{"value":"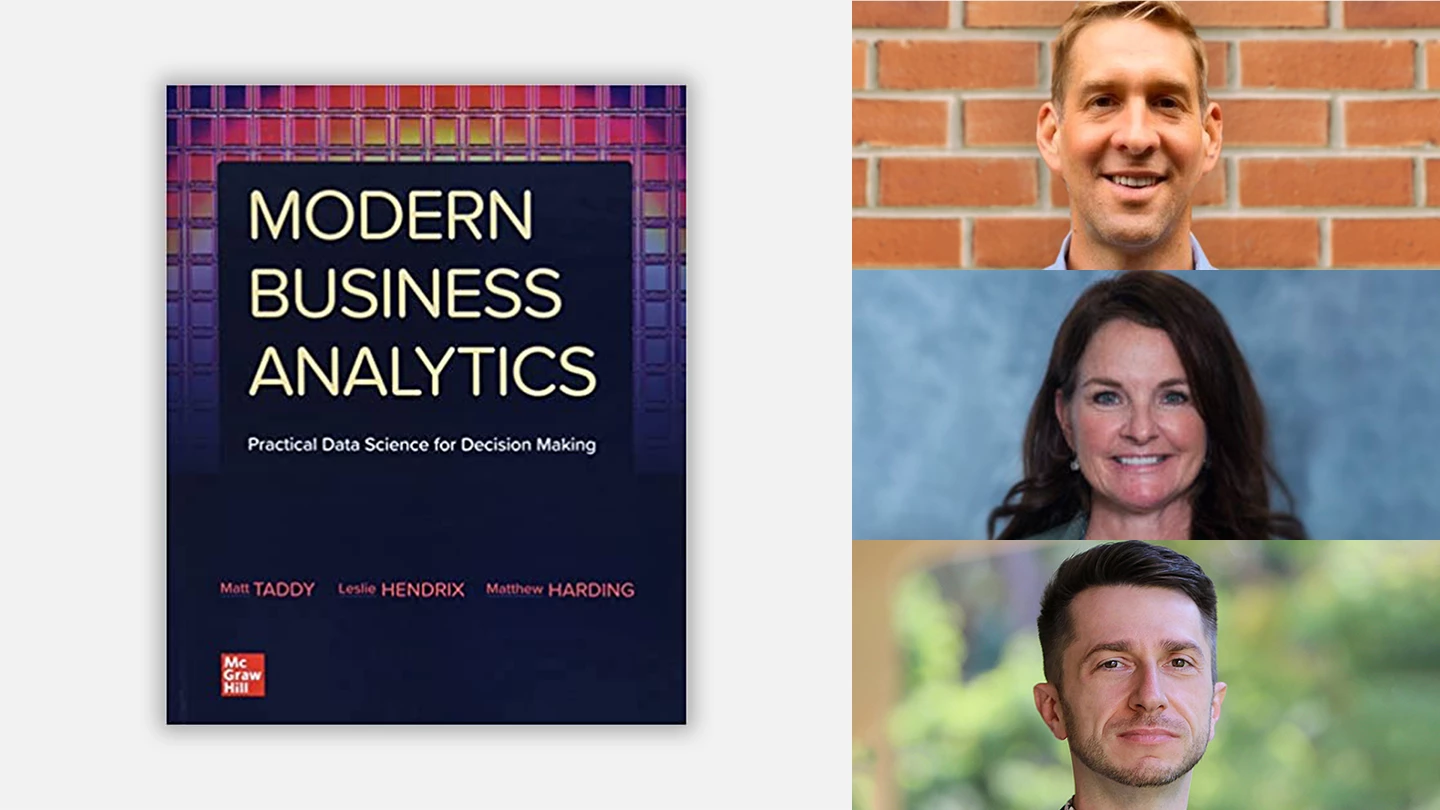\n\nMatt Taddy (top right), vice president of Amazon's Private Brands business, is the coauthor of a recently released data-science textbook, Modern Business Analytics, along with Leslie Hendrix (middle right), and Matthew C. Harding.\n\nMatt Taddy, vice president of Amazon’s Private Brands business, is the coauthor of [Modern Business Analytics](https://www.amazon.com/Modern-Business-Analytics-Matt-Taddy/dp/1264071671/ref=sr_1_1?crid=3M38RU1Z95VYO&keywords=Modern+Business+Analytics&qid=1651095743&sprefix=modern+business+analytics%2Caps%2C196&sr=8-1): Practical Data Science for Decision Making, a primer for those who want to gain the skills to use data science to help make decisions in business and beyond.\n\nWhen Matt Taddy earned his PhD in applied mathematics and statistics from the University of California, Santa Cruz, in 2008, the notion of a data-science specialization was still in its infancy.\n\nToday, the business-analytics profession, or the discipline of using data to make business, public policy, public health, and other decisions, is blossoming, and Taddy is excited about how the field is becoming more multi-disciplinary, incorporating statistics, machine learning, economics, and even the social sciences.\n\n“I benefitted from getting involved in the early stages before it became more specialized,” says Taddy.\n\nSince earning his PhD, Taddy has been a research assistant at NASA Ames Research Center and Sandia National Laboratories, a research fellow at eBay, the head of economics and data science for Business AI at Microsoft, a professor of econometrics and statistics at the University of Chicago Booth School of Business, the chief economist for Amazon’s North America Consumer organization, and now vice president of Amazon’s [Private Brands](https://jobs-us-east.amazon.com/en/teams/amazon-private-brands) business. His first textbook, [Business Data Science](https://www.amazon.com/Business-Data-Science-Combining-Accelerate/dp/1260452778), was published by McGraw Hill in 2019. At the time, he told [Amazon Science](https://www.amazon.science/business-data-science-is-a-lot-more-than-just-making-predictions-matt-taddy) that he began work on the book ten years prior when teaching a class of MBA students at the University of Chicago.\n\n“I realized that there was an appetite for the material covered in the book from people who weren’t specialists in statistics or machine learning,” he said. “This idea that we could teach this material to non-specialists really motivated me not to just write this book, but also to push for changing the curriculum at the University of Chicago.”\n\nSince publishing that textbook in 2019, his role at Amazon has evolved as has his interest in making great decisions from data. The result is a new textbook, [Modern Business Analytics: Practical Data Science for Decision Making](https://www.amazon.com/Modern-Business-Analytics-Matt-Taddy/dp/1264071671/ref=sr_1_1?crid=3M38RU1Z95VYO&keywords=Modern+Business+Analytics&qid=1651095743&sprefix=modern+business+analytics%2Caps%2C196&sr=8-1), which Taddy co-authored with [Leslie Hendrix](https://sc.edu/study/colleges_schools/moore/directory/hendrix.leslie.php), PhD, associate professor at the Darla Moore School of Business at the University of South Carolina, and [Matthew C. Harding](https://www.socsci.uci.edu/~harding1/), PhD, professor of economics and statistics at the University of California, Irvine.\n\n<video src=\\"https://dev-media.amazoncloud.cn/3d8e7336321b427b91588ab4642b1155_Matt%20Taddy%20-%20Modern%20Business%20Analytics%20Author%20Video.mp4\\" class=\\"manvaVedio\\" controls=\\"controls\\" style=\\"width:160px;height:160px\\"></video>\n\nAccording to the authors, “This book is a primer for those who want to gain the skills to use data science to help make decisions in business and beyond. The modern business analyst uses tools from machine learning, economics, and statistics to not only track what has happened but predict the future for their businesses.”