{"value":"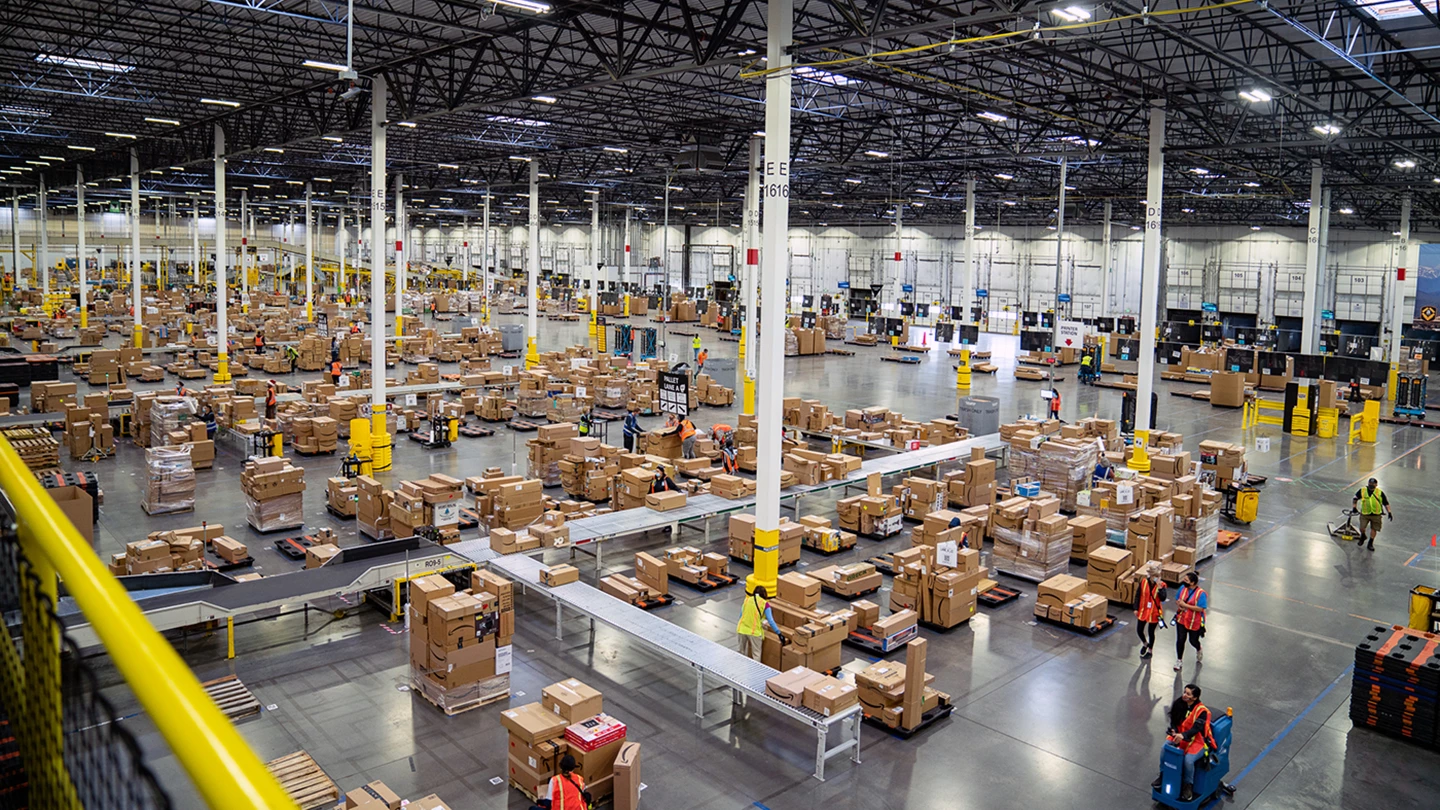\n\nAmazon's scale makes picking the right package for each product a challenge. Fortunately, machine learning approaches — particularly deep learning — thrive on big data and massive scale. These tools have helped Amazon reduce per-shipment packaging weight by 36% and eliminate more than a million tons of packaging.\n\nFinding the right amount of packaging to ship an item can be challenging — and at Amazon, an ever-changing catalog of hundreds of millions of products makes it an ongoing challenge. In addition, Amazon’s scale also means it is impossible to solve this challenge using manual inspection to choose packaging for each and every item. For the same reason, general packaging rules and run-of-the-mill logic just won’t cut it. What’s required is a cutting-edge-smart automated mechanism that can adapt on the fly to changing circumstances.\n\nFortunately, machine learning approaches — particularly deep learning — thrive on big data and massive scale, and a pioneering combination of natural language processing and computer vision is enabling Amazon to hone in on using the right amount of packaging. These tools have helped Amazon drive change over the past six years, reducing per-shipment packaging weight by 36% and eliminating more than a million tons of packaging, equivalent to more than 2 billion shipping boxes.\n\n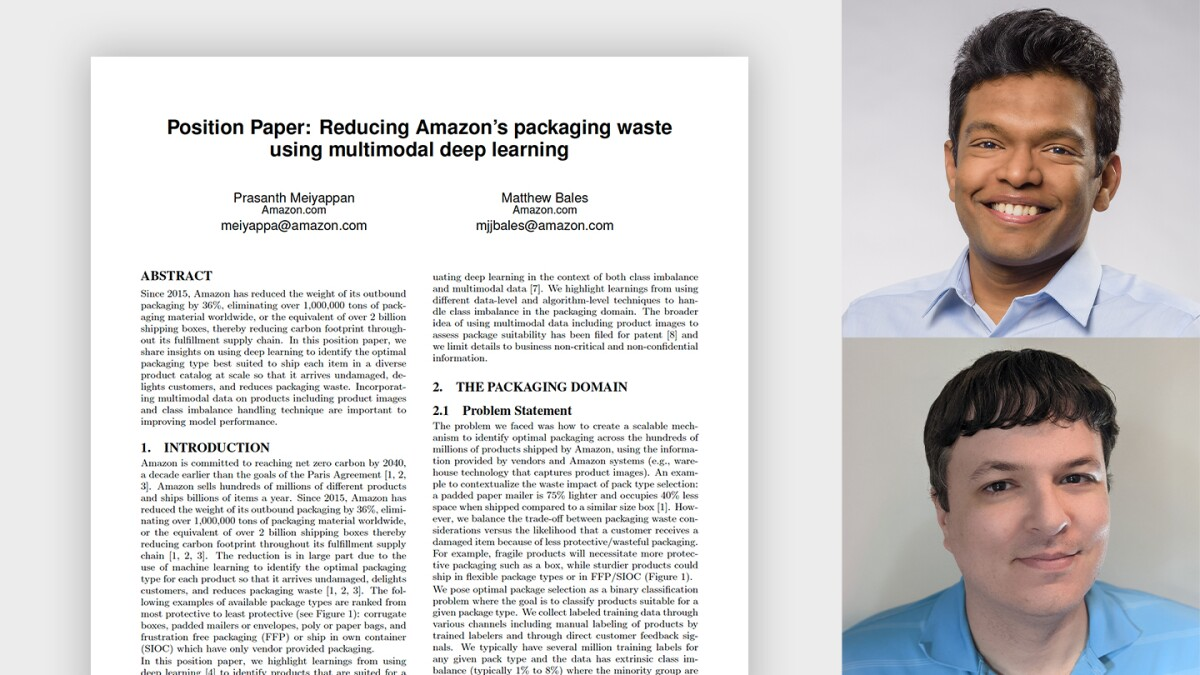\n\nPrasanth Meiyappan, top right, an applied scientist, and Matthew Bales, a research science manager, authored \"[Reducing Amazon’s packaging waste using multimodal deep learning](https://www.amazon.science/publications/position-paper-reducing-amazons-packaging-wasteusing-multimodal-deep-learning)\". Their position paper was one of [the 10 most read research papers on Amazon Science](https://www.amazon.science/latest-news/the-10-most-read-research-papers-of-2021) in 2021.\n\n“When I started at Amazon in 2017, we had a lot of physical testing of products going on, but not a scalable mechanism that could assess hundreds of millions of products to identify the optimal packaging type for each product,” says research science manager [Matthew Bales](). Bales, who is also a physicist, heads up machine learning within Amazon’s Customer Packaging Experience team.\n\n“Statistical tests were the first piece, but they are essentially only useful when products have already been shipped in more than one package type. We wanted the capability to predict how a product would fare in a less-protective, lighter, and more sustainable package type. And once you're in that predictive space, you need machine learning,” Bales explains.\n\n#### **The power of customer feedback**\n\nTo make a prediction about whether a given product could be safely shipped in a particular package type, Bales and his colleagues built a ML model based largely on the text-based data that customers find on the Amazon Store — the item name, description, price, package dimensions, and so on.\n\nSense, Act, and Scale \nRelated content\nCreating sustainable, data-driven buildings\nThe model was trained on millions of examples of products successfully delivered in various packaging types, and on examples of products that arrived damaged in given packaging types. Amazon has access to almost real-time feedback when a product is not sufficiently protected by its packaging, because customers report it via the Online Returns Center and other forms of feedback, including product reviews.\n\n“Customer feedback is paramount,” says Bales. “It powers all of our statistical testing.”\n\nThe model learned that certain keywords were particularly important when making packaging decisions. For example, keywords that indicated that a padded mailer would not be the right packaging included “ceramic”, “grocery”, “mug” and “glass”. These products were better shipped in a box. Keywords that suggested mailers were the right choice included “multipack” and “bag.” Those indicated the product might already have some form of protective packaging.