{"value":"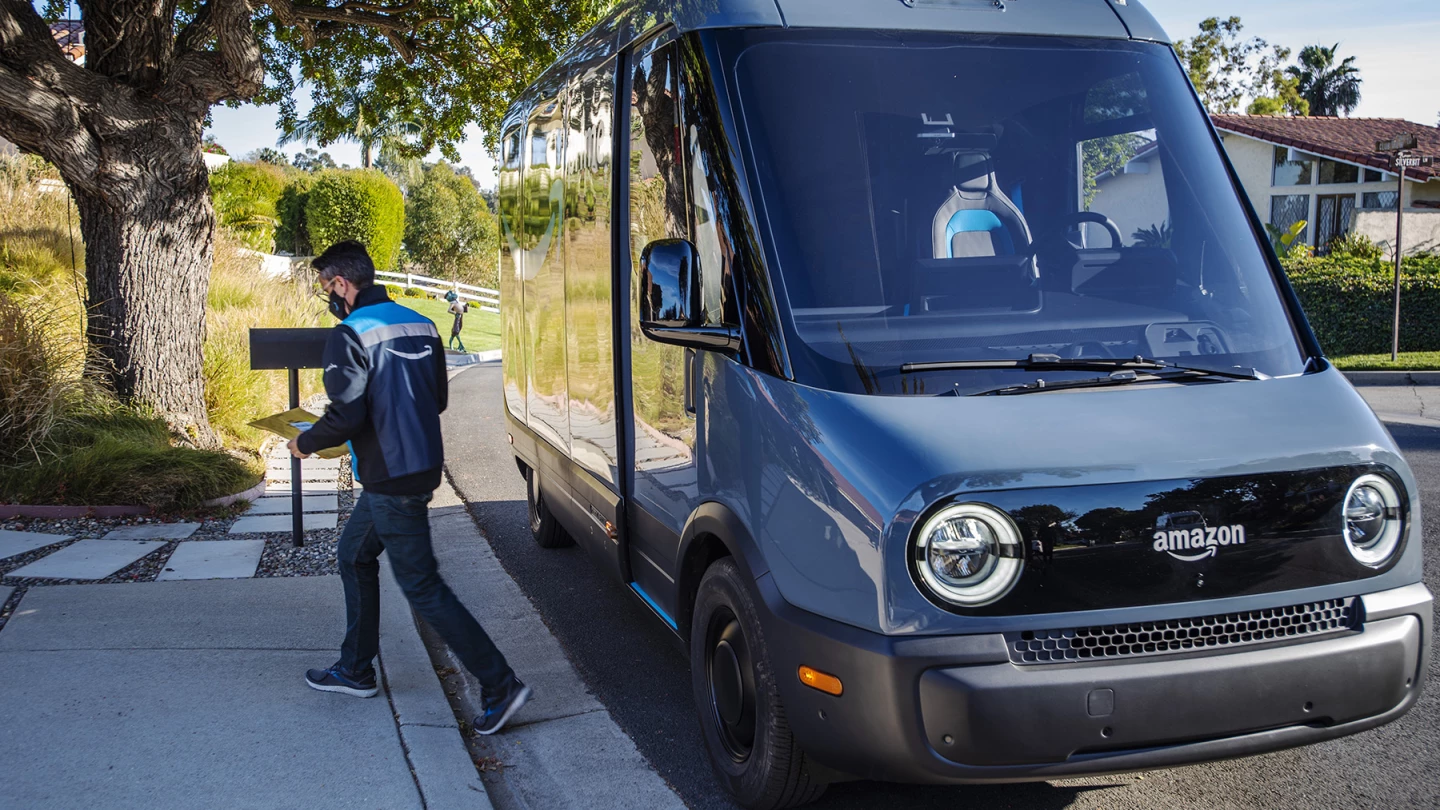\n\nWhen Amazon announced it would purchase 100,000 custom electric delivery vehicles, a team of scientists within the Amazon Logistics Research organization took on the challenge of determining the best strategy for deploying them.\n\nABOUT AMAZON\n\nWhen Amazon announced it would purchase 100,000 custom ++[electric delivery vehicles](https://www.aboutamazon.com/news/transportation/introducing-amazons-first-custom-electric-delivery-vehicle)++ as part of ++[The Climate Pledge](https://sustainability.aboutamazon.com/about/the-climate-pledge)++, a team of scientists within the Amazon Logistics (AMZL) Research organization took on the challenge of determining the best strategy for deploying them. Based on sophisticated models that simulate Amazon’s shipments and external parameters like power availability in each city, the team is developing a plan to gradually electrify Amazon’s entire fleet.\n\nThis is just one of many projects the AMZL Research Science team is tackling related to last-mile delivery. Last mile, as the name implies, is the last leg of the journey of a product to a customer’s doorstep. The team develops models to predict shipments per route (SPR) and distribution, which is the average number of packages delivered by a single driver in a given city on a given day (weeks to years in the future). These models help to predict the number and the different sizes of vans the company should purchase to meet the predicted demands.\n\n“With these complex models we develop, we have been influencing the company’s investment in vehicles, Delivery Service Partners, and their drivers,” says ++[Rohit Malshe](https://www.linkedin.com/in/rohit-malshe-90b0026/)++, a principal research scientist at Amazon.\n\n\n#### **How to forecast when everything is changing**\n\n\nThere are multiple scientific challenges involved in developing these models given the dynamic nature of Amazon’s operations.\n\n“One of these challenges is that our volume keeps growing. In general, as the volume grows, the shipments per route will also increase, but not linearly,” explains ++[Abhilasha Katariya](https://www.linkedin.com/in/abhilashakatariya/)++, a senior research scientist on the team. New delivery stations are frequently launched, leading to several changes in the geographical area that each station covers. Stations may incorporate different types of vehicles and modify their operation hours, which also impacts how much they can deliver. Additionally, road networks are subject to alterations as well, impacting driving time.