{"value":"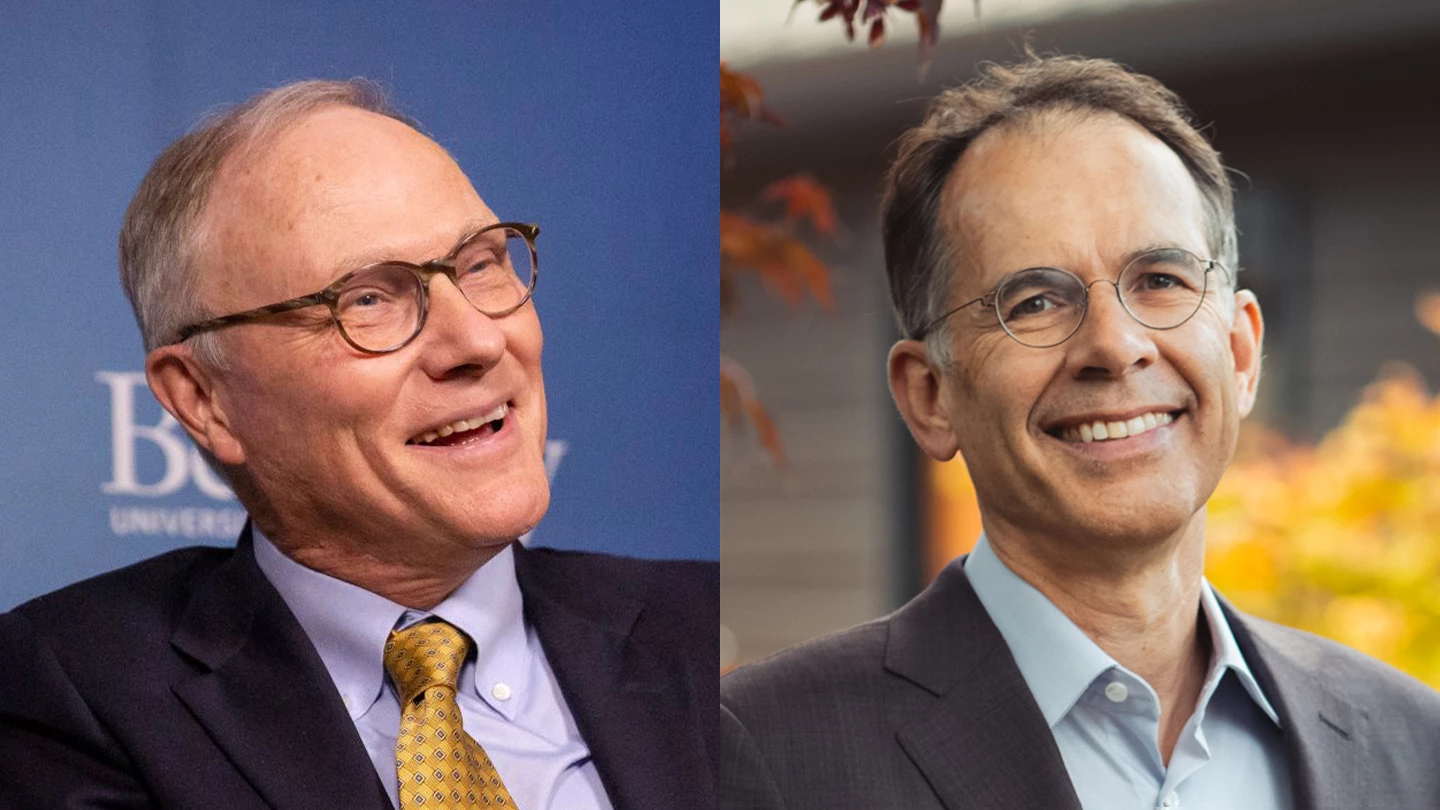\n\nDavid Card (left), an Amazon Scholar, a professor of economics at the University of California, Berkeley, and the outgoing president of the AEA, and Guido Imbens (right), an academic research consultant at Amazon and a professor at the Stanford Graduate School of Business.\n\nThe annual meeting of the American Economic Association (AEA) took place Jan. 7 - 9, and as it approached, Amazon Science had the chance to interview two of the three recipients of the 2021 Nobel Prize in economics — who also happen to be Amazon-affiliated economists.\n\nDavid Card, an Amazon Scholar, a professor of economics at the University of California, Berkeley, and the outgoing president of the AEA, won half the prize “for his empirical contributions to labor economics”.\n\nGuido Imbens, an academic research consultant at Amazon and a professor at the Stanford Graduate School of Business, shared the other half of the prize with MIT’s [Josh Angrist](https://news.mit.edu/2021/mit-economist-joshua-angrist-shares-nobel-prize-1011) for “methodological contributions to the analysis of causal relationships”.\n\n**Amazon Science:** The empirical approach to economics has been recognized by the Nobel Prize committee several times in the last few years, but it wasn't always as popular as it is today. I'm curious how you both first became interested in empirical approaches to economics.\n\n**David Card:** The heroes of economics for many, many decades were the theorists, and in the postwar era especially, there was a recognition that economic modeling was underdeveloped — the math was underdeveloped — and there was a need to formalize things and understand better what the models really delivered.\n\nThat need really proceeded through the ’60s, and Arrow and Debreu were these famous mathematical economists who developed some very elegant theoretical models of how the market works in an idealized economy.\n\nWhat happened in my time was people started to realize that we had the data to better look at real labor market phenomena and possibly make economics something different than just a kind of a branch of philosophy. [Arrow-Debreu ](https://en.wikipedia.org/wiki/Arrow%E2%80%93Debreu_model)is basically mathematical philosophy.\n\n**Guido Imbens:** I came from a very different tradition. I grew up in the Netherlands, and there was a strong tradition of econometrics started by people like Tinbergen. [Tinbergen](https://en.wikipedia.org/wiki/Jan_Tinbergen) had been very broad — he did econometrics, but he also did empirical work and was very heavily involved in policy analysis. But over time, the program he had started was becoming much more focused on technical econometrics.\n\n#### People started to realize that we had the data to better look at real labor market phenomena and possibly make economics something different than just a kind of a branch of philosophy.\n\nDavid Card\n\nSo as an undergraduate, we didn't really do any empirical work. We really just did a lot of mathematical statistics and some operations research and some economic theory. My thesis was a theoretical econometrics study.\n\nWhen I presented that at Harvard, Josh Angrist wasn't really all that impressed with it, and he actually opposed the department hiring me there because he thought the paper was boring. And he was probably right! But luckily, the more senior people there at the time thought I was at least somewhat promising. And so I got hired at Harvard. But then it was really Josh and [Larry Katz](https://scholar.harvard.edu/lkatz/home), one of the labor economists there, who got me interested in going to the labor seminar and got me exposed to the modern empirical work.\n\nThe context Josh and I started talking in really was this paper that I think came up in all three of the Nobel lectures, this paper by Ed Leamer, “[Let's Take the Con Out of Econometrics](https://www.jstor.org/stable/1803924?seq=1)”, where Leamer says, “Hardly anyone takes data analysis seriously. Or perhaps more accurately, hardly anyone takes anyone else’s data analysis seriously.”\n\nAnd I think Leamer was right: people did these very elaborate things, and it was all showing off complicated technical things, but it wasn't really very credible. In fact, Leamer presented a lecture based on that work at Harvard. And I remember Josh getting up at some point and saying, “Well, you talk about all this old stuff, but look at the work Card does. Look at the work Krueger does. Look at the work I do. It's very different.”\n\nAnd that felt right to me. It felt that the work was qualitatively very different from the work that Ed Leamer was describing and that he was complaining about.\n\n**AS:** So that's when you first became aware of Professor Card’s work. Professor Card, when did you first become aware of Professor Imbens’s work?\n\n**Card:** One of his early papers was pretty interesting. He was trying to combine data from micro survey evidence with benchmark numbers that you would get from a population, and it's actually a version of a kind of a problem that arises at Amazon all the time, which is, we've got noisy estimates of something, and we've got probably reliable estimates of some other aggregates, and there's often ways to try and combine those. I saw that and I thought that was very interesting.\n\nThen there’s the problem that Josh and Guido worked on that was most impactful and that was cited by the Nobel Prize committee. I had worked on an experiment, a real experiment [as opposed to a natural experiment], in welfare analysis in Canada, and it was providing an economic incentive to try and get single mothers off of welfare and into work. And we noticed that the group of mothers who complied or followed on with the experiment was reasonable size, but it wasn't 100%.