{"value":"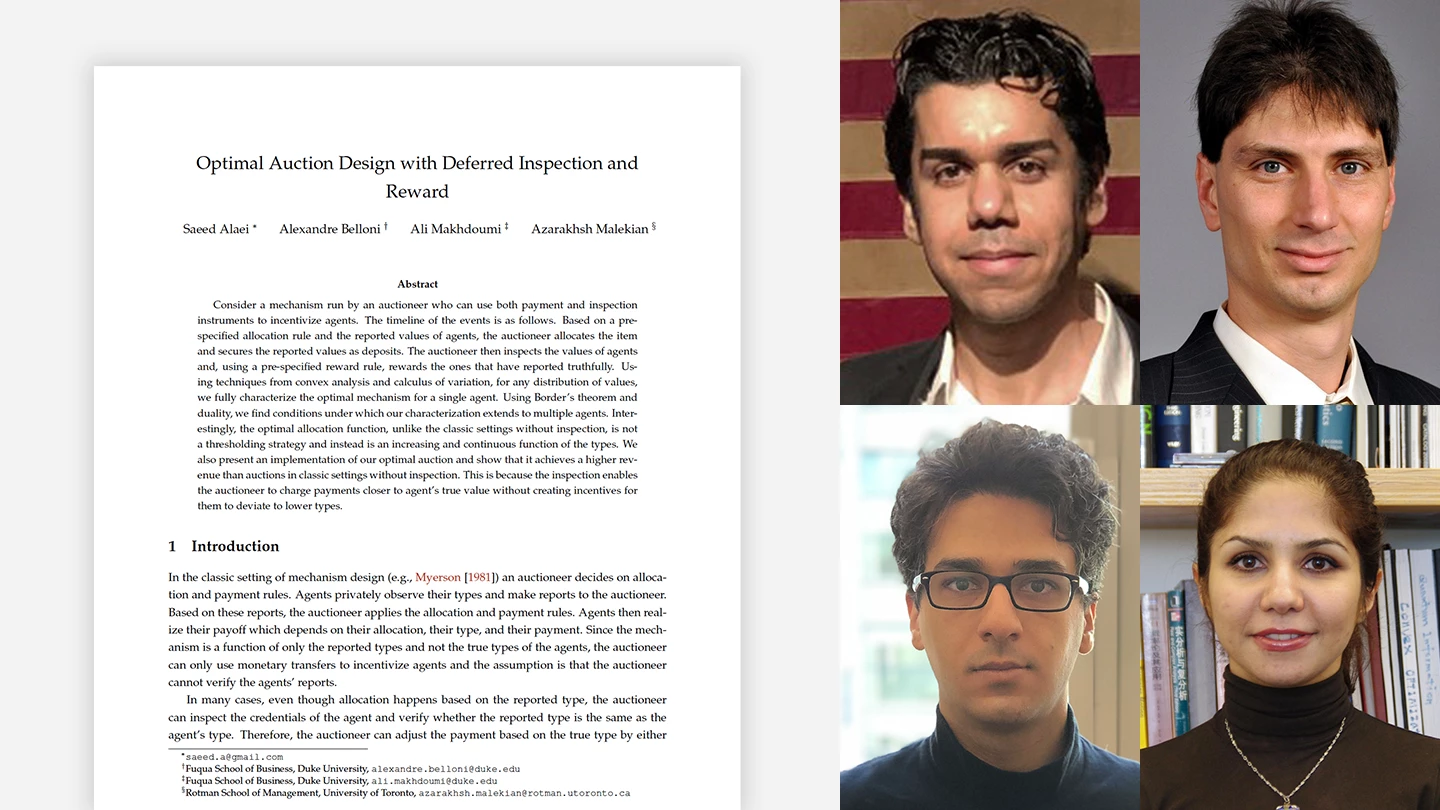\n\nIn their paper, \"[Optimal Auction Design with Deferred Inspection and Reward](https://papers.ssrn.com/sol3/papers.cfm?abstract_id=3700525)\", Saeed Alaei (top left), Alexandre Belloni (top right), Ali Makhdoumi (bottom left), and Azarakhsh Malekian (bottom right) developed a mechanism to incentivize buyers within an auction to bid higher by giving a bonus to bids whose value are closer to the true value of the item.\n\nAlexandre Belloni has been intrigued by operations research and optimization problems since his days at as an electrical engineering undergrad at the Pontifical Catholic University of Rio de Janeiro, back in his home country of Brazil. Further schooling just cemented that. His master’s in mathematical economics at the Institute for Pure and Applied Mathematics, also in Rio de Janeiro, “happened to have a strong optimization track,” he said. “Once I got there, the economics influence started to kick in,” he says. “And, given my background, I was always looking for the intersection of operations research and economics.”\n\nFor his PhD, Belloni worked on optimization and econometrics at the MIT Operations Research Center. His interest in economics continued to influence his academic path and most of his current research is focused on mechanism design problems, which he describes as “a broad class of ways to allocate resources.” “For example, auctions are a classic way that you can allocate an item and it is especially useful in cases where it’s difficult to price the value of the item.”\n\nBelloni says mechanism design is an incredible field to work on. “Not only there are many interesting perspectives to consider — such as information, computational, approximations, robustness, dynamics — but we also see several industry problems requiring to coordinate decentralized systems.”\n\nSince 2007, Belloni has also taught at the Fuqua Business School at Duke University, where he is currently the John D. Forsyth Professor of Decision Sciences. In 2018, he was recruited to become an [Amazon Scholar](https://www.amazon.science/scholars), joining the company in that capacity in January 2019. “I always thought that the best research is the one that is motivated by empirical, real problems. Amazon gives you a great opportunity to see the real problems,” he says.\n\nSince then, he has been studying problems related to mechanism design and machine learning at Fulfillment by Amazon (FBA), the subdivision of Amazon’s Supply Chain Optimization Technologies (SCOT) organization for third-party sellers who use Amazon’s storage and fulfillment capabilities.\n\nOne of the challenges Belloni and his FBA colleagues are currently addressing has to do with capacity management. Third-party sellers own and control their own inventories, and Amazon, with limited information, determines how to both balance the demand for space and ensure fulfillment center capacity is used efficiently and is available for products that customers love. “There has been tons of amazing work and we continue to obsess on finding better ways to manage capacity,” Belloni said.\n\nCoordinating and optimizing allocations is also at the core of a recent work by Belloni and colleagues. In the paper “[Optimal Auction Design with Deferred Inspection and Reward](https://papers.ssrn.com/sol3/papers.cfm?abstract_id=3700525)”, the authors develop a mechanism to incentivize buyers within an auction to bid higher by rewarding with a bonus the ones whose bids are closer to the true value of the item. This strategy can only be used in certain settings, where it is possible to monitor how the buyer is monetizing that good.\n\nIn this interview, Belloni discusses how he and his co-authors — Saeed Alaei, Ali Makhdoumi and Azarakhsh Malekian — came up with this new auction design that is especially suitable for digital goods and how it may impact revenues.\t\n\n#### **Q. What is the mechanism that you and your colleagues developed to optimize auction design? What are the implications for digital goods?**\n\n**A.