\n\n“The portion of the model that's learning from the Amazon Store has learned really well what the product is, and about its dimensions,” says Bales.\n\n<video src=\\"https://dev-media.amazoncloud.cn/e75bc18af80c498198546029544c70f2_Rec%200008.mp4\\" class=\\"manvaVedio\\" controls=\\"controls\\" style=\\"width:160px;height:160px\\"></video>\n\n**Reducing Amazon’s packaging waste using multimodal deep learning**\n\nIt’s an important step in the journey, but automatically learning what a product is represents only half the battle. Equally important is how the vendor packaged the product before sending it to a fulfillment center. For example, a ceramic mug may be packaged in clear plastic bag, or in a sturdy box.\n\nTo identify product packaging at scale, computer vision needed to be deployed. The ML team already knew that the product images on the Amazon Store weren’t helpful when selecting packaging. For example, a multipack of LED bulbs might be illustrated by a picture of a single, unpacked bulb, suggesting it is fragile, yet the multipack is, in fact, safely packaged by the vendor and doesn’t require additional packaging. It is best shipped in its own container.\n\nBales’s team addressed this challenge by using Amazon’s own image data. When products are delivered to fulfillment centers, many are sent via conveyor belt through special computer-vision tunnels equipped with cameras that capture images of the products from multiple angles. These tunnels are used for many things, including ascertaining product dimensions and spotting defects.\n\n[Prasanth Meiyappan](https://www.linkedin.com/in/pmeiyappan/), an Amazon applied scientist, expanded the training of the team’s ML model to include these standardized product images in addition to the text classifiers from the catalog — a multimodal approach.\n\n“Our model detects the packaging edges to determine shape, identifies a perforation, a bag around the product, or light shining through a glass bottle.” Meiyappan explains. But to some extent, how the model makes its judgement about what it detects in images is hard for a human to discern, because the product features identified and weighted by the model tend to be complex.\n\n“The important thing,” Bales notes, “is that the packaging decisions generated by the model are empirically accurate.”\n\nIncorporating both text-based and visual data improved the ML model’s performance by as much as 30%, compared with using text-based data alone. Bales and Meiyappan have [produced a position paper](https://www.amazon.science/publications/position-paper-reducing-amazons-packaging-wasteusing-multimodal-deep-learning) describing their work.\n\n#### Our model detects the packaging edges to determine shape, identifies a perforation, a bag around the product, or light shining through a glass bottle.\n\nPrasanth Meiyappan\n\n“When the model is certain of the best package type for a given product, we allow it to auto-certify it for that pack type,” says Bales. “When the model is less certain, it flags a product and its packaging for testing by a human.” The technology is currently being applied to product lines across North America, Europe, and Japan — automatically reducing waste at a growing scale.\n\n“It’s a triple win,” says Bales. “Reduced waste, increased customer satisfaction, and lower costs.”\n\n#### Balancing act\n\nTo arrive at this triple win, though, the team also had to take on a thorny challenge encountered frequently in the ML domain: class imbalance. In a nutshell, the problem is this: if you want an ML model to learn effectively, you ideally provide it with as many examples of failures as successes, so it can learn to differentiate effectively between the two.