\n\nThe team’s scientists must develop models that can handle the variability and complexity. To do that, they use a bottoms-up approach that starts at the zip code level. “This creates a foundation where any changes in the stations’ jurisdiction can be taken into consideration directly,” says Katariya.\n\n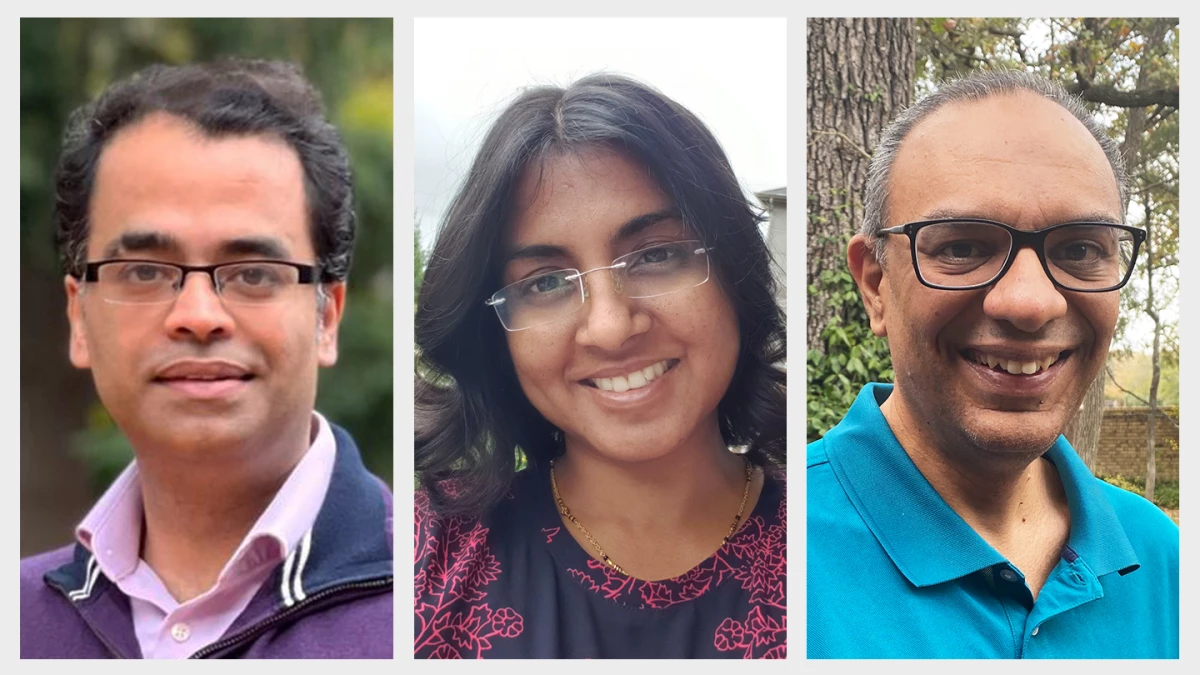\n\nLeft to right, Rohit Malshe, principal research scientist; Abhilasha Katariya, senior research scientist; and Natarajan Gautam, an Amazon Scholar and a professor at Texas A&M University, are all part of the Amazon Logistics Research Science team.\n\nPure machine-learning approaches are not adequate because the team must frequently make predictions based on new scenarios, for which there is no training data available. To compensate for the lack of training data, the team develops models that combine machine learning and physics-based models that have an optimization component which helps to take into account new variables.\n\nFor example, if a large van is added to an Amazon station that previously only worked with small and medium vans, there is no training data to inform the model. “But because the core of the model uses analytical and optimization components, we can still predict the shipments per route for a larger van,” says Katariya.\n\n“If you think about a machine learning model, typically interpolating is very easy. But, in our case, we typically want to extrapolate because we're always getting more volume,” says ++[Natarajan Gautam](https://www.linkedin.com/in/natarajan-gautam-62289a65/)++, an Amazon Scholar and a professor at Texas A&M University. “Using historical data to extrapolate is generally not recommended in machine learning, because you haven’t seen those things in the past.”\n\nThis is where the physics-based model comes in handy, although a pure physics-based model also wouldn’t work, notes Gautam, because there are so many simplifying assumptions that need to be made to obtain an analytically tractable model. “We want to get the best of both worlds, in some sense. We all want something that adequately represents what is observed, but we also want to be able to extrapolate when not observed.”\n\nAnother strategy the team employs to deal with situations where the parameters are constantly changing is to run the same model over and over again to do a type of course correction. “Just run the model every month, so that all the parameters that are changing are learned by the model, and then you are always getting the latest and greatest picture you should expect. This way you have a good model that handles all types of situations, even the ones where no data exists,” says Malshe.