-multitask-setup)\nRaghuveer Peri, [Srinivas Parthasarathy](https://www.amazon.science/author/srinivas-parthasarathy), [Charles Bradshaw](https://www.amazon.science/author/charles-bradshaw), [Shiva Sundaram](https://www.amazon.science/blog/null) \n\nSeveral papers address other **extensions of ASR**, such as speaker diarization, or tracking which of several speakers issues each utterance; inverse text normalization, or converting the raw ASR output into a format useful to downstream applications; and acoustic event classification, or recognizing sounds other than human voices:\n\n- [**BW-EDA-EEND: Streaming end-to-end neural speaker diarization for a variable number of speakers**](https://www.amazon.science/publications/bw-eda-eend-streaming-end-to-end-neural-speaker-diarization-for-a-variable-number-of-speakers)\n[Eunjung Han](https://www.amazon.science/author/eunjung-han), [Chul Lee](https://www.amazon.science/author/chul-lee), [Andreas Stolcke](https://www.amazon.science/author/andreas-stolcke)\n\n- [**Neural inverse text normalization**](https://www.amazon.science/publications/neural-inverse-text-normalization)\n[Monica Sunkara](https://www.amazon.science/author/monica-sunkara), [Chaitanya Shivade](https://www.amazon.science/author/chaitanya-shivade), [Sravan Bodapati](https://www.amazon.science/author/sravan-bodapati), [Katrin Kirchhoff](https://www.amazon.science/author/katrin-kirchhoff)\n\n- [**Unsupervised and semi-supervised few-shot acoustic event classification**](https://www.amazon.science/publications/unsupervised-and-semi-supervised-few-shot-acoustic-event-classification)\nHsin-Ping Huang, [Krishna C. Puvvada](https://www.amazon.science/author/krishna-c-puvvada), [Ming Sun](https://www.amazon.science/author/ming-sun), [Chao Wang](https://www.amazon.science/author/chao-wang)\n\n**Speech enhancement**, or removing noise and echo from the speech signal, has been a prominent topic at ICASSP since the conference began in 1976. But more recent work on the topic — including Amazon’s two papers this year — uses deep-learning methods:\n\n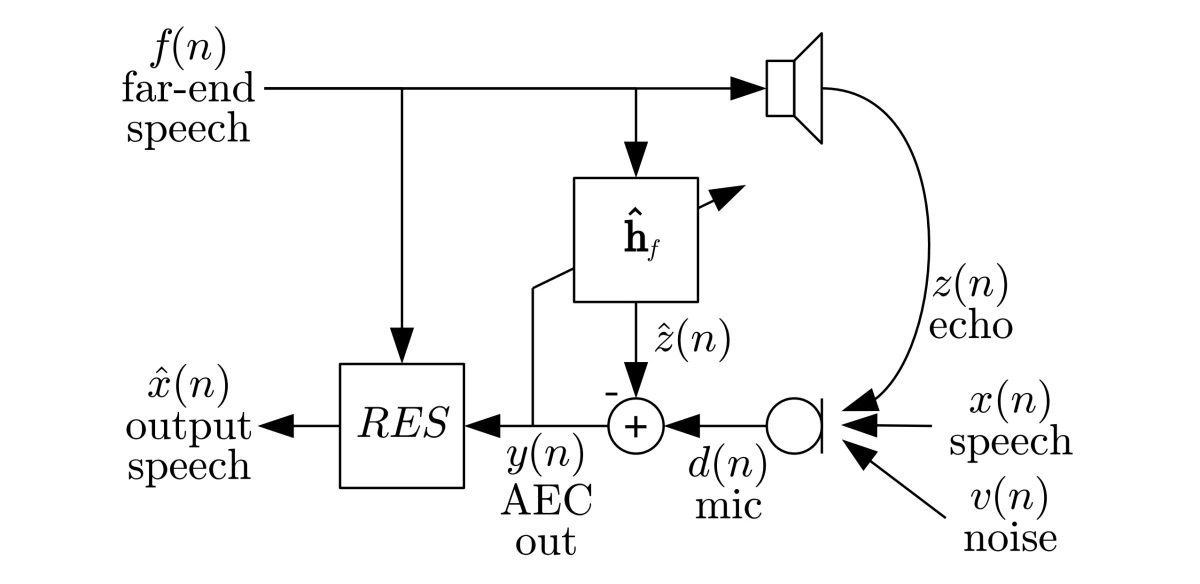\n\nThe structure of a joint echo control and noise suppression system from Amazon. A microphone (mic) captures the output of a loudspeaker, along with noise and echo. The echo is partially cancelled by an adaptive filter (ĥf), which uses the signal to the speaker. The microphone signal then passes to a residual-echo-suppression (RES) algorithm.\n\nFROM [\\"LOW-COMPLEXITY, REAL-TIME JOINT NEURAL ECHO CONTROL AND SPEECH ENHANCEMENT BASED ON PERCEPNET\\"](https://www.amazon.science/publications/low-complexity-real-time-joint-neural-echo-control-and-speech-enhancement-based-on-percepnet)\n\n- [**Enhancing into the codec: Noise robust speech coding with vector-quantized autoencoders**](https://www.amazon.science/publications/enhancing-into-the-codec-noise-robust-speech-coding-with-vector-quantized-autoencoders)\nJonah Casebeer, Vinjai Vale, [Umut Isik](https://www.amazon.science/author/umut-isik), [Jean-Marc Valin](https://www.amazon.science/author/jean-marc-valin), [Ritwik Giri](https://www.amazon.science/author/ritwik-giri), [Arvindh Krishnaswamy](https://www.amazon.science/author/arvindh-krishnaswamy)\n\n- [**Low-complexity, real-time joint neural echo control and speech enhancement based on Percepnet**](https://www.amazon.science/publications/low-complexity-real-time-joint-neural-echo-control-and-speech-enhancement-based-on-percepnet)\n[Jean-Marc Valin](https://www.amazon.science/author/jean-marc-valin), [Srikanth V. Tenneti](https://www.amazon.science/author/srikanth-v-tenneti), [Karim Helwani](https://www.amazon.science/author/karim-helwani), [Umut Isik](https://www.amazon.science/author/umut-isik), [Arvindh Krishnaswamy](https://www.amazon.science/author/arvindh-krishnaswamy)\n\nEvery interaction with Alexa begins with a wake word — usually “Alexa”, but sometimes “computer” or “Echo”. So at ICASSP, Amazon usually presents work on wake word detection — or **keyword spotting**, as it’s more generally known:\n\n- [**Exploring the application of synthetic audio in training keyword spotters**](https://www.amazon.science/publications/exploring-the-application-of-synthetic-audio-in-training-keyword-spotters)\n[Andrew Werchniak](https://www.amazon.science/author/andrew-werchniak), [Roberto Barra-Chicote](https://www.amazon.science/author/roberto-barra-chicote), [Yuriy Mishchenko](https://www.amazon.science/author/yuriy-mishchenko), [Jasha Droppo](https://www.amazon.science/author/jasha-droppo), [Jeff Condal](https://www.amazon.science/author/jeff-condal), [Peng Liu](https://www.amazon.science/author/peng-liu), [Anish Shah ](https://www.amazon.science/author/anish-shah)\n\nIn many spoken-language systems, the next step after ASR **is natural-language understanding** (NLU), or making sense of the text output from the ASR system:\n\n-[ **Introducing deep reinforcement learning to NLU ranking tasks**](https://www.amazon.science/publications/introducing-deep-reinforcement-learning-to-nlu-ranking-tasks)\n[Ge Yu](https://www.amazon.science/author/ge-yu), [Chengwei Su](https://www.amazon.science/author/chengwei-su), [Emre Barut](https://www.amazon.science/author/emre-barut) \n- [**Language model is all you need: Natural language understanding as question answering**](https://www.amazon.science/publications/language-model-is-all-you-need-natural-language-understanding-as-question-answering)\n[Mahdi Namazifar](https://www.amazon.science/author/mahdi-namazifar), [Alexandros Papangelis](https://www.amazon.science/author/alexandros-papangelis), [Gokhan Tur](https://www.amazon.science/author/gokhan-tur), [Dilek Hakkani-Tür ](https://www.amazon.science/author/dilek-hakkani-tur)\n\nIn some contexts, however, it’s possible to perform both ASR and NLU with a single model, in a task known as **spoken-language understanding:**\n\n- [**Do as I mean, not as I say: Sequence loss training for spoken language understanding**](https://www.amazon.science/publications/do-as-i-mean-not-as-i-say-sequence-loss-training-for-spoken-language-understanding)\n[Milind Rao](https://www.amazon.science/author/milind-rao), [Pranav Dheram](https://www.amazon.science/author/pranav-dheram), [Gautam Tiwari](https://www.amazon.science/author/gautam-tiwari), [Anirudh Raju](https://www.amazon.science/author/anirudh-raju), [Jasha Droppo](https://www.amazon.science/author/jasha-droppo), [Ariya Rastrow](https://www.amazon.science/author/ariya-rastrow), [Andreas Stolcke ](https://www.amazon.science/author/andreas-stolcke)\n\n- [**Graph enhanced query rewriting for spoken language understanding system**](https://www.amazon.science/publications/graph-enhanced-query-rewriting-for-spoken-language-understanding-system)\nSiyang