\n\nThe data used to train the model had many millions of examples of product/package pairings, yet depending on the package type, as little as 1% of those examples were for packages that turned out to be unsuitable in some way for the product within.\n\n#### The machine learning literature to do with packaging is pretty sparse. Not many people deal with the kind of datasets we are dealing with in the packaging domain.\n\nPrasanth Meiyappan\n\n“Prior to implementing ML, we’ve shipped some product in envelopes and mailers for some time,” says Bales. “So, we had loads of examples of things that were good in mailers, but didn't have a lot of examples of things that were bad in mailers. ML models have problems with this kind of overwhelming imbalance.”\n\n“The machine learning literature to do with packaging is pretty sparse,” Meiyappan says. “Not many people deal with the kind of datasets we are dealing with in the packaging domain. How effective a technique is in dealing with dataset imbalance is both domain and dataset specific.”\n\nThus the team’s approach to the class imbalance problem was primarily experimental. And of the six approaches they applied — four data based, two algorithm based — the clear winner produced a marked improvement in model accuracy. That was a data-based approach called two-phase learning with random under sampling which focuses the model on the minority class in the first phase of training and then on all of the data in the second. “In our position paper we share that knowledge with the ML community,” says Bales, “so that anyone who encounters a similar problem might choose to try this approach for themselves, to see if it also works in their problem space.”\n\n#### What’s next\n\nhe team said they are eager to expand the use of this tool by training the model to understand all Amazon’s customers languages while also incorporating the unique aspects of fulfilment in each country.\n\nWhile Amazon scientists continue to research other ways to utilize machine learning to eliminate waste, the company is also working to reduce packaging waste throughout the e-commerce supply chain. Amazon is, for example, [increasingly incentivizing](https://www.aboutamazon.com/packaging/overview/2021-incentive) its vendors to create optimized e-commerce packaging for themselves that saves space and materials without compromising product protection.\n\n#### Read the Amazon Sustainability Report\n\nAmazon is committed to building a sustainable business for customers and the planet. [Learn more about Amazon's goals, strategies, and policies in the Amazon Sustainability Report](https://sustainability.aboutamazon.com/).\n\nThe company’s [Shipment Zero](https://sustainability.aboutamazon.com/environment/sustainable-operations/shipment-zero) goal is to deliver 50% of shipments with net-zero carbon by 2030, which from a packaging perspective means shipping products without added Amazon packaging or in carbon-neutral packaging. This is part of the Amazon’s wider [Climate Pledge](https://www.aboutamazon.com/planet/climate-pledge) — a commitment to reach net-zero carbon by 2040, a decade earlier than the 2050 emissions target of the Paris Agreement.\n\nABOUT THE AUTHOR\n\n[**Sean O'Neill**](https://www.amazon.science/author/sean-oneill)\n\nSean O’Neill is a writer, editor, and science communicator based near Bristol, UK.","render":"<p><img src=\\"https://dev-media.amazoncloud.cn/aa54ba6bfa934f469733aa525d5cebec_image.png\\" alt=\\"image.png\\" /></p>\n<p>Amazon’s scale makes picking the right package for each product a challenge. Fortunately, machine learning approaches — particularly deep learning — thrive on big data and massive scale. These tools have helped Amazon reduce per-shipment packaging weight by 36% and eliminate more than a million tons of packaging.