\n\nMcGraw Hill, the book’s publisher, says: “This new higher-ed text takes a practical, modern approach to data science and business analytics for the analytics student and professional. It gives students the opportunity to learn by doing, with real data analysis examples that explain the ‘why’, rather than the ‘what’ in decision-making discussions. It uses R as the primary technology through the text and includes an end-of-chapter reference to the basic R recipes in each chapter. Modern Business Analytics: Practical Data Science for Decision Making has crossed the boundaries and created something truly interdisciplinary.”\n\nAmazon Science connected with Taddy to discuss how his thinking about the topic has evolved in the past three years, his belief that deeper business decisions require focusing on why things happen versus what has happened, and how he’s applying modern business analytics techniques in running Amazon’s Private Brands business.\n\n#### **Q. In 2019 you authored Business Data Science that brought together concepts from statistics, machine learning, and the social sciences to help businesses use data more effectively. How has your thinking evolved in the past three years? And how does Modern Business Analytics address that?**\n\n**A.** Modern Business Analytics is a direct follow to Business Data Science. From Business Data Science we learned there is an audience, but I received feedback from a number of professors who, for example, were teaching from Business Data Science for MBA classes, or advanced undergraduate data-science classes, or master’s in public policy programs, that we didn’t really deliver the content in a format that was accessible to a broader audience.\nMcGraw Hill approached me again and said there was an opportunity to do a better job serving a wider audience and asked if I would be interested. My response was ‘Of course. One reason I did the book initially was to try and hit the widest audience possible.’ Recognizing that I’m busy with my day job here at Amazon, McGraw Hill suggested I approach co-authors to help with content development. Fortunately, both Leslie and Matthew agreed to contribute. Matthew teaches from this book in an MBA program at the University of California, Irvine, and Leslie teaches from a version of this book for a business-analytics program at the University of South Carolina.\n\nIt is finally here!!!! [#businessanalytics](https://twitter.com/hashtag/businessanalytics?src=hash&ref_src=twsrc%5Etfw) [@MattTaddy](https://twitter.com/MattTaddy?ref_src=twsrc%5Etfw) [#EconTwitter](https://twitter.com/hashtag/EconTwitter?src=hash&ref_src=twsrc%5Etfw) [#MachineLearning](https://twitter.com/hashtag/MachineLearning?src=hash&ref_src=twsrc%5Etfw) [pic.twitter.com/rT2IWJdwM5](https://t.co/rT2IWJdwM5)— Matthew Harding 🏳️🌈 (@BigDataMetrics) [April 15, 2022](https://twitter.com/BigDataMetrics/status/1515111807811158016?ref_src=twsrc%5Etfw)\n\nThey've both experienced the challenges of onboarding students who have no exposure to programming languages, or students who are less proficient in math than the students I was originally exposed to when I wrote the material for Business Data Science. Leslie and Matthew brought a great new perspective to the project. Generally, you're never happy with the first version of anything. Leslie and Matthew helped simplify some of the explanations provided in the previous book and contributed more examples. From my experience this is what students benefit from the most. The result: we were able to include many more real-world examples into Modern Business Analytics and make the new book far more accessible to a broader audience.\n\nIn education it often takes a while for someone to develop an introductory-level book that pulls material from multiple disciplines and brings readers to the current state of the art. That’s what we challenged ourselves to deliver here. Our audience is anyone who wants to get the skills to use modern large-scale data to make decisions, whether they are in business, government, science, or anywhere else.\n\n#### **Q. It would seem that today's modern business analyst must be multidisciplinary, with machine learning, economics, statistics, and other skills. What’s the skill set you look for?**\n\n**A.