\n\nWe did some analysis of it trying to characterize them. Around the same time, I became aware of [Imbens’s and Angrist’s pape](https://www.nber.org/system/files/working_papers/t0118/t0118.pdfr), which basically formalized that a lot better and described what exactly was going on with this group. That framework just instantly took off, and everyone within a few years was thinking about problems that way.\n\nThis morning I was talking to another Amazon person about a problem. It was a difference analysis. I was saying we should try and characterize the compliers for this difference intervention. So it's exactly this problem.\n\nThe Nobel committee’s [press release](https://www.nobelprize.org/prizes/economic-sciences/2021/press-release/) for Card, Imbens, and Angrist’s prize announcement emphasizes their use of natural experiments, which it defines as “situations in which chance events or policy changes result in groups of people being treated differently, in a way that resembles clinical trials in medicine.” A seminal instance of this was [Card’s 1993 paper with his Princeton colleague Alan Krueger](https://davidcard.berkeley.edu/papers/njmin-aer.pdf), which compared fast-food restaurants in two demographically similar communities on either side of the New Jersey-Pennsylvania border, one of which had recently seen a minimum-wage hike and one of which hadn’t.\n\n**AS:** In the early days, there was skepticism about the empirical approach to economics. So every time you selected a new research project, you weren't just trying to answer an economics problem; you were also, in a sense, establishing the credibility of the approach. How did you select problems then? Was there a structure that you recognized as possibly lending itself to natural experiment?\n\n**Card:** I think that the natural-experiment thing — there was really a brief period where that was novel, to tell you the truth. Maybe 1989 to 1992 or 3. I did this paper on the [Mariel boatlift](https://davidcard.berkeley.edu/papers/mariel-impact.pdf), which was cited by the committee. But to tell you the truth, that was a very modest paper. I never presented it anywhere, and it's in a very modest journal. So I never thought of that paper as going anywhere [laughs].\n\nWhat happened was, it became more and more well understood that in order to make a claim of causality even from a natural-experiment setting, you had to have a fair amount of information from before the experiment took place to validate or verify that the group that you were calling the treatment group and the group that you were calling the control group actually were behaving the same.\n\nThat was a weakness of the project that Alan Krueger and I did. We had restaurants in New Jersey and Pennsylvania. We knew the minimum wage was going to increase — or we thought we knew that; it wasn't entirely clear at the time — but we surveyed the restaurants before, and then the minimum wage went up, and we surveyed them after, and that was good.\n\nBut we didn't really have multiple surveys from before to show that in the absence of the minimum wage, New Jersey and Pennsylvania restaurants had tracked each other for a long time. And these days, that's better understood. At Amazon for instance, people are doing intervention analyses of this type. They would normally look at what they call pre-trend analysis, make sure that the treatment group and the control group are trending the same beforehand.\n\nI think there are 1,000 questions in economics that have been open forever. Sometimes new datasets come along. That's been happening a lot in labor economics: huge administrative datasets have become available, richer and richer, and now we're getting datasets that are created by these tech firms. So my usual thing is, I think, that's a dataset that maybe we can answer this old question on. That’s more my approach.\n\n#### That's why being at Amazon has been great .... A lot of people have substantive questions they're trying to analyze with data, and they're kind of stuck in places, so there's a need for new methodologies.\n\nGuido Imbens\n\n**Imbens:** I come from a slightly different perspective. Most of my work has come from listening to people like David and Josh and seeing what type of problems they're working on, what type of methods they're using, and seeing if there's something to be added there — if there’s some way of improving the methods or places where maybe they're stuck, but listening to the people actually doing the empirical work rather than starting with the substantive questions.\n\nThat's why being at Amazon has been great, from my perspective. A lot of people have substantive questions they're trying to analyze with data, and they're kind of stuck in places, so there's a need for new methodologies. It's been a very fertile environment for me to come up with new research.\n\n**AS:** Methodologically, what are some of the outstanding questions that interest you both?\n\n**Imbens:** Well, one of the things is experimental design in complex environments. A lot of the experimental designs we’re using at the moment still come fairly directly from biomedical settings. We have a population, we randomize them into a treatment group and a control group, and then we compare outcomes for the two groups.\n\nBut in a lot of the settings we’re interested in at Amazon, there are very complex interactions between the units and their experiences, and dealing with that is very challenging. There are lots of special cases where we know somewhat what to do, but there are lots of cases where we don't know exactly what to do, and we need to do more complex experiments to get the answers to the questions we're interested in.