** The key thing about this paper is that, in certain settings, after the winner of an auction is revealed, we can actually learn what is the true value of the good for the agent [buyer]. Indeed, there are many settings where the values are (nearly) observed with some delay. In those cases, if the agent said the truth — that is, the bid is close to the true value — we can give them a bonus back from their initial deposits.\n\nIt turns out that we were able to fully characterize the optimal mechanism for a single agent. By using rewards after the inspection to help us screen the agent, we found that the optimal allocation is not a thresholding strategy, and instead is an increasing and continuous function of the reported value. Indeed, it is possible to have different payments (via the rewards) for the same allocation, which contrasts with the case without inspection where no such mechanism would be incentive compatible.\n\nThe results are quite relevant in settings where it is possible to monitor the value (or performance) of the good for the bidder. Digital goods are certainly one application that motivated our setting. For example, consider a platform that would like to sell some preferred advertisement position for a digital good to be displayed. Because consumption of the digital good occurs within the platform, its value is observed, whether it is the winner of the specific auction or not.\n\nThus, the paper provides insights on how to monetize on this additional monitoring while still allowing agents to fully control the maximum they would be paying to acquire the preferred advertisement position. This is attractive as agents are always concerned with liability and, in practice, they could be reluctant to accept a contract in which they do not know how much they could end up paying. So, we are taking this concern into consideration. We monitor them, but we cannot charge more than whatever the amount they bid. The agents are in full control of how much they will spend. Ultimately, we are rewarding a digital good that has high value to be able to screen further via monitoring.\n\n#### **Q. How were you able to extrapolate your results from a single buyer to multiple buyers?**\n\n**A.** A priori, it was unclear how the results would generalize for the multiple-agents case given the generality of the first result. The first step was to consider the so-called reduced-form representation where we model the expected allocation and payments of a bidder condition on his or her own type (by averaging out over the types of the other bidders). But to ensure the reduced form is implementable as an auction, it is well known the additional Border constraints needed to be considered, which can get tricky.\nUsing duality theory, we then find a sufficient condition under which the Border constraint in the reduced form of the problem can be dealt with nicely. The sufficient technical condition on the hazard rate of the distribution of the maximum value is not needed in the single-agent case. Indeed, the result for a single agent holds quite generally. Surprisingly, the same structural properties in the single-agent case are still preserved in the multiple-agents case.\n\nImportantly, we provide an implementation of our optimal auction for multiple agents — Border constraints guarantee an implementation exists but do not tell us how. In particular, we show that the implementation of the optimal auction involves allocating to the agent with the maximum bid and then rewarding this agent if they report truthfully. One aspect of this setup with inspection is that we can further distinguish bidders by having more freedom to manipulate the amount of allocation and payments. In typical auctions, without inspection, there is no value to do that and agents either get the good or not. In our case, we can essentially give you the good with only 50% chance if you bid low, for example.\n\nIndeed, we increase the chance of allocating the good as the bids increase and when we reach 100% change we can further increase the reward for reporting correctly. So, if you think about a second price auction, for example, the agent pays the second-highest price, and that's it. Here, the monitoring allows us further screen bidders after they bid which allows us to refine the final payments through the bonus. Thus bidders have an additional incentive to pay more (even in a single-agent case) just to make sure that they will have a higher chance of getting the good.