Yuan, [Saurabh Gupta](https://www.amazon.science/author/saurabh-gupta), [Xing Fan](https://www.amazon.science/author/xing-fan), [Derek Liu](https://www.amazon.science/author/derek-liu), [Yang Liu](https://www.amazon.science/author/yang-liu), C[henlei (Edward) Guo](https://www.amazon.science/author/chenlei-guo) \n\n- [**Top-down attention in end-to-end spoken language understanding**](https://www.amazon.science/publications/top-down-attention-in-end-to-end-spoken-language-understanding)\nYixin Chen, [Weiyi Lu](https://www.amazon.science/author/weiyi-lu), [Alejandro Mottini ](https://www.amazon.science/author/alejandro-mottini), [Erran Li](https://www.amazon.science/author/erran-li), [Jasha Droppo](https://www.amazon.science/author/jasha-droppo), [Zheng Du](https://www.amazon.science/author/zheng-du), [Belinda Zeng](https://www.amazon.science/author/belinda-zeng)\n\n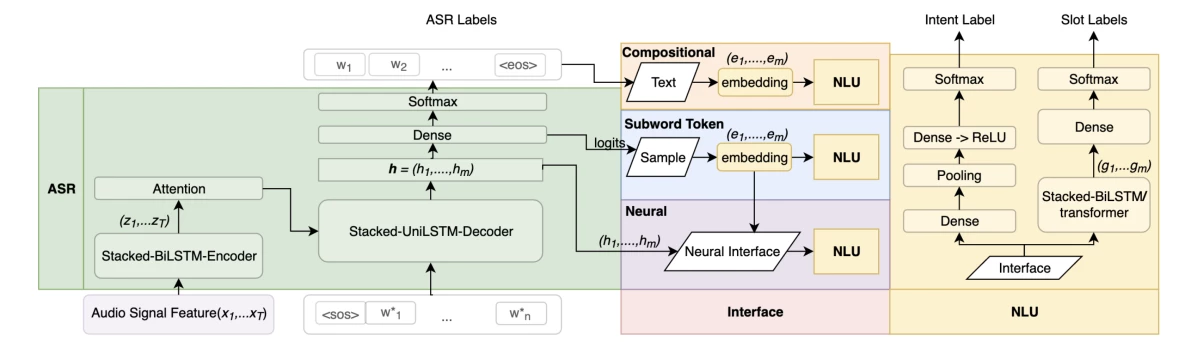\n\nA spoken-language-understanding system combines automatic speech recognition (ASR) and natural-language understanding (NLU) in a single model.\n\nFROM [\\"DO AS I MEAN, NOT AS I SAY: SEQUENCE LOSS TRAINING FOR SPOKEN LANGUAGE UNDERSTANDING\\"](https://www.amazon.science/publications/do-as-i-mean-not-as-i-say-sequence-loss-training-for-spoken-language-understanding)\n\nAn interaction with a voice service, which begins with keyword spotting, ASR, and NLU, often culminates with the agent’s use of synthesized speech to relay a response. The agent’s **text-to-speech** model converts the textual outputs of various NLU and dialogue systems into speech:\n\n- [**CAMP: A two-stage approach to modelling prosody in context**](https://www.amazon.science/publications/camp-a-two-stage-approach-to-modelling-prosody-in-context)\nZack Hodari, [Alexis Moinet](https://www.amazon.science/author/alexis-moinet), [Sri Karlapati](https://www.amazon.science/author/sri-karlapati), [Jaime Lorenzo-Trueba](https://www.amazon.science/author/jaime-lorenzo-trueba), [Thomas Merritt](https://www.amazon.science/author/thomas-merritt), [Arnaud Joly](https://www.amazon.science/author/arnaud-joly), [Ammar Abbas](https://www.amazon.science/author/ammar-abbas), [Penny Karanasou](https://www.amazon.science/author/penny-karanasou), [Thomas Drugman](https://www.amazon.science/author/thomas-drugman)\n\n- [**Low-resource expressive text-to-speech using data augmentation**](https://www.amazon.science/publications/low-resource-expressive-text-to-speech-using-data-augmentation)\n[Goeric Huybrechts](https://www.amazon.science/author/goeric-huybrechts), [Thomas Merritt](https://www.amazon.science/author/thomas-merritt), [Giulia Comini](https://www.amazon.science/author/giulia-comini), [Bartek Perz](https://www.amazon.science/author/bartek-perz), [Raahil Shah](https://www.amazon.science/author/raahil-shah), [Jaime Lorenzo-Trueba](https://www.amazon.science/author/jaime-lorenzo-trueba) \n\n- [**Prosodic representation learning and contextual sampling for neural text-to-speech**](https://www.amazon.science/publications/prosodic-representation-learning-and-contextual-sampling-for-neural-text-to-speech)\n[Sri Karlapati](https://www.amazon.science/author/sri-karlapati), [Ammar Abbas](), Zack Hodari], [Alexis Moinet](https://www.amazon.science/author/alexis-moinet), [Arnaud Joly](https://www.amazon.science/author/arnaud-joly), [Penny Karanasou](https://www.amazon.science/author/penny-karanasou), [Thomas Drugman](https://www.amazon.science/author/thomas-drugman)\n\n- [**Universal neural vocoding with Parallel WaveNet**](https://www.amazon.science/publications/universal-neural-vocoding-with-parallel-wavenet)\n[Yunlong Jiao](https://www.amazon.science/author/yunlong-jiao), [Adam Gabrys](https://www.amazon.science/author/adam-gabrys), Georgi Tinchev, [Bartosz Putrycz](https://www.amazon.science/author/bartosz-putrycz), [Daniel Korzekwa](https://www.amazon.science/author/daniel-korzekwa), [Viacheslav Klimkov](https://www.amazon.science/author/viacheslav-klimkov)\n\nAll of the preceding research topics have implications for voice services like Alexa, but Amazon has a range of other products and services that rely on audio-signal processing. Three of Amazon’s papers at this year’s ICASSP relate to **audio-video synchronization**: two deal with dubbing audio in one language onto video shot in another, and one describes how to detect synchronization errors in video — as when, for example, the sound of a tennis ball being struck and the shot of the racquet hitting the ball are misaligned:\n\n- [**Detection of audio-video synchronization errors via event detection**](https://www.amazon.science/publications/detection-of-audio-video-synchronization-errors-via-event-detection)\nJoshua P. Ebenezer, [Yongjun Wu](https://www.amazon.science/author/yongjun-wu), [Hai Wei](https://www.amazon.science/author/hai-wei), [Sriram Sethuraman](https://www.amazon.science/author/sriram-sethuraman), [Zongyi Liu ](https://www.amazon.science/author/zongyi-liu)\n\n- [**Improvements to prosodic alignment for automatic dubbing**](https://www.amazon.science/publications/improvements-to-prosodic-alignment-for-automatic-dubbing)\n[Yogesh Virkar](https://www.amazon.science/author/yogesh-virkar), [Marcello Federico](https://www.amazon.science/author/marcello-federico), [Robert Enyedi](https://www.amazon.science/author/robert-enyedi), [Roberto Barra-Chicote](https://www.amazon.science/author/roberto-barra-chicote)\n\n- [**Machine translation verbosity control for automatic dubbing**](https://www.amazon.science/publications/machine-translation-verbosity-control-for-automatic-dubbing)\n[Surafel Melaku Lakew](https://www.amazon.science/https:/www.amazon.science/author/surafel-melaku-lakew), [Marcello Federico](https://www.amazon.science/author/marcello-federico), [Yue Wang](https://www.amazon.science/author/yue-wang), [Cuong Hoang](https://www.amazon.science/author/cuong-hoang), [Yogesh Virkar](https://www.amazon.science/author/yogesh-virkar), [Roberto Barra-Chicote](https://www.amazon.science/author/roberto-barra-chicote), [Robert Enyedi ](https://www.amazon.science/author/robert-enyedi)\n\nAmazon’s Text-to-Speech team has an ICASSP paper on the unusual topic of **computer-assisted pronunciation training**, a feature of some language learning applications. The researchers’ method would enable language learning apps to accept a wider range of word pronunciations, to score pronunciations more accurately, and to provide more reliable feedback:\n\n- [**Mispronunciation detection in non-native (L2) English with uncertainty modeling**](https://www.amazon.science/publications/mispronunciation-detection-in-non-native-l2-english-with-uncertainty-modeling)\n[Daniel Korzekwa](https://www.amazon.science/author/daniel-korzekwa), [Jaime Lorenzo-Trueba](https://www.amazon.science/author/jaime-lorenzo-trueba), Szymon Zaporowski, [Shira Calamaro](https://www.amazon.science/author/shira-calamaro), [Thomas Drugman](https://www.amazon.science/author/thomas-drugman), Bozena Kostek \n\n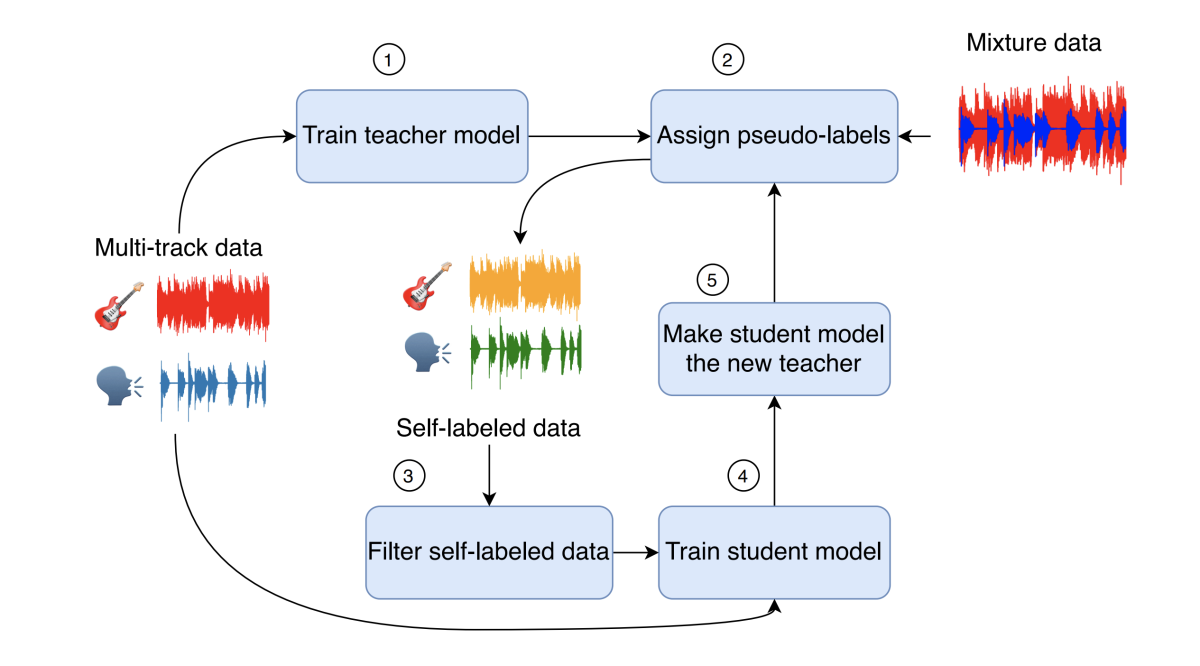\n\nThe architecture of a new Amazon model for separating a recording's vocal tracks and instrumental tracks.