** I haven’t found an individual with all of those ingredients in equal measure. It is more about how you build a team with a diversity of skills and backgrounds. Data scientists, research scientists, applied scientists, and economists all use the tools that we discuss in the book. When you’re building a team focused on making decisions from data, you don’t want individuals with the same skills. You want individuals with different levels of emphasis. Some are going to have a much stronger background in computer science. They're going to understand the algorithms component better. Others are going to have a stronger background in uncertainty quantification and the mathematics of what I refer to as modern statistics. Some will have an economics background element. Others will be comfortable addressing causal inference and structural analysis.\nWhat’s been really exciting about data science in the past 10 years is that we've created a common vocabulary so individuals from many disciplines can talk to each other. Today, you can build a team that has economists, applied scientists, research scientists, machine learning engineers, and data scientists working together to address a common challenge. When I first got into data science more than a decade ago this common vocabulary didn’t exist. There was a real boundary to working on data across disciplines. Fortunately, much of this has gone away. Now the economists and the machine-learning practitioners speak the same lingo making it much easier to build the diverse teams required to make decisions from data.\n\n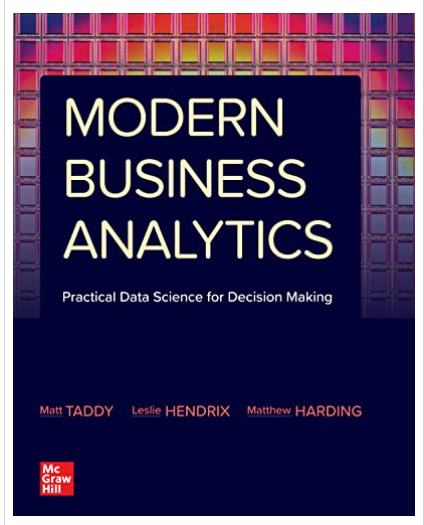\n\nI mentioned causal inference and structural analysis previously and want to point out another aspect of the book that is unique. A lot of work inside a tech company can focus on pure prediction, what I would consider standard machine learning problems where you want to discover patterns in correlation. For a broader audience beyond machine learners, we need to understand how to make policy decisions – how to use data to decide between option A or option B. For that type of decision-making you really have to get into the structure of why things are happening.\n\nI took that seriously in the first book and doubled down on it with this book. For example, we have a chapter that’s dedicated to either fully randomized experiments or quasi experimental settings. These are A/B experiments, or what we refer to at Amazon as Weblabs. If you’re familiar with these experiments you know they aren’t nearly as simple as the term A/B implies. There's a lot of complexity to these experiments — how you run them, how you analyze them. As a result, we focus a lot of attention on how to structure these A/B style trials and how you analyze data that has some experimental randomization as part of it.\n\nAnother chapter is devoted to understanding why things are happening when you’re working from purely observational data. Here we go deep into some of the methods we use heavily in industry — orthogonal or double machine learning using high dimensional control sets and other things such as synthetic controls. This chapter codifies the methods for utilizing causal analysis and structural analysis in observational settings. As mentioned previously, I think this differentiates the book from others for this audience. Causal inference can be intimidating and you don’t often see it addressed at this level. It will be a high bar for some students, but the feedback we’ve received from professors who are teaching from early versions of our work is that students, especially those with some industry experience, are really attracted to the material. The students are attracted to it because they have worked in industry and know how important it is to be able to properly conduct experiments and perform causal analyses.\n\n#### **Q. Can you provide some context about Amazon’s Private Brands business, and how you’re applying modern business analytics to make better decisions for your business.**\n\n**A.