\n\n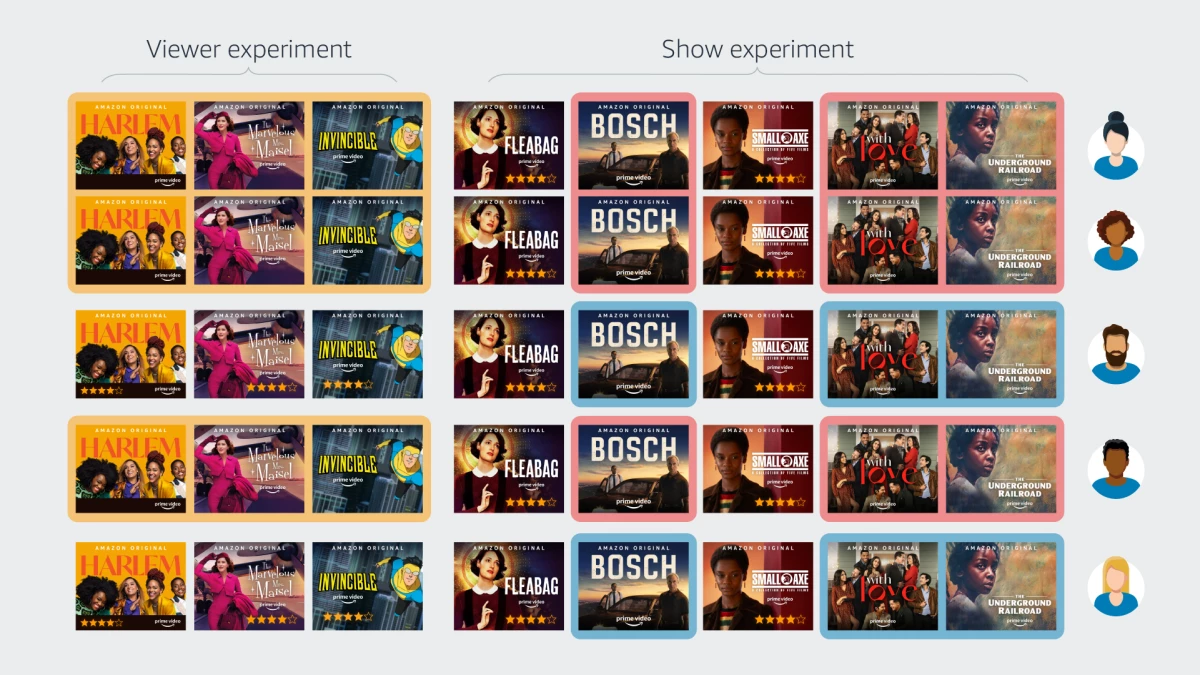\n\nAn example of what Imbens calls “experimental design in complex environments”. In this illustration, each of five viewers is shown promotions for eight different Prime Video shows. Some of those promotions contain extra information, indicated in the image by star ratings (the “treatment”). This design helps determine whether the treatment affects viewing habits (the viewer experiment) but also helps identify spillover effects, in which participation in the viewer experiment influences the viewer’s behavior in other contexts.\n\nThe second thing is, we do a lot of these experiments, but often the experiments are relatively small. They’re small in duration, and they’re small in size relative to the overall population. You know, it goes back to the paper we mentioned before, combining this observational-study data with experimental data. That raises a lot of interesting methodological challenges that I spend a lot of time thinking about these days.\n\n**AS:** I wondered if in the same way that in that early paper you were looking at survey data and population data, there's a way that natural experiments and economic field experiments can reinforce each other or give you a more reliable signal than you can get from either alone.\n\n**Card:** There's one thing that people do; I've done a few of these myself. It's called meta analysis. It's a technique where you take results from different studies and try and put them into a statistical model. In a way it's comparable to work Guido has done at Amazon, where you take a series of actual experiments, A/B experiments done in [Weblab](https://www.amazon.science/the-history-of-amazons-recommendation-algorithm), and basically combine them and say, “Okay, these aren't exactly the same products and the same conditions, but there's enough comparability that maybe I can build a model and use the information from the whole set to help inform what we're learning from any given one.”\n\nAnd you can do that in studies in economics. For example, I’ve done one on training programs. There are many of these training programs. Each of them — exactly as Guido was saying — is often quite small. And there are weird conditions: sometimes it's only young males or young females that are in the experiment, or they don't have very long follow-up, or sometimes the labor market is really strong, and other times it's really weak. So you can try and build a model of the outcome you get from any given study and then try and see if there are any systematic patterns there.\n\n**Imbens:** We do all these experiments, but often we kind of do them once, and then we put them aside. There's a lot of information over the years built up in all these experiments we've done, and finding more of these meta-analysis-type ways of combining them and exploiting all the information we have collected there — I think it's a very promising way to go.\n\n**AS:** How can empirical methods complement theoretical approaches — model building of the kind that, in some sense, the early empirical research was reacting against?\n\n**Card:** Normally, if you're building a model, there are a few key parameters, like you need to get some kind of an elasticity of what a customer will do if faced with a higher price or if offered a shorter, faster delivery speed versus slower delivery speed. And if you have those elasticities, then you can start building up a model.\n\nIf you have even a fairly complicated dynamic model, normally there's a relatively small number of these parameters, and the value of the model is to take this set of parameters and try and tell a bit richer story — not just how the customer responds to an offer of a faster delivery today but how that affects their future purchases and whether they come back and buy other products or whatever. But you need credible estimates of those elasticities. It's not helpful to build a model and then just pull numbers out of the air [laughs]. And that's why A/B experiments are so important at Amazon.\n\nAS: I asked about outstanding methodological questions that you're interested in, but how about economic questions more broadly that you think could really benefit from an empirical approach?\n\nCard: In my field [labor economics], we've begun to realize that different firms are setting different wages for the same kinds of workers. And we're starting to think about two issues related to that. One is, how do workers choose between jobs? Do they know about all the jobs out there? Do they just find out about some of the jobs? We're trying to figure out exactly why it's okay in the labor market for there to be multiple wages for a certain class of workers. Why don't all the workers immediately try to go to one job? This seems to be a very important phenomenon.\n\nAnd on the other side of that, how do employers think about it? What are the benefits to employers of a higher wage or lower wage? Is it just the recruiting, or is it retention, or is it productivity? Is it longer-term goals? That's front and center in the research that I do outside of Amazon. I asked about outstanding methodological questions that you're interested in, but how about economic questions more broadly that you think could really benefit from an empirical approach?