\n\nThe science team works very closely with people on the ground, both in station and on the road, to perfect these models. They frequently visit the delivery stations and interview the drivers whenever an opportunity arrives. “We make visits to stations and do ride-alongs so that we stay connected with how the business is evolving,” says Katariya. \n\nIn one of these meetings, Gautam says, station employees said their results were different from what the models were predicting. “We went back to the drawing board, looked at the code and the data they were getting ,and took a deep dive to find what was causing the problem”.\n\nThey realized the station started delivering to a new zip code, but it didn’t perform the same way the previous station did. That explained the difference between what the model was observing and the real-life data. Having a close connection with operations allowed them to identify the problem and adjust their model.\n\n\n#### **Dealing with COVID-19 disruptions**\n\n\nFor big decisions like vehicle purchases, the AMZL Research Science team forecasts on a 16-month horizon. However, when the team predicted the number of vans needed for 2020, their model didn’t consider the COVID-19 pandemic. “Suddenly there was so much more package demand that all our forecasts were basically incorrect,” says Malshe.\n\n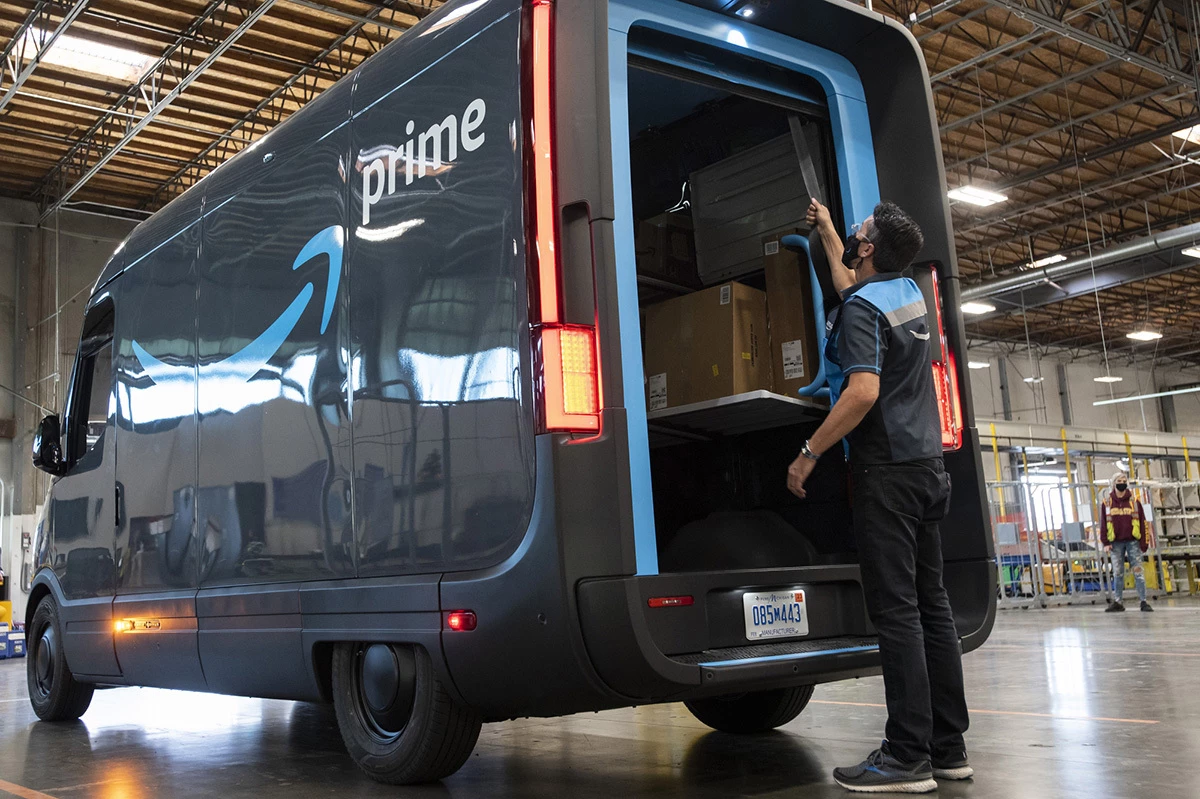\n\nFor big decisions like vehicle purchases, the AMZL Research Science team forecasts on a 16-month horizon.\n\nABOUT AMAZON\n\nHe says, when situations like these arise, the first thing the team does is to upgrade the forecasts to incorporate the additional volume. They also perform scenario analyses to check, for example, if the vehicles that had already been budgeted and procured would serve the purpose. Fortunately, in this case, because these decisions are made so far in advance, the team intentionally overbudgeted to account for uncertainties. “Luckily enough, the previous year, we had spent a lot of money on bigger vehicles, and they were able to absorb the additional package volume. So, when we ran these forecasts, we figured out we were in a good spot to be able to handle such changes,” says Malshe.\n\n“Another risk mitigation lever we applied is to make sure there is enough storage space in the delivery stations,” says Malshe. “We made sure we looked into every possible parameter to optimize for vehicles and their placement in various cities, and their deployment to various Delivery Service Partner companies so that they are utilized to the best of our capabilities.”