\n\nFROM [\\"SEMI-SUPERVISED SINGING VOICE SEPARATION WITH NOISE SELF-TRAINING\\"](https://www.amazon.science/publications/semi-supervised-singing-voice-separation-with-noise-self-training)\n\nAnother paper investigates the topic of **singing voice separation**, or separating vocal tracks from instrumental tracks in song recordings:\n\n- [**Semi-supervised singing voice separation with noise self-training**](https://www.amazon.science/publications/semi-supervised-singing-voice-separation-with-noise-self-training)\nZhepei Wang, [Ritwik Giri](https://www.amazon.science/author/ritwik-giri), [Umut Isik](https://www.amazon.science/author/umut-isik), [Jean-Marc Valin](https://www.amazon.science/author/jean-marc-valin), [Arvindh Krishnaswamy ](https://www.amazon.science/author/arvindh-krishnaswamy)\n\nFinally, two of Amazon’s ICASSP papers, although they do evaluate applications in speech recognition and audio classification, present general **machine learning methodologies** that could apply to a range of problems. One paper investigates federated learning, a distributed-learning technique in which multiple servers, each with a different, local store of training data, collectively build a machine learning model without exchanging data. The other presents a new loss function for training classification models on synthetic data created by transforming real data — for instance, training a sound classification model with samples that have noise added to them artificially.\n\n- [**Cross-silo federated training in the cloud with diversity scaling and semi-supervised learning**](https://www.amazon.science/publications/cross-silo-federated-training-in-the-cloud-with-diversity-scaling-and-semi-supervised-learning)\n[Kishore Nandury](https://www.amazon.science/author/kishore-nandury), [Anand Mohan](https://www.amazon.science/author/anand-mohan), [Frederick Weber](https://www.amazon.science/author/frederick-weber) \n- [**Enhancing audio augmentation methods with consistency learning**](https://www.amazon.science/publications/enhancing-audio-augmentation-methods-with-consistency-learning)\nTurab Iqbal, [Karim Helwani](https://www.amazon.science/author/karim-helwani), [Arvindh Krishnaswamy](https://www.amazon.science/author/arvindh-krishnaswamy), Wenwu Wang\n\nAlso at ICASSP, on June 8, seven Amazon scientists will be participating in a half-hour live Q&A. Conference registrants may [submit questions to the panelists](https://amazon.qualtrics.com/jfe/form/SV_0uf9M3PTwoL0Rfw) online.\n\nABOUT THE AUTHOR\n\n#### [Larry Hardesty](https://www.amazon.science/author/larry-hardesty)\n\nLarry Hardesty is the editor of the Amazon Science blog. Previously, he was a senior editor at MIT Technology Review and the computer science writer at the MIT News Office.\n\n\n\n\n","render":"<p>The International Conference on Acoustics, Speech, and Signal Processing (<a href=\\"https://www.amazon.science/conferences-and-events/icassp-2021\\" target=\\"_blank\\">ICASSP</a>) starts next week, and as Alexa principal research scientist Ariya Rastrow <a href=\\"https://www.amazon.science/blog/icassp-what-signal-processing-has-come-to-mean\\" target=\\"_blank\\">explained last year</a>, it casts a wide net. The topics of the 36 Amazon research papers at this year’s ICASSP range from the classic signal-processing problems of noise and echo cancellation to such far-flung problems as separating song vocals from instrumental tracks and regulating translation length.</p>\\n<p>A plurality of the papers, however, concentrate on the core technology of <strong>automatic speech recognition</strong> (ASR), or converting an acoustic speech signal into text:</p>\\n<ul>\\n<li>\\n<p><a href=\\"https://www.amazon.science/publications/asr-n-best-fusion-nets\\" target=\\"_blank\\"><strong>ASR n-best fusion nets</strong></a><br />\\n<a href=\\"https://www.amazon.science/author/xinyue-liu\\" target=\\"_blank\\">Xinyue Liu</a>, Mingda Li, <a href=\\"https://www.amazon.science/author/luoxin-chen\\" target=\\"_blank\\">Luoxin Chen</a>, <a href=\\"https://www.amazon.science/author/prashan-wanigasekara\\" target=\\"_blank\\">Prashan Wanigasekara</a>, <a href=\\"https://www.amazon.science/author/wael-hamza\\" target=\\"_blank\\">Weitong Ruan</a>, <a href=\\"https://www.amazon.science/author/haidar-khan\\" target=\\"_blank\\">Haidar Khan</a>, <a href=\\"https://www.amazon.science/author/wael-hamza\\" target=\\"_blank\\">Wael Hamza</a>, <a href=\\"https://www.amazon.science/author/chengwei-su\\" target=\\"_blank\\">Chengwei Su</a></p>\\n</li>\n<li>\\n<p><a href=\\"https://www.amazon.science/publications/bifocal-neural-asr-exploiting-keyword-spotting-for-inference-optimization\\" target=\\"_blank\\"><strong>Bifocal neural ASR: Exploiting keyword spotting for inference optimization</strong></a><br />\\nJon Macoskey, <a href=\\"https://www.amazon.science/author/grant-p-strimel\\" target=\\"_blank\\">Grant P. Strimel</a>, <a href=\\"https://www.amazon.science/author/ariya-rastrow\\" target=\\"_blank\\">Ariya Rastrow</a></p>\\n</li>\n<li>\\n<p><a href=\\"https://www.amazon.science/publications/domain-aware-neural-language-models-for-speech-recognition\\" target=\\"_blank\\"><strong>Domain-aware neural language models for speech recognition</strong></a><br />\\n<a href=\\"https://www.amazon.science/author/linda-liu\\" target=\\"_blank\\">Linda Liu</a>, <a href=\\"https://www.amazon.science/author/yile-gu\\" target=\\"_blank\\">Yile Gu</a>, <a href=\\"https://www.amazon.science/author/aditya-gourav\\" target=\\"_blank\\">Aditya Gourav</a>, <a href=\\"https://www.amazon.science/author/ankur-gandhe\\" target=\\"_blank\\">Ankur Gandhe</a>, <a href=\\"https://www.amazon.science/author/shashank-kalmane\\" target=\\"_blank\\">Shashank Kalmane</a>, <a href=\\"https://www.amazon.science/author/denis-filiminov\\" target=\\"_blank\\">Denis Filimonov</a>, <a href=\\"https://www.amazon.science/author/ariya-rastrow\\" target=\\"_blank\\">Ariya Rastrow</a>, <a href=\\"https://www.amazon.science/author/ivan-bulyko\\" target=\\"_blank\\">Ivan Bulyko</a></p>\\n</li>\n<li>\\n<p><a href=\\"https://www.amazon.science/publications/end-to-end-multi-channel-transformer-for-speech-recognition\\" target=\\"_blank\\"><strong>End-to-end