\n\n**Card:** In my field [labor economics], we've begun to realize that different firms are setting different wages for the same kinds of workers. And we're starting to think about two issues related to that. One is, how do workers choose between jobs? Do they know about all the jobs out there? Do they just find out about some of the jobs? We're trying to figure out exactly why it's okay in the labor market for there to be multiple wages for a certain class of workers. Why don't all the workers immediately try to go to one job? This seems to be a very important phenomenon.\n\nAnd on the other side of that, how do employers think about it? What are the benefits to employers of a higher wage or lower wage? Is it just the recruiting, or is it retention, or is it productivity? Is it longer-term goals? That's front and center in the research that I do outside of Amazon.\n\n**AS:** I asked about outstanding methodological questions that you're interested in, but how about economic questions more broadly that you think could really benefit from an empirical approach?\n\n**Card:** In my field [labor economics], we've begun to realize that different firms are setting different wages for the same kinds of workers. And we're starting to think about two issues related to that. One is, how do workers choose between jobs? Do they know about all the jobs out there? Do they just find out about some of the jobs? We're trying to figure out exactly why it's okay in the labor market for there to be multiple wages for a certain class of workers. Why don't all the workers immediately try to go to one job? This seems to be a very important phenomenon.\n\nAnd on the other side of that, how do employers think about it? What are the benefits to employers of a higher wage or lower wage? Is it just the recruiting, or is it retention, or is it productivity? Is it longer-term goals? That's front and center in the research that I do outside of Amazon.\n\n**AS:** I was curious if there were any cases where a problem presented itself, and at first you didn't think there was any way to get an empirical handle on it, and then you figured out that there was.\n\n**Card:** I saw a really interesting paper that was done by a PhD student who was visiting my center at Berkeley. In European football, there are a lot of non-white players, and fan racism is pretty pervasive. This guy noticed that during COVID, they played a lot of games with no fans. So he was able to compare the performance of the non-white and white players in the pre-COVID era and the COVID era, with and without fans, and showed that the non-white players did a little bit better. That's the kind of question where you’re saying, How are we ever going to study that? But if you're thinking and looking around, there's always some angle that might be useful.\n\n**Imbens:** That's a very clever idea. I agree with David. If you just pay attention, there are a lot of things happening that allow you to answer important questions. Maybe fan insults in sports itself isn't that big a deal, but clearly, racism in the labor market and having people treated differently is a big problem. And here you get a very clear handle on an aspect of it. And once you show it's a problem there, it's very likely that it shows up in arguably substantively much more important settings where it's really hard to study.\n\n#### We're supposed to be social scientists who are trying to see what people are doing and the problems they confront and trying to analyze them. ... That's different than this old-fashioned Adam Smith view of the economy as a perfectly functioning tool that we're just supposed to admire.\n\nDavid Card\n\nIn the Netherlands for a long time, they had a limit on the number of students who could go to medical school. And it wasn't decided by the medical schools themselves; they couldn't choose whom to admit. It was partly based on a lottery. At some point, someone used that to figure out how much access to medical school is actually worth. So essentially, you have two people who are both qualified to go to medical school; one gets lucky in the lottery; one doesn't. And it turns out you're giving the person who wins the lottery basically a lot of money. Obviously, in many professions we can't just randomly assign people to different types of jobs. But here you get a handle on the value of rationing that type of education.\n\n**Card:** I think that's really important. You know, we're supposed to be social scientists who are trying to see what people are doing and the problems they confront and trying to analyze them. In a way, that's different than this sort of old-fashioned Adam Smith view of the economy as a perfectly functioning tool that we're just supposed to admire. That is a difference, I think.\n\nABOUT THE AUTHOR\n\n[**Larry Hardesty**](https://www.amazon.science/author/larry-hardesty)\n\nLarry Hardesty is the editor of the Amazon Science blog. Previously, he was a senior editor at MIT Technology Review and the computer science writer at the MIT News Office.","render":"<p><img src=\\"https://dev-media.amazoncloud.cn/e3223461adcc42e1bfb2bde356aacbf6_image.png\\" alt=\\"image.png\\" /></p>\n<p>David Card (left), an Amazon Scholar, a professor of economics at the University of California, Berkeley, and the outgoing president of the AEA, and Guido Imbens (right), an academic research consultant at Amazon and a professor at the Stanford Graduate School of Business.</p>\n<p>The annual meeting of the American Economic Association (AEA) took place Jan. 7 - 9, and as it approached, Amazon Science had the chance to interview two of the three recipients of the 2021 Nobel Prize in economics — who also happen to be Amazon-affiliated economists.</p>\n<p>David Card, an Amazon Scholar, a professor of economics at the University of California, Berkeley, and the outgoing president of the AEA, won half the prize “for his empirical contributions to labor economics”.