\n\n\n#### **‘Many challenges and interesting solutions’**\n\n\nThe electrification of Amazon’s fleet presents its own set of challenges. Some of these include how to make sure batteries in the vehicles don’t run out of charge on the road; how to optimize electricity and power consumption; and how to account for extreme weather, long trips and hilly areas. “We will keep learning on all of these items as we go forward, and each year we will come up with more innovations to overcome any barriers,” says Malshe. \n\nFor Malshe, the diversity of the scientists working on the team – which includes people with various backgrounds, industries, educations, and skill sets – is what contributes to its success in tackling these unresolved challenges.\n\n\"We have people on our team who are extremely data savvy. We have team members who know SQL coding in depth and some are extremely good in Python coding. Other team members have expertise in areas like machine learning, optimization, pure modeling, Monte Carlo simulations and what not,\" says Malshe, who is himself a chemical engineer with experience in logistics.\n\n “Usually two to three people are working on every project. It divides and conquers various tasks and ultimately gives everyone an opportunity to do valuable work,” he says.\n\nIn addition to the team’s range of expertise, Katariya says another team success factor is its ability to collaborate on a wide range of problems. “Each problem has a different challenge, some have a very simple mathematical solution, but are very heavy on the implementation side, and others may require more complex models from a mathematical perspective, but are easier to implement.”\n\nAnd there are many more challenges to be tackled. In fact, Gautam says, some of his peers have yet to fully grasp the challenges involved in this field of research.\n\n“A lot of people think of last mile as solving ++[a vehicle routing problem](https://www.sciencedirect.com/topics/economics-econometrics-and-finance/vehicle-routing-problem)++. But we do a lot more than that,” he says. “There are so many challenges and interesting solutions that you just can’t take it off the shelf, you really have to invent as you go along. There are tremendous opportunities to do that here and the range of challenges we get to address is what makes being involved with this team so professionally rewarding.”