** I took over the role leading our worldwide Private Brands organization within the last year and much of what we do is seemingly simple, straightforward customer-centric product development. When I think about our business, I think about what product assortment we need in the long term. To determine this, I have to understand what products our customers expect from Amazon private brands and what they are able to get in private-label format from our competitors. Those are fairly straightforward business questions to address. Our customers expect us to have really attractive prices, so we need to ensure that our customers find sharp every-day low prices for the products that we're providing. And our customers expect quality. We need to make sure that they are getting the quality they expect and that our manufacturers are getting feedback from customers that allows them to produce better products.\n\nThat all sounds like pretty vanilla stuff. I could be talking about any number of MBA case studies and all of our competitor retailers are asking similar questions of their private label businesses.\n\n#### **\"I want us to build the ML services that allow us to quickly determine from customer feedback where there might be issues or opportunities anywhere in our production.\" **\n\nMatt Taddy\n\nBut then I think about how I can use data and science to help me make the right decisions. Go back to my first question. How do I understand what customers expect to find? It’s not straightforward. Can the data tell us that our customers perceive our product as competitively priced even though it comes in a different bottle, it's got a different formulation, and there might be quality differences? It turns out that, yes, we can use data and ML to understand how customers evaluate the value proposition of our products. This information is useful both in how we build products and how we price them.\n\nAnother idea that’s super exciting to me, and which seems obvious, is you need customer feedback to improve your products. At Amazon we get feedback on a very large scale. We get it through customer reviews. We can use ML and statistics to dive deep into that information and use it to produce anecdotes and feedback signals that we use to improve the quality, pricing, and overall customer experience for our products. All of our competitor retailers building private label products are asking the same questions about how to improve products for customers. But at Amazon Private Brands we’re asking how we can do this analysis faster and in a more automated fashion to quickly get the insights back to our manufacturers.\n\nToday, we implement traditional quality processes that you would expect from any large manufacturer. We are with the best in class there. That said, we can go much further filtering all of the customer information we're getting through reviews and use it to inform our manufacturing partners to start this process of continual improvement and close the gap between customers and manufacturers. I want us to build the ML services that allow us to quickly determine from customer feedback where there might be issues or opportunities anywhere in our production. We make shampoo. We make toilet paper. We make batteries. We make T-shirts. We make a large variety of products, and we come at it from a very Amazonian point of view which is to apply a data-centric mindset. And that, in turn, leads us to concepts from the book.\n\nABOUT THE AUTHOR\n\n#### **Staff writer**","render":"<p><img src=\\"https://dev-media.amazoncloud.cn/3ac0169f5d2b4705b9f1452e2a1de04d_image.png\\" alt=\\"image.png\\" /></p>\n<p>Matt Taddy (top right), vice president of Amazon’s Private Brands business, is the coauthor of a recently released data-science textbook, Modern Business Analytics, along with Leslie Hendrix (middle right), and Matthew C. Harding.</p>\n<p>Matt Taddy, vice president of Amazon’s Private Brands business, is the coauthor of <a href=\\"https://www.amazon.com/Modern-Business-Analytics-Matt-Taddy/dp/1264071671/ref=sr_1_1?crid=3M38RU1Z95VYO&keywords=Modern+Business+Analytics&qid=1651095743&sprefix=modern+business+analytics%2Caps%2C196&sr=8-1\\" target=\\"_blank\\">Modern Business Analytics</a>: Practical Data Science for Decision Making, a primer for those who want to gain the skills to use data science to help make decisions in business and beyond.