</p>\n<p>Guido Imbens, an academic research consultant at Amazon and a professor at the Stanford Graduate School of Business, shared the other half of the prize with MIT’s <a href=\\"https://news.mit.edu/2021/mit-economist-joshua-angrist-shares-nobel-prize-1011\\" target=\\"_blank\\">Josh Angrist</a> for “methodological contributions to the analysis of causal relationships”.</p>\\n<p><strong>Amazon Science:</strong> The empirical approach to economics has been recognized by the Nobel Prize committee several times in the last few years, but it wasn’t always as popular as it is today. I’m curious how you both first became interested in empirical approaches to economics.</p>\\n<p><strong>David Card:</strong> The heroes of economics for many, many decades were the theorists, and in the postwar era especially, there was a recognition that economic modeling was underdeveloped — the math was underdeveloped — and there was a need to formalize things and understand better what the models really delivered.</p>\\n<p>That need really proceeded through the ’60s, and Arrow and Debreu were these famous mathematical economists who developed some very elegant theoretical models of how the market works in an idealized economy.</p>\n<p>What happened in my time was people started to realize that we had the data to better look at real labor market phenomena and possibly make economics something different than just a kind of a branch of philosophy. <a href=\\"https://en.wikipedia.org/wiki/Arrow%E2%80%93Debreu_model\\" target=\\"_blank\\">Arrow-Debreu </a>is basically mathematical philosophy.</p>\\n<p><strong>Guido Imbens:</strong> I came from a very different tradition. I grew up in the Netherlands, and there was a strong tradition of econometrics started by people like Tinbergen. <a href=\\"https://en.wikipedia.org/wiki/Jan_Tinbergen\\" target=\\"_blank\\">Tinbergen</a> had been very broad — he did econometrics, but he also did empirical work and was very heavily involved in policy analysis. But over time, the program he had started was becoming much more focused on technical econometrics.</p>\\n<h4><a id=\\"People_started_to_realize_that_we_had_the_data_to_better_look_at_real_labor_market_phenomena_and_possibly_make_economics_something_different_than_just_a_kind_of_a_branch_of_philosophy_20\\"></a>People started to realize that we had the data to better look at real labor market phenomena and possibly make economics something different than just a kind of a branch of philosophy.</h4>\\n<p>David Card</p>\n<p>So as an undergraduate, we didn’t really do any empirical work. We really just did a lot of mathematical statistics and some operations research and some economic theory. My thesis was a theoretical econometrics study.</p>\n<p>When I presented that at Harvard, Josh Angrist wasn’t really all that impressed with it, and he actually opposed the department hiring me there because he thought the paper was boring. And he was probably right! But luckily, the more senior people there at the time thought I was at least somewhat promising. And so I got hired at Harvard. But then it was really Josh and <a href=\\"https://scholar.harvard.edu/lkatz/home\\" target=\\"_blank\\">Larry Katz</a>, one of the labor economists there, who got me interested in going to the labor seminar and got me exposed to the modern empirical work.</p>\\n<p>The context Josh and I started talking in really was this paper that I think came up in all three of the Nobel lectures, this paper by Ed Leamer, “<a href=\\"https://www.jstor.org/stable/1803924?seq=1\\" target=\\"_blank\\">Let’s Take the Con Out of Econometrics</a>”, where Leamer says, “Hardly anyone takes data analysis seriously. Or perhaps more accurately, hardly anyone takes anyone else’s data analysis seriously.”</p>\\n<p>And I think Leamer was right: people did these very elaborate things, and it was all showing off complicated technical things, but it wasn’t really very credible. In fact, Leamer presented a lecture based on that work at Harvard. And I remember Josh getting up at some point and saying, “Well, you talk about all this old stuff, but look at the work Card does. Look at the work Krueger does. Look at the work I do. It’s very different.”</p>\n<p>And that felt right to me. It felt that the work was qualitatively very different from the work that Ed Leamer was describing and that he was complaining about.</p>\n<p><strong>AS:</strong> So that’s when you first became aware of Professor Card’s work. Professor Card, when did you first become aware of Professor Imbens’s work?</p>\\n<p><strong>Card:</strong> One of his early papers was pretty interesting. He was trying to combine data from micro survey evidence with benchmark numbers that you would get from a population, and it’s actually a version of a kind of a problem that arises at Amazon all the time, which is, we’ve got noisy estimates of something, and we’ve got probably reliable estimates of some other aggregates, and there’s often ways to try and combine those. I saw that and I thought that was very interesting.</p>\\n<p>Then there’s the problem that Josh and Guido worked on that was most impactful and that was cited by the Nobel Prize committee. I had worked on an experiment, a real experiment [as opposed to a natural experiment], in welfare analysis in Canada, and it was providing an economic incentive to try and get single mothers off of welfare and into work. And we noticed that the group of mothers who complied or followed on with the experiment was reasonable size, but it wasn’t 100%.