</p>\n<p>Finding the right amount of packaging to ship an item can be challenging — and at Amazon, an ever-changing catalog of hundreds of millions of products makes it an ongoing challenge. In addition, Amazon’s scale also means it is impossible to solve this challenge using manual inspection to choose packaging for each and every item. For the same reason, general packaging rules and run-of-the-mill logic just won’t cut it. What’s required is a cutting-edge-smart automated mechanism that can adapt on the fly to changing circumstances.</p>\n<p>Fortunately, machine learning approaches — particularly deep learning — thrive on big data and massive scale, and a pioneering combination of natural language processing and computer vision is enabling Amazon to hone in on using the right amount of packaging. These tools have helped Amazon drive change over the past six years, reducing per-shipment packaging weight by 36% and eliminating more than a million tons of packaging, equivalent to more than 2 billion shipping boxes.</p>\n<p><img src=\\"https://dev-media.amazoncloud.cn/f76993025d014980b8202952a7b316ba_image.png\\" alt=\\"image.png\\" /></p>\n<p>Prasanth Meiyappan, top right, an applied scientist, and Matthew Bales, a research science manager, authored “<a href=\\"https://www.amazon.science/publications/position-paper-reducing-amazons-packaging-wasteusing-multimodal-deep-learning\\" target=\\"_blank\\">Reducing Amazon’s packaging waste using multimodal deep learning</a>”. Their position paper was one of <a href=\\"https://www.amazon.science/latest-news/the-10-most-read-research-papers-of-2021\\" target=\\"_blank\\">the 10 most read research papers on Amazon Science</a> in 2021.</p>\\n<p>“When I started at Amazon in 2017, we had a lot of physical testing of products going on, but not a scalable mechanism that could assess hundreds of millions of products to identify the optimal packaging type for each product,” says research science manager <a href=\\"\\" target=\\"_blank\\">Matthew Bales</a>. Bales, who is also a physicist, heads up machine learning within Amazon’s Customer Packaging Experience team.</p>\\n<p>“Statistical tests were the first piece, but they are essentially only useful when products have already been shipped in more than one package type. We wanted the capability to predict how a product would fare in a less-protective, lighter, and more sustainable package type. And once you’re in that predictive space, you need machine learning,” Bales explains.</p>\n<h4><a id=\\"The_power_of_customer_feedback_16\\"></a><strong>The power of customer feedback</strong></h4>\\n<p>To make a prediction about whether a given product could be safely shipped in a particular package type, Bales and his colleagues built a ML model based largely on the text-based data that customers find on the Amazon Store — the item name, description, price, package dimensions, and so on.</p>\n<p>Sense, Act, and Scale<br />\\nRelated content<br />\\nCreating sustainable, data-driven buildings<br />\\nThe model was trained on millions of examples of products successfully delivered in various packaging types, and on examples of products that arrived damaged in given packaging types. Amazon has access to almost real-time feedback when a product is not sufficiently protected by its packaging, because customers report it via the Online Returns Center and other forms of feedback, including product reviews.</p>\n<p>“Customer feedback is paramount,” says Bales. “It powers all of our statistical testing.”</p>\n<p>The model learned that certain keywords were particularly important when making packaging decisions. For example, keywords that indicated that a padded mailer would not be the right packaging included “ceramic”, “grocery”, “mug” and “glass”. These products were better shipped in a box. Keywords that suggested mailers were the right choice included “multipack” and “bag.” Those indicated the product might already have some form of protective packaging.</p>\n<p>“The portion of the model that’s learning from the Amazon Store has learned really well what the product is, and about its dimensions,” says Bales.