\n\n#### **Q. What impact does your optimization have on revenue? And how does that differ from auctions in classic settings?**\n\n**A.** This auction will, by design, generate higher revenues than the standard option (without monitoring). Intuitively, because of the bonus, if the agent tries to take advantage of you by bidding too low, they are not getting any bonus back. Now, if the agent tells you the truth, then they're going to get a decent bonus. So, this creates this incentive that makes them willing to push towards the true value.\n\nIn the paper, we present a nice characterization of why the revenue is going to be bigger. The typical idea in an auction is that you need to pay information rent for the agents. And what happens is that this monitoring reduces the information rent by design. More precisely, the information rent gets reduced by a factor related to the best alternative bid the agent could place. That comes out very clearly in the math.\n\nWe cannot say that we are going to do 20% or 30% more because that's very specific of the company. However, note that this will be particularly impactful with a small number of agents. Thin markets where there is a single bidder, for example, who could typically walk away with a lot of surplus. In specific settings (depending on distributions, number of agents, etc.) we provide examples in the paper where gains are significant. Nonetheless, we can clearly say that we always reduce the information rent.\n\nABOUT THE AUTHOR\n\n#### **Staff writer**","render":"<p><img src=\\"https://dev-media.amazoncloud.cn/f57d78c7e0014c63ab0db1de92cf97f3_image.png\\" alt=\\"image.png\\" /></p>\n<p>In their paper, “<a href=\\"https://papers.ssrn.com/sol3/papers.cfm?abstract_id=3700525\\" target=\\"_blank\\">Optimal Auction Design with Deferred Inspection and Reward</a>”, Saeed Alaei (top left), Alexandre Belloni (top right), Ali Makhdoumi (bottom left), and Azarakhsh Malekian (bottom right) developed a mechanism to incentivize buyers within an auction to bid higher by giving a bonus to bids whose value are closer to the true value of the item.</p>\\n<p>Alexandre Belloni has been intrigued by operations research and optimization problems since his days at as an electrical engineering undergrad at the Pontifical Catholic University of Rio de Janeiro, back in his home country of Brazil. Further schooling just cemented that. His master’s in mathematical economics at the Institute for Pure and Applied Mathematics, also in Rio de Janeiro, “happened to have a strong optimization track,” he said. “Once I got there, the economics influence started to kick in,” he says. “And, given my background, I was always looking for the intersection of operations research and economics.”</p>\n<p>For his PhD, Belloni worked on optimization and econometrics at the MIT Operations Research Center. His interest in economics continued to influence his academic path and most of his current research is focused on mechanism design problems, which he describes as “a broad class of ways to allocate resources.” “For example, auctions are a classic way that you can allocate an item and it is especially useful in cases where it’s difficult to price the value of the item.”</p>\n<p>Belloni says mechanism design is an incredible field to work on. “Not only there are many interesting perspectives to consider — such as information, computational, approximations, robustness, dynamics — but we also see several industry problems requiring to coordinate decentralized systems.”</p>\n<p>Since 2007, Belloni has also taught at the Fuqua Business School at Duke University, where he is currently the John D. Forsyth Professor of Decision Sciences. In 2018, he was recruited to become an <a href=\\"https://www.amazon.science/scholars\\" target=\\"_blank\\">Amazon Scholar</a>, joining the company in that capacity in January 2019. “I always thought that the best research is the one that is motivated by empirical, real problems. Amazon gives you a great opportunity to see the real problems,” he says.