</p>\n<p>We did some analysis of it trying to characterize them. Around the same time, I became aware of <a href=\\"https://www.nber.org/system/files/working_papers/t0118/t0118.pdfr\\" target=\\"_blank\\">Imbens’s and Angrist’s pape</a>, which basically formalized that a lot better and described what exactly was going on with this group. That framework just instantly took off, and everyone within a few years was thinking about problems that way.</p>\\n<p>This morning I was talking to another Amazon person about a problem. It was a difference analysis. I was saying we should try and characterize the compliers for this difference intervention. So it’s exactly this problem.</p>\n<p>The Nobel committee’s <a href=\\"https://www.nobelprize.org/prizes/economic-sciences/2021/press-release/\\" target=\\"_blank\\">press release</a> for Card, Imbens, and Angrist’s prize announcement emphasizes their use of natural experiments, which it defines as “situations in which chance events or policy changes result in groups of people being treated differently, in a way that resembles clinical trials in medicine.” A seminal instance of this was <a href=\\"https://davidcard.berkeley.edu/papers/njmin-aer.pdf\\" target=\\"_blank\\">Card’s 1993 paper with his Princeton colleague Alan Krueger</a>, which compared fast-food restaurants in two demographically similar communities on either side of the New Jersey-Pennsylvania border, one of which had recently seen a minimum-wage hike and one of which hadn’t.</p>\\n<p><strong>AS:</strong> In the early days, there was skepticism about the empirical approach to economics. So every time you selected a new research project, you weren’t just trying to answer an economics problem; you were also, in a sense, establishing the credibility of the approach. How did you select problems then? Was there a structure that you recognized as possibly lending itself to natural experiment?</p>\\n<p><strong>Card:</strong> I think that the natural-experiment thing — there was really a brief period where that was novel, to tell you the truth. Maybe 1989 to 1992 or 3. I did this paper on the <a href=\\"https://davidcard.berkeley.edu/papers/mariel-impact.pdf\\" target=\\"_blank\\">Mariel boatlift</a>, which was cited by the committee. But to tell you the truth, that was a very modest paper. I never presented it anywhere, and it’s in a very modest journal. So I never thought of that paper as going anywhere [laughs].</p>\\n<p>What happened was, it became more and more well understood that in order to make a claim of causality even from a natural-experiment setting, you had to have a fair amount of information from before the experiment took place to validate or verify that the group that you were calling the treatment group and the group that you were calling the control group actually were behaving the same.</p>\n<p>That was a weakness of the project that Alan Krueger and I did. We had restaurants in New Jersey and Pennsylvania. We knew the minimum wage was going to increase — or we thought we knew that; it wasn’t entirely clear at the time — but we surveyed the restaurants before, and then the minimum wage went up, and we surveyed them after, and that was good.</p>\n<p>But we didn’t really have multiple surveys from before to show that in the absence of the minimum wage, New Jersey and Pennsylvania restaurants had tracked each other for a long time. And these days, that’s better understood. At Amazon for instance, people are doing intervention analyses of this type. They would normally look at what they call pre-trend analysis, make sure that the treatment group and the control group are trending the same beforehand.</p>\n<p>I think there are 1,000 questions in economics that have been open forever. Sometimes new datasets come along. That’s been happening a lot in labor economics: huge administrative datasets have become available, richer and richer, and now we’re getting datasets that are created by these tech firms. So my usual thing is, I think, that’s a dataset that maybe we can answer this old question on. That’s more my approach.</p>\n<h4><a id=\\"Thats_why_being_at_Amazon_has_been_great__A_lot_of_people_have_substantive_questions_theyre_trying_to_analyze_with_data_and_theyre_kind_of_stuck_in_places_so_theres_a_need_for_new_methodologies_58\\"></a>That’s why being at Amazon has been great … A lot of people have substantive questions they’re trying to analyze with data, and they’re kind of stuck in places, so there’s a need for new methodologies.</h4>\\n<p>Guido Imbens</p>\n<p><strong>Imbens:</strong> I come from a slightly different perspective. Most of my work has come from listening to people like David and Josh and seeing what type of problems they’re working on, what type of methods they’re using, and seeing if there’s something to be added there — if there’s some way of improving the methods or places where maybe they’re stuck, but listening to the people actually doing the empirical work rather than starting with the substantive questions.</p>\\n<p>That’s why being at Amazon has been great, from my perspective. A lot of people have substantive questions they’re trying to analyze with data, and they’re kind of stuck in places, so there’s a need for new methodologies. It’s been a very fertile environment for me to come up with new research.</p>\n<p><strong>AS:</strong> Methodologically, what are some of the outstanding questions that interest you both?</p>\\n<p><strong>Imbens:</strong> Well, one of the things is experimental design in complex environments. A lot of the experimental designs we’re using at the moment still come fairly directly from biomedical settings. We have a population, we randomize them into a treatment group and a control group, and then we compare outcomes for the two groups.