multi-channel transformer for speech recognition</strong></a><br />\\n<a href=\\"https://www.amazon.science/author/feng-ju-chang\\" target=\\"_blank\\">Feng-Ju Chang</a>, <a href=\\"https://www.amazon.science/author/martin-radfar\\" target=\\"_blank\\">Martin Radfar</a>, <a href=\\"https://www.amazon.science/author/athanasios-mouchtaris\\" target=\\"_blank\\">Athanasios Mouchtaris</a>, <a href=\\"https://www.amazon.science/author/brian-king\\" target=\\"_blank\\">Brian King</a>, <a href=\\"https://www.amazon.science/author/siegfried-kunzmann\\" target=\\"_blank\\">Siegfried Kunzmann</a></p>\\n</li>\n<li>\\n<p><a href=\\"https://www.amazon.science/publications/improved-robustness-to-disfluencies-in-rnn-transducer-based-speech-recognition\\" target=\\"_blank\\"><strong>Improved robustness to disfluencies in RNN-transducer-based speech recognition</strong></a><br />\\n<a href=\\"https://www.amazon.science/author/valentin-mendelev\\" target=\\"_blank\\">Valentin Mendelev</a>, Tina Raissi, Guglielmo Camporese, <a href=\\"https://www.amazon.science/author/manuel-giollo\\" target=\\"_blank\\">Manuel Giollo</a></p>\\n</li>\n<li>\\n<p><a href=\\"https://www.amazon.science/publications/personalization-strategies-for-end-to-end-speech-recognition-systems\\" target=\\"_blank\\"><strong>Personalization strategies for end-to-end speech recognition systems</strong></a><br />\\n<a href=\\"https://www.amazon.science/author/aditya-gourav\\" target=\\"_blank\\">Aditya Gourav</a>, <a href=\\"https://www.amazon.science/author/linda-liu\\" target=\\"_blank\\">Linda Liu</a>, <a href=\\"https://www.amazon.science/author/ankur-gandhe\\" target=\\"_blank\\">Ankur Gandhe</a>, <a href=\\"https://www.amazon.science/author/yile-gu\\" target=\\"_blank\\">Yile Gu</a>, <a href=\\"https://www.amazon.science/author/guitang-lan\\" target=\\"_blank\\">Guitang Lan</a>, <a href=\\"https://www.amazon.science/author/xiangyang-huang\\" target=\\"_blank\\">Xiangyang Huang</a>, <a href=\\"https://www.amazon.science/author/shashank-kalmane\\" target=\\"_blank\\">Shashank Kalmane</a>, <a href=\\"https://www.amazon.science/author/gautam-tiwari\\" target=\\"_blank\\">Gautam Tiwari</a>, <a href=\\"https://www.amazon.science/author/denis-filiminov\\" target=\\"_blank\\">Denis Filimonov</a>, <a href=\\"https://www.amazon.science/author/ariya-rastrow\\" target=\\"_blank\\">Ariya Rastrow</a>, <a href=\\"https://www.amazon.science/author/andreas-stolcke\\" target=\\"_blank\\">Andreas Stolcke</a>, <a href=\\"https://www.amazon.science/author/ivan-bulyko\\" target=\\"_blank\\">Ivan Bulyko</a></p>\\n</li>\n<li>\\n<p><a href=\\"https://www.amazon.science/publications/redat-accent-invariant-representation-for-end-to-end-asr-by-domain-adversarial-training-with-relabeling\\" target=\\"_blank\\"><strong>reDAT: Accent-invariant representation for end-to-end ASR by domain adversarial training with relabeling</strong></a><br />\\nHu Hu, <a href=\\"https://www.amazon.science/author/xuesong-yang\\" target=\\"_blank\\">Xuesong Yang</a>, <a href=\\"https://www.amazon.science/author/zeynab-raeesy\\" target=\\"_blank\\">Zeynab Raeesy</a>, <a href=\\"https://www.amazon.science/author/jinxi-guo\\" target=\\"_blank\\">Jinxi Guo</a>, <a href=\\"https://www.amazon.science/author/gokce-keskin\\" target=\\"_blank\\">Gokce Keskin</a>, <a href=\\"https://www.amazon.science/author/harish-arsikere\\" target=\\"_blank\\">Harish Arsikere</a>, <a href=\\"https://www.amazon.science/author/ariya-rastrow\\" target=\\"_blank\\">Ariya Rastrow</a>, <a href=\\"https://www.amazon.science/author/andreas-stolcke\\" target=\\"_blank\\">Andreas Stolcke</a>, <a href=\\"https://www.amazon.science/author/roland-maas\\" target=\\"_blank\\">Roland Maas</a></p>\\n</li>\n<li>\\n<p><a href=\\"https://www.amazon.science/publications/sparsification-via-compressed-sensing-for-automatic-speech-recognition\\" target=\\"_blank\\"><strong>Sparsification via compressed sensing for automatic speech recognition</strong></a><br />\\nKai Zhen, <a href=\\"https://www.amazon.science/author/hieu-duy-nguyen\\" target=\\"_blank\\">Hieu Duy Nguyen</a>, <a href=\\"https://www.amazon.science/author/feng-ju-chang\\" target=\\"_blank\\">Feng-Ju Chang</a>, <a href=\\"https://www.amazon.science/author/athanasios-mouchtaris\\" target=\\"_blank\\">Athanasios Mouchtaris</a>, <a href=\\"https://www.amazon.science/author/ariya-rastrow\\" target=\\"_blank\\">Ariya Rastrow</a></p>\\n</li>\n<li>\\n<p><a href=\\"https://www.amazon.science/publications/streaming-multi-speaker-asr-with-rnn-t\\" target=\\"_blank\\"><strong>Streaming multi-speaker ASR with RNN-T</strong></a><br />\\n<a href=\\"https://www.amazon.science/author/ilya-sklyar\\" target=\\"_blank\\">Ilya Sklyar</a>, <a href=\\"https://www.amazon.science/author/anna-piunova\\" target=\\"_blank\\">Anna Piunova</a>, <a href=\\"https://www.amazon.science/author/yulan-liu\\" target=\\"_blank\\">Yulan Liu</a></p>\\n</li>\n<li>\\n<p><a href=\\"https://www.amazon.science/publications/using-synthetic-audio-to-improve-the-recognition-of-out-of-vocabulary-words-in-end-to-end-asr-systems\\" target=\\"_blank\\"><strong>Using synthetic audio to improve the recognition of out-of-vocabulary words in end-to-end ASR systems</strong></a><br />\\nXianrui Zheng, <a href=\\"https://www.amazon.science/author/yulan-liu\\" target=\\"_blank\\">Yulan Liu</a>, <a href=\\"https://www.amazon.science/author/deniz-gunceler\\" target=\\"_blank\\">Deniz Gunceler</a>, <a href=\\"https://www.amazon.science/author/daniel-willett\\" target=\\"_blank\\">Daniel Willett</a></p>\\n</li>\n</ul>\\n<p><img src=\\"https://dev-media.amazoncloud.cn/15c4b6efdfa140a28a0c4a3a8e0006e2_image.png\\" alt=\\"image.png\\" /></p>\n<p>To enable personalization of end-to-end automatic-speech-recognition systems, Linda Liu, Aditya Gourav and their colleagues use a word-level biasing finite state transducer, or FST (left). A subword-level FST preserves the weights of the word-level FST. For instance, the weight between state 0 and 5 of the subword-level FST (representing the word “player”) is (-1.6) +(- 1.6)+(-4.8) = -8.