</p>\\n<p>Since then, he has been studying problems related to mechanism design and machine learning at Fulfillment by Amazon (FBA), the subdivision of Amazon’s Supply Chain Optimization Technologies (SCOT) organization for third-party sellers who use Amazon’s storage and fulfillment capabilities.</p>\n<p>One of the challenges Belloni and his FBA colleagues are currently addressing has to do with capacity management. Third-party sellers own and control their own inventories, and Amazon, with limited information, determines how to both balance the demand for space and ensure fulfillment center capacity is used efficiently and is available for products that customers love. “There has been tons of amazing work and we continue to obsess on finding better ways to manage capacity,” Belloni said.</p>\n<p>Coordinating and optimizing allocations is also at the core of a recent work by Belloni and colleagues. In the paper “<a href=\\"https://papers.ssrn.com/sol3/papers.cfm?abstract_id=3700525\\" target=\\"_blank\\">Optimal Auction Design with Deferred Inspection and Reward</a>”, the authors develop a mechanism to incentivize buyers within an auction to bid higher by rewarding with a bonus the ones whose bids are closer to the true value of the item. This strategy can only be used in certain settings, where it is possible to monitor how the buyer is monetizing that good.</p>\\n<p>In this interview, Belloni discusses how he and his co-authors — Saeed Alaei, Ali Makhdoumi and Azarakhsh Malekian — came up with this new auction design that is especially suitable for digital goods and how it may impact revenues.</p>\n<h4><a id=\\"Q_What_is_the_mechanism_that_you_and_your_colleagues_developed_to_optimize_auction_design_What_are_the_implications_for_digital_goods_20\\"></a><strong>Q. What is the mechanism that you and your colleagues developed to optimize auction design? What are the implications for digital goods?</strong></h4>\\n<p><strong>A.</strong> The key thing about this paper is that, in certain settings, after the winner of an auction is revealed, we can actually learn what is the true value of the good for the agent [buyer]. Indeed, there are many settings where the values are (nearly) observed with some delay. In those cases, if the agent said the truth — that is, the bid is close to the true value — we can give them a bonus back from their initial deposits.</p>\\n<p>It turns out that we were able to fully characterize the optimal mechanism for a single agent. By using rewards after the inspection to help us screen the agent, we found that the optimal allocation is not a thresholding strategy, and instead is an increasing and continuous function of the reported value. Indeed, it is possible to have different payments (via the rewards) for the same allocation, which contrasts with the case without inspection where no such mechanism would be incentive compatible.</p>\n<p>The results are quite relevant in settings where it is possible to monitor the value (or performance) of the good for the bidder. Digital goods are certainly one application that motivated our setting. For example, consider a platform that would like to sell some preferred advertisement position for a digital good to be displayed. Because consumption of the digital good occurs within the platform, its value is observed, whether it is the winner of the specific auction or not.</p>\n<p>Thus, the paper provides insights on how to monetize on this additional monitoring while still allowing agents to fully control the maximum they would be paying to acquire the preferred advertisement position. This is attractive as agents are always concerned with liability and, in practice, they could be reluctant to accept a contract in which they do not know how much they could end up paying. So, we are taking this concern into consideration. We monitor them, but we cannot charge more than whatever the amount they bid. The agents are in full control of how much they will spend. Ultimately, we are rewarding a digital good that has high value to be able to screen further via monitoring.