</p>\\n<p>When Matt Taddy earned his PhD in applied mathematics and statistics from the University of California, Santa Cruz, in 2008, the notion of a data-science specialization was still in its infancy.</p>\n<p>Today, the business-analytics profession, or the discipline of using data to make business, public policy, public health, and other decisions, is blossoming, and Taddy is excited about how the field is becoming more multi-disciplinary, incorporating statistics, machine learning, economics, and even the social sciences.</p>\n<p>“I benefitted from getting involved in the early stages before it became more specialized,” says Taddy.</p>\n<p>Since earning his PhD, Taddy has been a research assistant at NASA Ames Research Center and Sandia National Laboratories, a research fellow at eBay, the head of economics and data science for Business AI at Microsoft, a professor of econometrics and statistics at the University of Chicago Booth School of Business, the chief economist for Amazon’s North America Consumer organization, and now vice president of Amazon’s <a href=\\"https://jobs-us-east.amazon.com/en/teams/amazon-private-brands\\" target=\\"_blank\\">Private Brands</a> business. His first textbook, <a href=\\"https://www.amazon.com/Business-Data-Science-Combining-Accelerate/dp/1260452778\\" target=\\"_blank\\">Business Data Science</a>, was published by McGraw Hill in 2019. At the time, he told <a href=\\"https://www.amazon.science/business-data-science-is-a-lot-more-than-just-making-predictions-matt-taddy\\" target=\\"_blank\\">Amazon Science</a> that he began work on the book ten years prior when teaching a class of MBA students at the University of Chicago.</p>\\n<p>“I realized that there was an appetite for the material covered in the book from people who weren’t specialists in statistics or machine learning,” he said. “This idea that we could teach this material to non-specialists really motivated me not to just write this book, but also to push for changing the curriculum at the University of Chicago.”</p>\n<p>Since publishing that textbook in 2019, his role at Amazon has evolved as has his interest in making great decisions from data. The result is a new textbook, <a href=\\"https://www.amazon.com/Modern-Business-Analytics-Matt-Taddy/dp/1264071671/ref=sr_1_1?crid=3M38RU1Z95VYO&keywords=Modern+Business+Analytics&qid=1651095743&sprefix=modern+business+analytics%2Caps%2C196&sr=8-1\\" target=\\"_blank\\">Modern Business Analytics: Practical Data Science for Decision Making</a>, which Taddy co-authored with <a href=\\"https://sc.edu/study/colleges_schools/moore/directory/hendrix.leslie.php\\" target=\\"_blank\\">Leslie Hendrix</a>, PhD, associate professor at the Darla Moore School of Business at the University of South Carolina, and <a href=\\"https://www.socsci.uci.edu/~harding1/\\" target=\\"_blank\\">Matthew C. Harding</a>, PhD, professor of economics and statistics at the University of California, Irvine.</p>\\n<p><video src=\\"https://dev-media.amazoncloud.cn/3d8e7336321b427b91588ab4642b1155_Matt%20Taddy%20-%20Modern%20Business%20Analytics%20Author%20Video.mp4\\" controls=\\"controls\\"></video></p>\\n<p>According to the authors, “This book is a primer for those who want to gain the skills to use data science to help make decisions in business and beyond. The modern business analyst uses tools from machine learning, economics, and statistics to not only track what has happened but predict the future for their businesses.”</p>\n<p>McGraw Hill, the book’s publisher, says: “This new higher-ed text takes a practical, modern approach to data science and business analytics for the analytics student and professional. It gives students the opportunity to learn by doing, with real data analysis examples that explain the ‘why’, rather than the ‘what’ in decision-making discussions. It uses R as the primary technology through the text and includes an end-of-chapter reference to the basic R recipes in each chapter. Modern Business Analytics: Practical Data Science for Decision Making has crossed the boundaries and created something truly interdisciplinary.”