</p>\n<p>FROM <a href=\\"https://www.amazon.science/publications/personalization-strategies-for-end-to-end-speech-recognition-systems\\" target=\\"_blank\\">“PERSONALIZATION STRATEGIES FOR END-TO-END SPEECH RECOGNITION SYSTEMS”</a></p>\\n<p>Two of the papers address <strong>language (or code) switching</strong>, a more complicated version of ASR in which the speech recognizer must also determine which of several possible languages is being spoken:</p>\\n<ul>\\n<li>\\n<p><a href=\\"https://www.amazon.science/publications/joint-asr-and-language-identification-using-rnn-t-an-efficent-approach-to-dynamic-language-switching\\" target=\\"_blank\\"><strong>Joint ASR and language identification using RNN-T: An efficent approach to dynamic language switching</strong></a><br />\\n<a href=\\"https://www.amazon.science/author/surabhi-punjabi\\" target=\\"_blank\\">Surabhi Punjabi</a>, <a href=\\"https://www.amazon.science/author/harish-arsikere\\" target=\\"_blank\\">Harish Arsikere</a>, <a href=\\"https://www.amazon.science/author/zeynab-raeesy\\" target=\\"_blank\\">Zeynab Raeesy</a>, <a href=\\"https://www.amazon.science/author/chander-chandak\\" target=\\"_blank\\">Chander Chandak</a>, <a href=\\"https://www.amazon.science/author/nikhil-bhave\\" target=\\"_blank\\">Nikhil Bhave</a>, <a href=\\"https://www.amazon.science/author/markus-mueller\\" target=\\"_blank\\">Markus Mueller</a>, <a href=\\"https://www.amazon.science/author/sergio-murillo\\" target=\\"_blank\\">Sergio Murillo</a>, <a href=\\"https://www.amazon.science/author/ariya-rastrow\\" target=\\"_blank\\">Ariya Rastrow</a>, <a href=\\"https://www.amazon.science/author/andreas-stolcke\\" target=\\"_blank\\">Andreas Stolcke</a>, <a href=\\"https://www.amazon.science/author/jasha-droppo\\" target=\\"_blank\\">Jasha Droppo</a>, <a href=\\"https://www.amazon.science/author/sri-garimella\\" target=\\"_blank\\">Sri Garimella</a>, <a href=\\"https://www.amazon.science/author/roland-maas\\" target=\\"_blank\\">Roland Maas</a>, <a href=\\"https://www.amazon.science/author/mat-hans\\" target=\\"_blank\\">Mat Hans</a>, <a href=\\"https://www.amazon.science/author/athanasios-mouchtaris\\" target=\\"_blank\\">Athanasios Mouchtaris</a>, <a href=\\"https://www.amazon.science/author/siegfried-kunzmann\\" target=\\"_blank\\">Siegfried Kunzmann</a></p>\\n</li>\n<li>\\n<p><a href=\\"https://www.amazon.science/publications/transformer-transducers-for-code-switched-speech-recognition\\" target=\\"_blank\\"><strong>Transformer-transducers for code-switched speech recognition</strong></a><br />\\nSiddharth Dalmia, <a href=\\"https://www.amazon.science/author/yuzong-liu\\" target=\\"_blank\\">Yuzong Liu</a>, <a href=\\"https://www.amazon.science/author/srikanth-ronanki\\" target=\\"_blank\\">Srikanth Ronanki</a>, <a href=\\"https://www.amazon.science/author/katrin-kirchhoff\\" target=\\"_blank\\">Katrin Kirchhoff </a></p>\\n</li>\n</ul>\\n<p>The acoustic speech signal contains more information than just the speaker’s words; how the words are said can change their meaning. Such <strong>paralinguistic signals</strong> can be useful for a voice agent trying to determine how to interpret the raw text. Two of Amazon’s ICASSP papers focus on such signals:</p>\\n<ul>\\n<li>\\n<p><a href=\\"https://www.amazon.science/publications/contrastive-unsupervised-learning-for-speech-emotion-recognition\\" target=\\"_blank\\"><strong>Contrastive unsupervised learning for speech emotion recognition</strong></a><br />\\nMao Li, <a href=\\"https://www.amazon.science/author/bo-yang\\" target=\\"_blank\\">Bo Yang</a>, <a href=\\"https://www.amazon.science/author/joshua-levy\\" target=\\"_blank\\">Joshua Levy</a>, <a href=\\"https://www.amazon.science/author/andreas-stolcke\\" target=\\"_blank\\">Andreas Stolcke</a>, <a href=\\"https://www.amazon.science/author/viktor-rozgic\\" target=\\"_blank\\">Viktor Rozgic</a>, <a href=\\"https://www.amazon.science/author/spyros-matsoukas\\" target=\\"_blank\\">Spyros Matsoukas</a>, <a href=\\"https://www.amazon.science/author/constantinos-papayiannis\\" target=\\"_blank\\">Constantinos Papayiannis</a>, <a href=\\"https://www.amazon.science/author/daniel-bone\\" target=\\"_blank\\">Daniel Bone</a>, <a href=\\"https://www.amazon.science/author/chao-wang\\" target=\\"_blank\\">Chao Wang</a></p>\\n</li>\n<li>\\n<p><a href=\\"https://www.amazon.science/publications/disentanglement-for-audiovisual-emotion-recognition-using-multitask-setup\\" target=\\"_blank\\"><strong>Disentanglement for audiovisual emotion recognition using multitask setup</strong></a><br />\\nRaghuveer Peri, <a href=\\"https://www.amazon.science/author/srinivas-parthasarathy\\" target=\\"_blank\\">Srinivas Parthasarathy</a>, <a href=\\"https://www.amazon.science/author/charles-bradshaw\\" target=\\"_blank\\">Charles Bradshaw</a>, <a href=\\"https://www.amazon.science/blog/null\\" target=\\"_blank\\">Shiva Sundaram</a></p>\\n</li>\n</ul>\\n<p>Several papers address other <strong>extensions of ASR</strong>, such as speaker diarization, or tracking which of several speakers issues each utterance; inverse text normalization, or converting the raw ASR output into a format useful to downstream applications; and acoustic event classification, or recognizing sounds other than human voices:</p>\\n<ul>\\n<li>\\n<p><a href=\\"https://www.amazon.science/publications/bw-eda-eend-streaming-end-to-end-neural-speaker-diarization-for-a-variable-number-of-speakers\\" target=\\"_blank\\"><strong>BW-EDA-EEND: Streaming end-to-end neural speaker diarization for a variable number of speakers</strong></a><br />\\n<a href=\\"https://www.amazon.science/author/eunjung-han\\" target=\\"_blank\\">Eunjung Han</a>, <a href=\\"https://www.amazon.science/author/chul-lee\\" target=\\"_blank\\">Chul Lee</a>, <a href=\\"https://www.amazon.science/author/andreas-stolcke\\" target=\\"_blank\\">Andreas Stolcke</a></p>\\n</li>\n<li>\\n<p><a href=\\"https://www.amazon.science/publications/neural-inverse-text-normalization\\" target=\\"_blank\\"><strong>Neural inverse text normalization</strong></a><br />\\n<a href=\\"https://www.amazon.science/author/monica-sunkara\\" target=\\"_blank\\">Monica Sunkara</a>, <a href=\\"https://www.amazon.science/author/chaitanya-shivade\\" target=\\"_blank\\">Chaitanya Shivade</a>, <a href=\\"https://www.amazon.science/author/sravan-bodapati\\" target=\\"_blank\\">Sravan Bodapati</a>, <a href=\\"https://www.amazon.science/author/katrin-kirchhoff\\" target=\\"_blank\\">Katrin Kirchhoff</a></p>\\n</li>\n<li>\\n<p><a href=\\"https://www.amazon.science/publications/unsupervised-and-semi-supervised-few-shot-acoustic-event-classification\\" target=\\"_blank\\"><strong>Unsupervised and semi-supervised few-shot acoustic event classification</strong></a><br />\\nHsin-Ping Huang, <a href=\\"https://www.amazon.science/author/krishna-c-puvvada\\" target=\\"_blank\\">Krishna C. Puvvada</a>, <a href=\\"https://www.amazon.science/author/ming-sun\\" target=\\"_blank\\">Ming Sun</a>, <a href=\\"https://www.amazon.science/author/chao-wang\\" target=\\"_blank\\">Chao Wang</a></p>\\n</li>\n</ul>\\n<p><strong>Speech enhancement</strong>, or removing noise and echo from the speech signal, has been a prominent topic at ICASSP since the conference began in 1976. But more recent work on the topic — including Amazon’s two papers this year — uses deep-learning methods:</p>\\n<p><img src=\\"https://dev-media.amazoncloud.cn/8a67b6ead95941bf9b209f4c3804b6ce_image.png\\" alt=\\"image.png\\" /></p>\n<p>The structure of a joint echo control and noise suppression system from Amazon. A microphone (mic) captures the output of a loudspeaker, along with noise and echo. The echo is partially cancelled by an adaptive filter (ĥf), which uses the signal to the speaker. The microphone signal then passes to a residual-echo-suppression (RES) algorithm.