</p>\\n<p>But in a lot of the settings we’re interested in at Amazon, there are very complex interactions between the units and their experiences, and dealing with that is very challenging. There are lots of special cases where we know somewhat what to do, but there are lots of cases where we don’t know exactly what to do, and we need to do more complex experiments to get the answers to the questions we’re interested in.</p>\n<p><img src=\\"https://dev-media.amazoncloud.cn/c5ed315884694c15afc990a3fd6f1877_image.png\\" alt=\\"image.png\\" /></p>\n<p>An example of what Imbens calls “experimental design in complex environments”. In this illustration, each of five viewers is shown promotions for eight different Prime Video shows. Some of those promotions contain extra information, indicated in the image by star ratings (the “treatment”). This design helps determine whether the treatment affects viewing habits (the viewer experiment) but also helps identify spillover effects, in which participation in the viewer experiment influences the viewer’s behavior in other contexts.</p>\n<p>The second thing is, we do a lot of these experiments, but often the experiments are relatively small. They’re small in duration, and they’re small in size relative to the overall population. You know, it goes back to the paper we mentioned before, combining this observational-study data with experimental data. That raises a lot of interesting methodological challenges that I spend a lot of time thinking about these days.</p>\n<p><strong>AS:</strong> I wondered if in the same way that in that early paper you were looking at survey data and population data, there’s a way that natural experiments and economic field experiments can reinforce each other or give you a more reliable signal than you can get from either alone.</p>\\n<p><strong>Card:</strong> There’s one thing that people do; I’ve done a few of these myself. It’s called meta analysis. It’s a technique where you take results from different studies and try and put them into a statistical model. In a way it’s comparable to work Guido has done at Amazon, where you take a series of actual experiments, A/B experiments done in <a href=\\"https://www.amazon.science/the-history-of-amazons-recommendation-algorithm\\" target=\\"_blank\\">Weblab</a>, and basically combine them and say, “Okay, these aren’t exactly the same products and the same conditions, but there’s enough comparability that maybe I can build a model and use the information from the whole set to help inform what we’re learning from any given one.”</p>\\n<p>And you can do that in studies in economics. For example, I’ve done one on training programs. There are many of these training programs. Each of them — exactly as Guido was saying — is often quite small. And there are weird conditions: sometimes it’s only young males or young females that are in the experiment, or they don’t have very long follow-up, or sometimes the labor market is really strong, and other times it’s really weak. So you can try and build a model of the outcome you get from any given study and then try and see if there are any systematic patterns there.</p>\n<p><strong>Imbens:</strong> We do all these experiments, but often we kind of do them once, and then we put them aside. There’s a lot of information over the years built up in all these experiments we’ve done, and finding more of these meta-analysis-type ways of combining them and exploiting all the information we have collected there — I think it’s a very promising way to go.</p>\\n<p><strong>AS:</strong> How can empirical methods complement theoretical approaches — model building of the kind that, in some sense, the early empirical research was reacting against?</p>\\n<p><strong>Card:</strong> Normally, if you’re building a model, there are a few key parameters, like you need to get some kind of an elasticity of what a customer will do if faced with a higher price or if offered a shorter, faster delivery speed versus slower delivery speed. And if you have those elasticities, then you can start building up a model.</p>\\n<p>If you have even a fairly complicated dynamic model, normally there’s a relatively small number of these parameters, and the value of the model is to take this set of parameters and try and tell a bit richer story — not just how the customer responds to an offer of a faster delivery today but how that affects their future purchases and whether they come back and buy other products or whatever. But you need credible estimates of those elasticities. It’s not helpful to build a model and then just pull numbers out of the air [laughs]. And that’s why A/B experiments are so important at Amazon.</p>\n<p>AS: I asked about outstanding methodological questions that you’re interested in, but how about economic questions more broadly that you think could really benefit from an empirical approach?</p>\n<p>Card: In my field [labor economics], we’ve begun to realize that different firms are setting different wages for the same kinds of workers. And we’re starting to think about two issues related to that. One is, how do workers choose between jobs? Do they know about all the jobs out there? Do they just find out about some of the jobs? We’re trying to figure out exactly why it’s okay in the labor market for there to be multiple wages for a certain class of workers. Why don’t all the workers immediately try to go to one job? This seems to be a very important phenomenon.</p>\n<p>And on the other side of that, how do employers think about it? What are the benefits to employers of a higher wage or lower wage? Is it just the recruiting, or is it retention, or is it productivity? Is it longer-term goals? That’s front and center in the research that I do outside of Amazon. I asked about outstanding methodological questions that you’re interested in, but how about economic questions more broadly that you think could really benefit from an empirical approach?