</p>\n<p>Amazon Science connected with Taddy to discuss how his thinking about the topic has evolved in the past three years, his belief that deeper business decisions require focusing on why things happen versus what has happened, and how he’s applying modern business analytics techniques in running Amazon’s Private Brands business.</p>\n<h4><a id=\\"Q_In_2019_you_authored_Business_Data_Science_that_brought_together_concepts_from_statistics_machine_learning_and_the_social_sciences_to_help_businesses_use_data_more_effectively_How_has_your_thinking_evolved_in_the_past_three_years_And_how_does_Modern_Business_Analytics_address_that_26\\"></a><strong>Q. In 2019 you authored Business Data Science that brought together concepts from statistics, machine learning, and the social sciences to help businesses use data more effectively. How has your thinking evolved in the past three years? And how does Modern Business Analytics address that?</strong></h4>\\n<p><strong>A.</strong> Modern Business Analytics is a direct follow to Business Data Science. From Business Data Science we learned there is an audience, but I received feedback from a number of professors who, for example, were teaching from Business Data Science for MBA classes, or advanced undergraduate data-science classes, or master’s in public policy programs, that we didn’t really deliver the content in a format that was accessible to a broader audience.<br />\\nMcGraw Hill approached me again and said there was an opportunity to do a better job serving a wider audience and asked if I would be interested. My response was ‘Of course. One reason I did the book initially was to try and hit the widest audience possible.’ Recognizing that I’m busy with my day job here at Amazon, McGraw Hill suggested I approach co-authors to help with content development. Fortunately, both Leslie and Matthew agreed to contribute. Matthew teaches from this book in an MBA program at the University of California, Irvine, and Leslie teaches from a version of this book for a business-analytics program at the University of South Carolina.</p>\n<p>It is finally here!!! <a href=\\"https://twitter.com/hashtag/businessanalytics?src=hash&ref_src=twsrc%5Etfw\\" target=\\"_blank\\">#businessanalytics</a> <a href=\\"https://twitter.com/MattTaddy?ref_src=twsrc%5Etfw\\" target=\\"_blank\\">@MattTaddy</a> <a href=\\"https://twitter.com/hashtag/EconTwitter?src=hash&ref_src=twsrc%5Etfw\\" target=\\"_blank\\">#EconTwitter</a> <a href=\\"https://twitter.com/hashtag/MachineLearning?src=hash&ref_src=twsrc%5Etfw\\" target=\\"_blank\\">#MachineLearning</a> <a href=\\"https://t.co/rT2IWJdwM5\\" target=\\"_blank\\">pic.twitter.com/rT2IWJdwM5</a>— Matthew Harding 🏳️🌈 (@BigDataMetrics) <a href=\\"https://twitter.com/BigDataMetrics/status/1515111807811158016?ref_src=twsrc%5Etfw\\" target=\\"_blank\\">April 15, 2022</a></p>\\n<p>They’ve both experienced the challenges of onboarding students who have no exposure to programming languages, or students who are less proficient in math than the students I was originally exposed to when I wrote the material for Business Data Science. Leslie and Matthew brought a great new perspective to the project. Generally, you’re never happy with the first version of anything. Leslie and Matthew helped simplify some of the explanations provided in the previous book and contributed more examples. From my experience this is what students benefit from the most. The result: we were able to include many more real-world examples into Modern Business Analytics and make the new book far more accessible to a broader audience.</p>\n<p>In education it often takes a while for someone to develop an introductory-level book that pulls material from multiple disciplines and brings readers to the current state of the art. That’s what we challenged ourselves to deliver here. Our audience is anyone who wants to get the skills to use modern large-scale data to make decisions, whether they are in business, government, science, or anywhere else.