</p>\n<p>FROM <a href=\\"https://www.amazon.science/publications/low-complexity-real-time-joint-neural-echo-control-and-speech-enhancement-based-on-percepnet\\" target=\\"_blank\\">“LOW-COMPLEXITY, REAL-TIME JOINT NEURAL ECHO CONTROL AND SPEECH ENHANCEMENT BASED ON PERCEPNET”</a></p>\\n<ul>\\n<li>\\n<p><a href=\\"https://www.amazon.science/publications/enhancing-into-the-codec-noise-robust-speech-coding-with-vector-quantized-autoencoders\\" target=\\"_blank\\"><strong>Enhancing into the codec: Noise robust speech coding with vector-quantized autoencoders</strong></a><br />\\nJonah Casebeer, Vinjai Vale, <a href=\\"https://www.amazon.science/author/umut-isik\\" target=\\"_blank\\">Umut Isik</a>, <a href=\\"https://www.amazon.science/author/jean-marc-valin\\" target=\\"_blank\\">Jean-Marc Valin</a>, <a href=\\"https://www.amazon.science/author/ritwik-giri\\" target=\\"_blank\\">Ritwik Giri</a>, <a href=\\"https://www.amazon.science/author/arvindh-krishnaswamy\\" target=\\"_blank\\">Arvindh Krishnaswamy</a></p>\\n</li>\n<li>\\n<p><a href=\\"https://www.amazon.science/publications/low-complexity-real-time-joint-neural-echo-control-and-speech-enhancement-based-on-percepnet\\" target=\\"_blank\\"><strong>Low-complexity, real-time joint neural echo control and speech enhancement based on Percepnet</strong></a><br />\\n<a href=\\"https://www.amazon.science/author/jean-marc-valin\\" target=\\"_blank\\">Jean-Marc Valin</a>, <a href=\\"https://www.amazon.science/author/srikanth-v-tenneti\\" target=\\"_blank\\">Srikanth V. Tenneti</a>, <a href=\\"https://www.amazon.science/author/karim-helwani\\" target=\\"_blank\\">Karim Helwani</a>, <a href=\\"https://www.amazon.science/author/umut-isik\\" target=\\"_blank\\">Umut Isik</a>, <a href=\\"https://www.amazon.science/author/arvindh-krishnaswamy\\" target=\\"_blank\\">Arvindh Krishnaswamy</a></p>\\n</li>\n</ul>\\n<p>Every interaction with Alexa begins with a wake word — usually “Alexa”, but sometimes “computer” or “Echo”. So at ICASSP, Amazon usually presents work on wake word detection — or <strong>keyword spotting</strong>, as it’s more generally known:</p>\\n<ul>\\n<li><a href=\\"https://www.amazon.science/publications/exploring-the-application-of-synthetic-audio-in-training-keyword-spotters\\" target=\\"_blank\\"><strong>Exploring the application of synthetic audio in training keyword spotters</strong></a><br />\\n<a href=\\"https://www.amazon.science/author/andrew-werchniak\\" target=\\"_blank\\">Andrew Werchniak</a>, <a href=\\"https://www.amazon.science/author/roberto-barra-chicote\\" target=\\"_blank\\">Roberto Barra-Chicote</a>, <a href=\\"https://www.amazon.science/author/yuriy-mishchenko\\" target=\\"_blank\\">Yuriy Mishchenko</a>, <a href=\\"https://www.amazon.science/author/jasha-droppo\\" target=\\"_blank\\">Jasha Droppo</a>, <a href=\\"https://www.amazon.science/author/jeff-condal\\" target=\\"_blank\\">Jeff Condal</a>, <a href=\\"https://www.amazon.science/author/peng-liu\\" target=\\"_blank\\">Peng Liu</a>, <a href=\\"https://www.amazon.science/author/anish-shah\\" target=\\"_blank\\">Anish Shah </a></li>\\n</ul>\n<p>In many spoken-language systems, the next step after ASR <strong>is natural-language understanding</strong> (NLU), or making sense of the text output from the ASR system:</p>\\n<p>-<a href=\\"https://www.amazon.science/publications/introducing-deep-reinforcement-learning-to-nlu-ranking-tasks\\" target=\\"_blank\\"> <strong>Introducing deep reinforcement learning to NLU ranking tasks</strong></a><br />\\n<a href=\\"https://www.amazon.science/author/ge-yu\\" target=\\"_blank\\">Ge Yu</a>, <a href=\\"https://www.amazon.science/author/chengwei-su\\" target=\\"_blank\\">Chengwei Su</a>, <a href=\\"https://www.amazon.science/author/emre-barut\\" target=\\"_blank\\">Emre Barut</a></p>\\n<ul>\\n<li><a href=\\"https://www.amazon.science/publications/language-model-is-all-you-need-natural-language-understanding-as-question-answering\\" target=\\"_blank\\"><strong>Language model is all you need: Natural language understanding as question answering</strong></a><br />\\n<a href=\\"https://www.amazon.science/author/mahdi-namazifar\\" target=\\"_blank\\">Mahdi Namazifar</a>, <a href=\\"https://www.amazon.science/author/alexandros-papangelis\\" target=\\"_blank\\">Alexandros Papangelis</a>, <a href=\\"https://www.amazon.science/author/gokhan-tur\\" target=\\"_blank\\">Gokhan Tur</a>, <a href=\\"https://www.amazon.science/author/dilek-hakkani-tur\\" target=\\"_blank\\">Dilek Hakkani-Tür </a></li>\\n</ul>\n<p>In some contexts, however, it’s possible to perform both ASR and NLU with a single model, in a task known as <strong>spoken-language understanding:</strong></p>\\n<ul>\\n<li>\\n<p><a href=\\"https://www.amazon.science/publications/do-as-i-mean-not-as-i-say-sequence-loss-training-for-spoken-language-understanding\\" target=\\"_blank\\"><strong>Do as I mean, not as I say: Sequence loss training for spoken language understanding</strong></a><br />\\n<a href=\\"https://www.amazon.science/author/milind-rao\\" target=\\"_blank\\">Milind Rao</a>, <a href=\\"https://www.amazon.science/author/pranav-dheram\\" target=\\"_blank\\">Pranav Dheram</a>, <a href=\\"https://www.amazon.science/author/gautam-tiwari\\" target=\\"_blank\\">Gautam Tiwari</a>, <a href=\\"https://www.amazon.science/author/anirudh-raju\\" target=\\"_blank\\">Anirudh Raju</a>, <a href=\\"https://www.amazon.science/author/jasha-droppo\\" target=\\"_blank\\">Jasha Droppo</a>, <a href=\\"https://www.amazon.science/author/ariya-rastrow\\" target=\\"_blank\\">Ariya Rastrow</a>, <a href=\\"https://www.amazon.science/author/andreas-stolcke\\" target=\\"_blank\\">Andreas Stolcke </a></p>\\n</li>\n<li>\\n<p><a href=\\"https://www.amazon.science/publications/graph-enhanced-query-rewriting-for-spoken-language-understanding-system\\" target=\\"_blank\\"><strong>Graph enhanced query rewriting for spoken language understanding system</strong></a><br />\\nSiyang Yuan, <a href=\\"https://www.amazon.science/author/saurabh-gupta\\" target=\\"_blank\\">Saurabh Gupta</a>, <a href=\\"https://www.amazon.science/author/xing-fan\\" target=\\"_blank\\">Xing