</p>\n<p><video src=\\"https://dev-media.amazoncloud.cn/e75bc18af80c498198546029544c70f2_Rec%200008.mp4\\" controls=\\"controls\\"></video></p>\\n<p><strong>Reducing Amazon’s packaging waste using multimodal deep learning</strong></p>\\n<p>It’s an important step in the journey, but automatically learning what a product is represents only half the battle. Equally important is how the vendor packaged the product before sending it to a fulfillment center. For example, a ceramic mug may be packaged in clear plastic bag, or in a sturdy box.</p>\n<p>To identify product packaging at scale, computer vision needed to be deployed. The ML team already knew that the product images on the Amazon Store weren’t helpful when selecting packaging. For example, a multipack of LED bulbs might be illustrated by a picture of a single, unpacked bulb, suggesting it is fragile, yet the multipack is, in fact, safely packaged by the vendor and doesn’t require additional packaging. It is best shipped in its own container.</p>\n<p>Bales’s team addressed this challenge by using Amazon’s own image data. When products are delivered to fulfillment centers, many are sent via conveyor belt through special computer-vision tunnels equipped with cameras that capture images of the products from multiple angles. These tunnels are used for many things, including ascertaining product dimensions and spotting defects.</p>\n<p><a href=\\"https://www.linkedin.com/in/pmeiyappan/\\" target=\\"_blank\\">Prasanth Meiyappan</a>, an Amazon applied scientist, expanded the training of the team’s ML model to include these standardized product images in addition to the text classifiers from the catalog — a multimodal approach.</p>\\n<p>“Our model detects the packaging edges to determine shape, identifies a perforation, a bag around the product, or light shining through a glass bottle.” Meiyappan explains. But to some extent, how the model makes its judgement about what it detects in images is hard for a human to discern, because the product features identified and weighted by the model tend to be complex.</p>\n<p>“The important thing,” Bales notes, “is that the packaging decisions generated by the model are empirically accurate.”</p>\n<p>Incorporating both text-based and visual data improved the ML model’s performance by as much as 30%, compared with using text-based data alone. Bales and Meiyappan have <a href=\\"https://www.amazon.science/publications/position-paper-reducing-amazons-packaging-wasteusing-multimodal-deep-learning\\" target=\\"_blank\\">produced a position paper</a> describing their work.</p>\\n<h4><a id=\\"Our_model_detects_the_packaging_edges_to_determine_shape_identifies_a_perforation_a_bag_around_the_product_or_light_shining_through_a_glass_bottle_49\\"></a>Our model detects the packaging edges to determine shape, identifies a perforation, a bag around the product, or light shining through a glass bottle.</h4>\\n<p>Prasanth Meiyappan</p>\n<p>“When the model is certain of the best package type for a given product, we allow it to auto-certify it for that pack type,” says Bales. “When the model is less certain, it flags a product and its packaging for testing by a human.” The technology is currently being applied to product lines across North America, Europe, and Japan — automatically reducing waste at a growing scale.</p>\n<p>“It’s a triple win,” says Bales. “Reduced waste, increased customer satisfaction, and lower costs.”</p>\n<h4><a id=\\"Balancing_act_57\\"></a>Balancing act</h4>\\n<p>To arrive at this triple win, though, the team also had to take on a thorny challenge encountered frequently in the ML domain: class imbalance. In a nutshell, the problem is this: if you want an ML model to learn effectively, you ideally provide it with as many examples of failures as successes, so it can learn to differentiate effectively between the two.