</p>\n<h4><a id=\\"Q_It_would_seem_that_todays_modern_business_analyst_must_be_multidisciplinary_with_machine_learning_economics_statistics_and_other_skills_Whats_the_skill_set_you_look_for_37\\"></a><strong>Q. It would seem that today’s modern business analyst must be multidisciplinary, with machine learning, economics, statistics, and other skills. What’s the skill set you look for?</strong></h4>\\n<p><strong>A.</strong> I haven’t found an individual with all of those ingredients in equal measure. It is more about how you build a team with a diversity of skills and backgrounds. Data scientists, research scientists, applied scientists, and economists all use the tools that we discuss in the book. When you’re building a team focused on making decisions from data, you don’t want individuals with the same skills. You want individuals with different levels of emphasis. Some are going to have a much stronger background in computer science. They’re going to understand the algorithms component better. Others are going to have a stronger background in uncertainty quantification and the mathematics of what I refer to as modern statistics. Some will have an economics background element. Others will be comfortable addressing causal inference and structural analysis.<br />\\nWhat’s been really exciting about data science in the past 10 years is that we’ve created a common vocabulary so individuals from many disciplines can talk to each other. Today, you can build a team that has economists, applied scientists, research scientists, machine learning engineers, and data scientists working together to address a common challenge. When I first got into data science more than a decade ago this common vocabulary didn’t exist. There was a real boundary to working on data across disciplines. Fortunately, much of this has gone away. Now the economists and the machine-learning practitioners speak the same lingo making it much easier to build the diverse teams required to make decisions from data.</p>\n<p><img src=\\"https://dev-media.amazoncloud.cn/878ad064d53240c0a70e2d522523a906_2.png\\" alt=\\"2.png\\" /></p>\n<p>I mentioned causal inference and structural analysis previously and want to point out another aspect of the book that is unique. A lot of work inside a tech company can focus on pure prediction, what I would consider standard machine learning problems where you want to discover patterns in correlation. For a broader audience beyond machine learners, we need to understand how to make policy decisions – how to use data to decide between option A or option B. For that type of decision-making you really have to get into the structure of why things are happening.</p>\n<p>I took that seriously in the first book and doubled down on it with this book. For example, we have a chapter that’s dedicated to either fully randomized experiments or quasi experimental settings. These are A/B experiments, or what we refer to at Amazon as Weblabs. If you’re familiar with these experiments you know they aren’t nearly as simple as the term A/B implies. There’s a lot of complexity to these experiments — how you run them, how you analyze them. As a result, we focus a lot of attention on how to structure these A/B style trials and how you analyze data that has some experimental randomization as part of it.</p>\n<p>Another chapter is devoted to understanding why things are happening when you’re working from purely observational data. Here we go deep into some of the methods we use heavily in industry — orthogonal or double machine learning using high dimensional control sets and other things such as synthetic controls. This chapter codifies the methods for utilizing causal analysis and structural analysis in observational settings. As mentioned previously, I think this differentiates the book from others for this audience. Causal inference can be intimidating and you don’t often see it addressed at this level. It will be a high bar for some students, but the feedback we’ve received from professors who are teaching from early versions of our work is that students, especially those with some industry experience, are really attracted to the material. The students are attracted to it because they have worked in industry and know how important it is to be able to properly conduct experiments and perform causal analyses.