Fan</a>, <a href=\\"https://www.amazon.science/author/derek-liu\\" target=\\"_blank\\">Derek Liu</a>, <a href=\\"https://www.amazon.science/author/yang-liu\\" target=\\"_blank\\">Yang Liu</a>, C<a href=\\"https://www.amazon.science/author/chenlei-guo\\" target=\\"_blank\\">henlei (Edward) Guo</a></p>\\n</li>\n<li>\\n<p><a href=\\"https://www.amazon.science/publications/top-down-attention-in-end-to-end-spoken-language-understanding\\" target=\\"_blank\\"><strong>Top-down attention in end-to-end spoken language understanding</strong></a><br />\\nYixin Chen, <a href=\\"https://www.amazon.science/author/weiyi-lu\\" target=\\"_blank\\">Weiyi Lu</a>, <a href=\\"https://www.amazon.science/author/alejandro-mottini\\" target=\\"_blank\\">Alejandro Mottini </a>, <a href=\\"https://www.amazon.science/author/erran-li\\" target=\\"_blank\\">Erran Li</a>, <a href=\\"https://www.amazon.science/author/jasha-droppo\\" target=\\"_blank\\">Jasha Droppo</a>, <a href=\\"https://www.amazon.science/author/zheng-du\\" target=\\"_blank\\">Zheng Du</a>, <a href=\\"https://www.amazon.science/author/belinda-zeng\\" target=\\"_blank\\">Belinda Zeng</a></p>\\n</li>\n</ul>\\n<p><img src=\\"https://dev-media.amazoncloud.cn/f753621dbe374383bf89768b087db820_image.png\\" alt=\\"image.png\\" /></p>\n<p>A spoken-language-understanding system combines automatic speech recognition (ASR) and natural-language understanding (NLU) in a single model.</p>\n<p>FROM <a href=\\"https://www.amazon.science/publications/do-as-i-mean-not-as-i-say-sequence-loss-training-for-spoken-language-understanding\\" target=\\"_blank\\">“DO AS I MEAN, NOT AS I SAY: SEQUENCE LOSS TRAINING FOR SPOKEN LANGUAGE UNDERSTANDING”</a></p>\\n<p>An interaction with a voice service, which begins with keyword spotting, ASR, and NLU, often culminates with the agent’s use of synthesized speech to relay a response. The agent’s <strong>text-to-speech</strong> model converts the textual outputs of various NLU and dialogue systems into speech:</p>\\n<ul>\\n<li>\\n<p><a href=\\"https://www.amazon.science/publications/camp-a-two-stage-approach-to-modelling-prosody-in-context\\" target=\\"_blank\\"><strong>CAMP: A two-stage approach to modelling prosody in context</strong></a><br />\\nZack Hodari, <a href=\\"https://www.amazon.science/author/alexis-moinet\\" target=\\"_blank\\">Alexis Moinet</a>, <a href=\\"https://www.amazon.science/author/sri-karlapati\\" target=\\"_blank\\">Sri Karlapati</a>, <a href=\\"https://www.amazon.science/author/jaime-lorenzo-trueba\\" target=\\"_blank\\">Jaime Lorenzo-Trueba</a>, <a href=\\"https://www.amazon.science/author/thomas-merritt\\" target=\\"_blank\\">Thomas Merritt</a>, <a href=\\"https://www.amazon.science/author/arnaud-joly\\" target=\\"_blank\\">Arnaud Joly</a>, <a href=\\"https://www.amazon.science/author/ammar-abbas\\" target=\\"_blank\\">Ammar Abbas</a>, <a href=\\"https://www.amazon.science/author/penny-karanasou\\" target=\\"_blank\\">Penny Karanasou</a>, <a href=\\"https://www.amazon.science/author/thomas-drugman\\" target=\\"_blank\\">Thomas Drugman</a></p>\\n</li>\n<li>\\n<p><a href=\\"https://www.amazon.science/publications/low-resource-expressive-text-to-speech-using-data-augmentation\\" target=\\"_blank\\"><strong>Low-resource expressive text-to-speech using data augmentation</strong></a><br />\\n<a href=\\"https://www.amazon.science/author/goeric-huybrechts\\" target=\\"_blank\\">Goeric Huybrechts</a>, <a href=\\"https://www.amazon.science/author/thomas-merritt\\" target=\\"_blank\\">Thomas Merritt</a>, <a href=\\"https://www.amazon.science/author/giulia-comini\\" target=\\"_blank\\">Giulia Comini</a>, <a href=\\"https://www.amazon.science/author/bartek-perz\\" target=\\"_blank\\">Bartek Perz</a>, <a href=\\"https://www.amazon.science/author/raahil-shah\\" target=\\"_blank\\">Raahil Shah</a>, <a href=\\"https://www.amazon.science/author/jaime-lorenzo-trueba\\" target=\\"_blank\\">Jaime Lorenzo-Trueba</a></p>\\n</li>\n<li>\\n<p><a href=\\"https://www.amazon.science/publications/prosodic-representation-learning-and-contextual-sampling-for-neural-text-to-speech\\" target=\\"_blank\\"><strong>Prosodic representation learning and contextual sampling for neural text-to-speech</strong></a><br />\\n<a href=\\"https://www.amazon.science/author/sri-karlapati\\" target=\\"_blank\\">Sri Karlapati</a>, <a href=\\"\\" target=\\"_blank\\">Ammar Abbas</a>, Zack Hodari], <a href=\\"https://www.amazon.science/author/alexis-moinet\\" target=\\"_blank\\">Alexis Moinet</a>, <a href=\\"https://www.amazon.science/author/arnaud-joly\\" target=\\"_blank\\">Arnaud Joly</a>, <a href=\\"https://www.amazon.science/author/penny-karanasou\\" target=\\"_blank\\">Penny Karanasou</a>, <a href=\\"https://www.amazon.science/author/thomas-drugman\\" target=\\"_blank\\">Thomas Drugman</a></p>\\n</li>\n<li>\\n<p><a href=\\"https://www.amazon.science/publications/universal-neural-vocoding-with-parallel-wavenet\\" target=\\"_blank\\"><strong>Universal neural vocoding with Parallel WaveNet</strong></a><br />\\n<a href=\\"https://www.amazon.science/author/yunlong-jiao\\" target=\\"_blank\\">Yunlong Jiao</a>, <a href=\\"https://www.amazon.science/author/adam-gabrys\\" target=\\"_blank\\">Adam Gabrys</a>, Georgi Tinchev, <a href=\\"https://www.amazon.science/author/bartosz-putrycz\\" target=\\"_blank\\">Bartosz Putrycz</a>, <a href=\\"https://www.amazon.science/author/daniel-korzekwa\\" target=\\"_blank\\">Daniel Korzekwa</a>, <a href=\\"https://www.amazon.science/author/viacheslav-klimkov\\" target=\\"_blank\\">Viacheslav Klimkov</a></p>\\n</li>\n</ul>\\n<p>All of the preceding research topics have implications for voice services like Alexa, but Amazon has a range of other products and services that rely on audio-signal processing. Three of Amazon’s papers at this year’s ICASSP relate to <strong>audio-video synchronization</strong>: two deal with dubbing audio in one language onto video shot in another, and one describes how to detect synchronization errors in video — as when, for example, the sound of a tennis ball being struck and the shot of the racquet hitting the ball are misaligned:</p>\\n<ul>\\n<li>\\n<p><a href=\\"https://www.amazon.science/publications/detection-of-audio-video-synchronization-errors-via-event-detection\\" target=\\"_blank\\"><strong>Detection of audio-video synchronization errors via event detection</strong></a><br />\\nJoshua P. Ebenezer, <a href=\\"https://www.amazon.science/author/yongjun-wu\\" target=\\"_blank\\">Yongjun Wu</a>, <a href=\\"https://www.amazon.science/author/hai-wei\\" target=\\"_blank\\">Hai Wei</a>, <a href=\\"https://www.amazon.science/author/sriram-sethuraman\\" target=\\"_blank\\">Sriram