</p>\n<h4><a id=\\"Q_How_were_you_able_to_extrapolate_your_results_from_a_single_buyer_to_multiple_buyers_30\\"></a><strong>Q. How were you able to extrapolate your results from a single buyer to multiple buyers?</strong></h4>\\n<p><strong>A.</strong> A priori, it was unclear how the results would generalize for the multiple-agents case given the generality of the first result. The first step was to consider the so-called reduced-form representation where we model the expected allocation and payments of a bidder condition on his or her own type (by averaging out over the types of the other bidders). But to ensure the reduced form is implementable as an auction, it is well known the additional Border constraints needed to be considered, which can get tricky.<br />\\nUsing duality theory, we then find a sufficient condition under which the Border constraint in the reduced form of the problem can be dealt with nicely. The sufficient technical condition on the hazard rate of the distribution of the maximum value is not needed in the single-agent case. Indeed, the result for a single agent holds quite generally. Surprisingly, the same structural properties in the single-agent case are still preserved in the multiple-agents case.</p>\n<p>Importantly, we provide an implementation of our optimal auction for multiple agents — Border constraints guarantee an implementation exists but do not tell us how. In particular, we show that the implementation of the optimal auction involves allocating to the agent with the maximum bid and then rewarding this agent if they report truthfully. One aspect of this setup with inspection is that we can further distinguish bidders by having more freedom to manipulate the amount of allocation and payments. In typical auctions, without inspection, there is no value to do that and agents either get the good or not. In our case, we can essentially give you the good with only 50% chance if you bid low, for example.</p>\n<p>Indeed, we increase the chance of allocating the good as the bids increase and when we reach 100% change we can further increase the reward for reporting correctly. So, if you think about a second price auction, for example, the agent pays the second-highest price, and that’s it. Here, the monitoring allows us further screen bidders after they bid which allows us to refine the final payments through the bonus. Thus bidders have an additional incentive to pay more (even in a single-agent case) just to make sure that they will have a higher chance of getting the good.</p>\n<h4><a id=\\"Q_What_impact_does_your_optimization_have_on_revenue_And_how_does_that_differ_from_auctions_in_classic_settings_39\\"></a><strong>Q. What impact does your optimization have on revenue? And how does that differ from auctions in classic settings?</strong></h4>\\n<p><strong>A.</strong> This auction will, by design, generate higher revenues than the standard option (without monitoring). Intuitively, because of the bonus, if the agent tries to take advantage of you by bidding too low, they are not getting any bonus back. Now, if the agent tells you the truth, then they’re going to get a decent bonus. So, this creates this incentive that makes them willing to push towards the true value.</p>\\n<p>In the paper, we present a nice characterization of why the revenue is going to be bigger. The typical idea in an auction is that you need to pay information rent for the agents. And what happens is that this monitoring reduces the information rent by design. More precisely, the information rent gets reduced by a factor related to the best alternative bid the agent could place. That comes out very clearly in the math.</p>\n<p>We cannot say that we are going to do 20% or 30% more because that’s very specific of the company. However, note that this will be particularly impactful with a small number of agents. Thin markets where there is a single bidder, for example, who could typically walk away with a lot of surplus. In specific settings (depending on distributions, number of agents, etc.) we provide examples in the paper where gains are significant. Nonetheless, we can clearly say that we always reduce the information rent.</p>\n<p>ABOUT THE AUTHOR</p>\n<h4><a id=\\"Staff_writer_49\\"></a><strong>Staff writer</strong></h4>\n"}