</p>\n<h4><a id=\\"Q_Can_you_provide_some_context_about_Amazons_Private_Brands_business_and_how_youre_applying_modern_business_analytics_to_make_better_decisions_for_your_business_50\\"></a><strong>Q. Can you provide some context about Amazon’s Private Brands business, and how you’re applying modern business analytics to make better decisions for your business.</strong></h4>\\n<p><strong>A.</strong> I took over the role leading our worldwide Private Brands organization within the last year and much of what we do is seemingly simple, straightforward customer-centric product development. When I think about our business, I think about what product assortment we need in the long term. To determine this, I have to understand what products our customers expect from Amazon private brands and what they are able to get in private-label format from our competitors. Those are fairly straightforward business questions to address. Our customers expect us to have really attractive prices, so we need to ensure that our customers find sharp every-day low prices for the products that we’re providing. And our customers expect quality. We need to make sure that they are getting the quality they expect and that our manufacturers are getting feedback from customers that allows them to produce better products.</p>\\n<p>That all sounds like pretty vanilla stuff. I could be talking about any number of MBA case studies and all of our competitor retailers are asking similar questions of their private label businesses.</p>\n<h4><a id=\\"I_want_us_to_build_the_ML_services_that_allow_us_to_quickly_determine_from_customer_feedback_where_there_might_be_issues_or_opportunities_anywhere_in_our_production__56\\"></a>**“I want us to build the ML services that allow us to quickly determine from customer feedback where there might be issues or opportunities anywhere in our production.” **</h4>\\n<p>Matt Taddy</p>\n<p>But then I think about how I can use data and science to help me make the right decisions. Go back to my first question. How do I understand what customers expect to find? It’s not straightforward. Can the data tell us that our customers perceive our product as competitively priced even though it comes in a different bottle, it’s got a different formulation, and there might be quality differences? It turns out that, yes, we can use data and ML to understand how customers evaluate the value proposition of our products. This information is useful both in how we build products and how we price them.</p>\n<p>Another idea that’s super exciting to me, and which seems obvious, is you need customer feedback to improve your products. At Amazon we get feedback on a very large scale. We get it through customer reviews. We can use ML and statistics to dive deep into that information and use it to produce anecdotes and feedback signals that we use to improve the quality, pricing, and overall customer experience for our products. All of our competitor retailers building private label products are asking the same questions about how to improve products for customers. But at Amazon Private Brands we’re asking how we can do this analysis faster and in a more automated fashion to quickly get the insights back to our manufacturers.</p>\n<p>Today, we implement traditional quality processes that you would expect from any large manufacturer. We are with the best in class there. That said, we can go much further filtering all of the customer information we’re getting through reviews and use it to inform our manufacturing partners to start this process of continual improvement and close the gap between customers and manufacturers. I want us to build the ML services that allow us to quickly determine from customer feedback where there might be issues or opportunities anywhere in our production. We make shampoo. We make toilet paper. We make batteries. We make T-shirts. We make a large variety of products, and we come at it from a very Amazonian point of view which is to apply a data-centric mindset. And that, in turn, leads us to concepts from the book.</p>\n<p>ABOUT THE AUTHOR</p>\n<h4><a id=\\"Staff_writer_68\\"></a><strong>Staff writer</strong></h4>\n"}