</p>\n<p>The data used to train the model had many millions of examples of product/package pairings, yet depending on the package type, as little as 1% of those examples were for packages that turned out to be unsuitable in some way for the product within.</p>\n<h4><a id=\\"The_machine_learning_literature_to_do_with_packaging_is_pretty_sparse_Not_many_people_deal_with_the_kind_of_datasets_we_are_dealing_with_in_the_packaging_domain_63\\"></a>The machine learning literature to do with packaging is pretty sparse. Not many people deal with the kind of datasets we are dealing with in the packaging domain.</h4>\\n<p>Prasanth Meiyappan</p>\n<p>“Prior to implementing ML, we’ve shipped some product in envelopes and mailers for some time,” says Bales. “So, we had loads of examples of things that were good in mailers, but didn’t have a lot of examples of things that were bad in mailers. ML models have problems with this kind of overwhelming imbalance.”</p>\n<p>“The machine learning literature to do with packaging is pretty sparse,” Meiyappan says. “Not many people deal with the kind of datasets we are dealing with in the packaging domain. How effective a technique is in dealing with dataset imbalance is both domain and dataset specific.”</p>\n<p>Thus the team’s approach to the class imbalance problem was primarily experimental. And of the six approaches they applied — four data based, two algorithm based — the clear winner produced a marked improvement in model accuracy. That was a data-based approach called two-phase learning with random under sampling which focuses the model on the minority class in the first phase of training and then on all of the data in the second. “In our position paper we share that knowledge with the ML community,” says Bales, “so that anyone who encounters a similar problem might choose to try this approach for themselves, to see if it also works in their problem space.”</p>\n<h4><a id=\\"Whats_next_73\\"></a>What’s next</h4>\\n<p>he team said they are eager to expand the use of this tool by training the model to understand all Amazon’s customers languages while also incorporating the unique aspects of fulfilment in each country.</p>\n<p>While Amazon scientists continue to research other ways to utilize machine learning to eliminate waste, the company is also working to reduce packaging waste throughout the e-commerce supply chain. Amazon is, for example, <a href=\\"https://www.aboutamazon.com/packaging/overview/2021-incentive\\" target=\\"_blank\\">increasingly incentivizing</a> its vendors to create optimized e-commerce packaging for themselves that saves space and materials without compromising product protection.</p>\\n<h4><a id=\\"Read_the_Amazon_Sustainability_Report_79\\"></a>Read the Amazon Sustainability Report</h4>\\n<p>Amazon is committed to building a sustainable business for customers and the planet. <a href=\\"https://sustainability.aboutamazon.com/\\" target=\\"_blank\\">Learn more about Amazon’s goals, strategies, and policies in the Amazon Sustainability Report</a>.</p>\\n<p>The company’s <a href=\\"https://sustainability.aboutamazon.com/environment/sustainable-operations/shipment-zero\\" target=\\"_blank\\">Shipment Zero</a> goal is to deliver 50% of shipments with net-zero carbon by 2030, which from a packaging perspective means shipping products without added Amazon packaging or in carbon-neutral packaging. This is part of the Amazon’s wider <a href=\\"https://www.aboutamazon.com/planet/climate-pledge\\" target=\\"_blank\\">Climate Pledge</a> — a commitment to reach net-zero carbon by 2040, a decade earlier than the 2050 emissions target of the Paris Agreement.</p>\\n<p>ABOUT THE AUTHOR</p>\n<p><a href=\\"https://www.amazon.science/author/sean-oneill\\" target=\\"_blank\\"><strong>Sean O’Neill</strong></a></p>\n<p>Sean O’Neill is a writer, editor, and science communicator based near Bristol, UK.</p>\n"}