</p>\n<p><strong>Card:</strong> In my field [labor economics], we’ve begun to realize that different firms are setting different wages for the same kinds of workers. And we’re starting to think about two issues related to that. One is, how do workers choose between jobs? Do they know about all the jobs out there? Do they just find out about some of the jobs? We’re trying to figure out exactly why it’s okay in the labor market for there to be multiple wages for a certain class of workers. Why don’t all the workers immediately try to go to one job? This seems to be a very important phenomenon.</p>\\n<p>And on the other side of that, how do employers think about it? What are the benefits to employers of a higher wage or lower wage? Is it just the recruiting, or is it retention, or is it productivity? Is it longer-term goals? That’s front and center in the research that I do outside of Amazon.</p>\n<p><strong>AS:</strong> I asked about outstanding methodological questions that you’re interested in, but how about economic questions more broadly that you think could really benefit from an empirical approach?</p>\\n<p><strong>Card:</strong> In my field [labor economics], we’ve begun to realize that different firms are setting different wages for the same kinds of workers. And we’re starting to think about two issues related to that. One is, how do workers choose between jobs? Do they know about all the jobs out there? Do they just find out about some of the jobs? We’re trying to figure out exactly why it’s okay in the labor market for there to be multiple wages for a certain class of workers. Why don’t all the workers immediately try to go to one job? This seems to be a very important phenomenon.</p>\\n<p>And on the other side of that, how do employers think about it? What are the benefits to employers of a higher wage or lower wage? Is it just the recruiting, or is it retention, or is it productivity? Is it longer-term goals? That’s front and center in the research that I do outside of Amazon.</p>\n<p><strong>AS:</strong> I was curious if there were any cases where a problem presented itself, and at first you didn’t think there was any way to get an empirical handle on it, and then you figured out that there was.</p>\\n<p><strong>Card:</strong> I saw a really interesting paper that was done by a PhD student who was visiting my center at Berkeley. In European football, there are a lot of non-white players, and fan racism is pretty pervasive. This guy noticed that during COVID, they played a lot of games with no fans. So he was able to compare the performance of the non-white and white players in the pre-COVID era and the COVID era, with and without fans, and showed that the non-white players did a little bit better. That’s the kind of question where you’re saying, How are we ever going to study that? But if you’re thinking and looking around, there’s always some angle that might be useful.</p>\\n<p><strong>Imbens:</strong> That’s a very clever idea. I agree with David. If you just pay attention, there are a lot of things happening that allow you to answer important questions. Maybe fan insults in sports itself isn’t that big a deal, but clearly, racism in the labor market and having people treated differently is a big problem. And here you get a very clear handle on an aspect of it. And once you show it’s a problem there, it’s very likely that it shows up in arguably substantively much more important settings where it’s really hard to study.</p>\\n<h4><a id=\\"Were_supposed_to_be_social_scientists_who_are_trying_to_see_what_people_are_doing_and_the_problems_they_confront_and_trying_to_analyze_them__Thats_different_than_this_oldfashioned_Adam_Smith_view_of_the_economy_as_a_perfectly_functioning_tool_that_were_just_supposed_to_admire_114\\"></a>We’re supposed to be social scientists who are trying to see what people are doing and the problems they confront and trying to analyze them. … That’s different than this old-fashioned Adam Smith view of the economy as a perfectly functioning tool that we’re just supposed to admire.</h4>\\n<p>David Card</p>\n<p>In the Netherlands for a long time, they had a limit on the number of students who could go to medical school. And it wasn’t decided by the medical schools themselves; they couldn’t choose whom to admit. It was partly based on a lottery. At some point, someone used that to figure out how much access to medical school is actually worth. So essentially, you have two people who are both qualified to go to medical school; one gets lucky in the lottery; one doesn’t. And it turns out you’re giving the person who wins the lottery basically a lot of money. Obviously, in many professions we can’t just randomly assign people to different types of jobs. But here you get a handle on the value of rationing that type of education.</p>\n<p><strong>Card:</strong> I think that’s really important. You know, we’re supposed to be social scientists who are trying to see what people are doing and the problems they confront and trying to analyze them. In a way, that’s different than this sort of old-fashioned Adam Smith view of the economy as a perfectly functioning tool that we’re just supposed to admire. That is a difference, I think.</p>\\n<p>ABOUT THE AUTHOR</p>\n<p><a href=\\"https://www.amazon.science/author/larry-hardesty\\" target=\\"_blank\\"><strong>Larry Hardesty</strong></a></p>\n<p>Larry Hardesty is the editor of the Amazon Science blog. Previously, he was a senior editor at MIT Technology Review and the computer science writer at the MIT News Office.</p>\n"}