Sethuraman</a>, <a href=\\"https://www.amazon.science/author/zongyi-liu\\" target=\\"_blank\\">Zongyi Liu </a></p>\\n</li>\n<li>\\n<p><a href=\\"https://www.amazon.science/publications/improvements-to-prosodic-alignment-for-automatic-dubbing\\" target=\\"_blank\\"><strong>Improvements to prosodic alignment for automatic dubbing</strong></a><br />\\n<a href=\\"https://www.amazon.science/author/yogesh-virkar\\" target=\\"_blank\\">Yogesh Virkar</a>, <a href=\\"https://www.amazon.science/author/marcello-federico\\" target=\\"_blank\\">Marcello Federico</a>, <a href=\\"https://www.amazon.science/author/robert-enyedi\\" target=\\"_blank\\">Robert Enyedi</a>, <a href=\\"https://www.amazon.science/author/roberto-barra-chicote\\" target=\\"_blank\\">Roberto Barra-Chicote</a></p>\\n</li>\n<li>\\n<p><a href=\\"https://www.amazon.science/publications/machine-translation-verbosity-control-for-automatic-dubbing\\" target=\\"_blank\\"><strong>Machine translation verbosity control for automatic dubbing</strong></a><br />\\n<a href=\\"https://www.amazon.science/https:/www.amazon.science/author/surafel-melaku-lakew\\" target=\\"_blank\\">Surafel Melaku Lakew</a>, <a href=\\"https://www.amazon.science/author/marcello-federico\\" target=\\"_blank\\">Marcello Federico</a>, <a href=\\"https://www.amazon.science/author/yue-wang\\" target=\\"_blank\\">Yue Wang</a>, <a href=\\"https://www.amazon.science/author/cuong-hoang\\" target=\\"_blank\\">Cuong Hoang</a>, <a href=\\"https://www.amazon.science/author/yogesh-virkar\\" target=\\"_blank\\">Yogesh Virkar</a>, <a href=\\"https://www.amazon.science/author/roberto-barra-chicote\\" target=\\"_blank\\">Roberto Barra-Chicote</a>, <a href=\\"https://www.amazon.science/author/robert-enyedi\\" target=\\"_blank\\">Robert Enyedi </a></p>\\n</li>\n</ul>\\n<p>Amazon’s Text-to-Speech team has an ICASSP paper on the unusual topic of <strong>computer-assisted pronunciation training</strong>, a feature of some language learning applications. The researchers’ method would enable language learning apps to accept a wider range of word pronunciations, to score pronunciations more accurately, and to provide more reliable feedback:</p>\\n<ul>\\n<li><a href=\\"https://www.amazon.science/publications/mispronunciation-detection-in-non-native-l2-english-with-uncertainty-modeling\\" target=\\"_blank\\"><strong>Mispronunciation detection in non-native (L2) English with uncertainty modeling</strong></a><br />\\n<a href=\\"https://www.amazon.science/author/daniel-korzekwa\\" target=\\"_blank\\">Daniel Korzekwa</a>, <a href=\\"https://www.amazon.science/author/jaime-lorenzo-trueba\\" target=\\"_blank\\">Jaime Lorenzo-Trueba</a>, Szymon Zaporowski, <a href=\\"https://www.amazon.science/author/shira-calamaro\\" target=\\"_blank\\">Shira Calamaro</a>, <a href=\\"https://www.amazon.science/author/thomas-drugman\\" target=\\"_blank\\">Thomas Drugman</a>, Bozena Kostek</li>\\n</ul>\n<p><img src=\\"https://dev-media.amazoncloud.cn/8c27775d555241e6a158f61419d36e54_image.png\\" alt=\\"image.png\\" /></p>\n<p>The architecture of a new Amazon model for separating a recording’s vocal tracks and instrumental tracks.</p>\n<p>FROM <a href=\\"https://www.amazon.science/publications/semi-supervised-singing-voice-separation-with-noise-self-training\\" target=\\"_blank\\">“SEMI-SUPERVISED SINGING VOICE SEPARATION WITH NOISE SELF-TRAINING”</a></p>\\n<p>Another paper investigates the topic of <strong>singing voice separation</strong>, or separating vocal tracks from instrumental tracks in song recordings:</p>\\n<ul>\\n<li><a href=\\"https://www.amazon.science/publications/semi-supervised-singing-voice-separation-with-noise-self-training\\" target=\\"_blank\\"><strong>Semi-supervised singing voice separation with noise self-training</strong></a><br />\\nZhepei Wang, <a href=\\"https://www.amazon.science/author/ritwik-giri\\" target=\\"_blank\\">Ritwik Giri</a>, <a href=\\"https://www.amazon.science/author/umut-isik\\" target=\\"_blank\\">Umut Isik</a>, <a href=\\"https://www.amazon.science/author/jean-marc-valin\\" target=\\"_blank\\">Jean-Marc Valin</a>, <a href=\\"https://www.amazon.science/author/arvindh-krishnaswamy\\" target=\\"_blank\\">Arvindh Krishnaswamy </a></li>\\n</ul>\n<p>Finally, two of Amazon’s ICASSP papers, although they do evaluate applications in speech recognition and audio classification, present general <strong>machine learning methodologies</strong> that could apply to a range of problems. One paper investigates federated learning, a distributed-learning technique in which multiple servers, each with a different, local store of training data, collectively build a machine learning model without exchanging data. The other presents a new loss function for training classification models on synthetic data created by transforming real data — for instance, training a sound classification model with samples that have noise added to them artificially.</p>\\n<ul>\\n<li><a href=\\"https://www.amazon.science/publications/cross-silo-federated-training-in-the-cloud-with-diversity-scaling-and-semi-supervised-learning\\" target=\\"_blank\\"><strong>Cross-silo federated training in the cloud with diversity scaling and semi-supervised learning</strong></a><br />\\n<a href=\\"https://www.amazon.science/author/kishore-nandury\\" target=\\"_blank\\">Kishore Nandury</a>, <a href=\\"https://www.amazon.science/author/anand-mohan\\" target=\\"_blank\\">Anand Mohan</a>, <a href=\\"https://www.amazon.science/author/frederick-weber\\" target=\\"_blank\\">Frederick Weber</a></li>\\n<li><a href=\\"https://www.amazon.science/publications/enhancing-audio-augmentation-methods-with-consistency-learning\\" target=\\"_blank\\"><strong>Enhancing audio augmentation methods with consistency learning</strong></a><br />\\nTurab Iqbal, <a href=\\"https://www.amazon.science/author/karim-helwani\\" target=\\"_blank\\">Karim Helwani</a>, <a href=\\"https://www.amazon.science/author/arvindh-krishnaswamy\\" target=\\"_blank\\">Arvindh Krishnaswamy</a>, Wenwu Wang</li>\\n</ul>\n<p>Also at ICASSP, on June 8, seven Amazon scientists will be participating in a half-hour live Q&A. Conference registrants may <a href=\\"https://amazon.qualtrics.com/jfe/form/SV_0uf9M3PTwoL0Rfw\\" target=\\"_blank\\">submit questions to the panelists</a> online.</p>\\n<p>ABOUT THE AUTHOR</p>\n<h4><a id=\\"Larry_Hardestyhttpswwwamazonscienceauthorlarryhardesty_162\\"></a><a href=\\"https://www.amazon.science/author/larry-hardesty\\" target=\\"_blank\\">Larry Hardesty</a></h4>\\n<p>Larry Hardesty is the editor of the Amazon Science blog. Previously, he was a senior editor at MIT Technology Review and the computer science writer at the MIT News Office.</p>\n"}