\n\nABOUT THE AUTHOR\n\n#### **[Mariana Lenharo](https://www.amazon.science/author/mariana-lenharo)**\n\nMariana is a science and health journalist based in Brazil.","render":"<p><img src=\"https://dev-media.amazoncloud.cn/33066cb21e1947248b23ac872c8f22cd_image.png\" alt=\"image.png\" /></p>\n<p>When Amazon announced it would purchase 100,000 custom electric delivery vehicles, a team of scientists within the Amazon Logistics Research organization took on the challenge of determining the best strategy for deploying them.</p>\n<p>ABOUT AMAZON</p>\n<p>When Amazon announced it would purchase 100,000 custom <ins><a href=\"https://www.aboutamazon.com/news/transportation/introducing-amazons-first-custom-electric-delivery-vehicle\" target=\"_blank\">electric delivery vehicles</a></ins> as part of <ins><a href=\"https://sustainability.aboutamazon.com/about/the-climate-pledge\" target=\"_blank\">The Climate Pledge</a></ins>, a team of scientists within the Amazon Logistics (AMZL) Research organization took on the challenge of determining the best strategy for deploying them. Based on sophisticated models that simulate Amazon’s shipments and external parameters like power availability in each city, the team is developing a plan to gradually electrify Amazon’s entire fleet.</p>\n<p>This is just one of many projects the AMZL Research Science team is tackling related to last-mile delivery. Last mile, as the name implies, is the last leg of the journey of a product to a customer’s doorstep. The team develops models to predict shipments per route (SPR) and distribution, which is the average number of packages delivered by a single driver in a given city on a given day (weeks to years in the future). These models help to predict the number and the different sizes of vans the company should purchase to meet the predicted demands.</p>\n<p>“With these complex models we develop, we have been influencing the company’s investment in vehicles, Delivery Service Partners, and their drivers,” says <ins><a href=\"https://www.linkedin.com/in/rohit-malshe-90b0026/\" target=\"_blank\">Rohit Malshe</a></ins>, a principal research scientist at Amazon.</p>\n<h4><a id=\"How_to_forecast_when_everything_is_changing_13\"></a><strong>How to forecast when everything is changing</strong></h4>\n<p>There are multiple scientific challenges involved in developing these models given the dynamic nature of Amazon’s operations.</p>\n<p>“One of these challenges is that our volume keeps growing. In general, as the volume grows, the shipments per route will also increase, but not linearly,” explains <ins><a href=\"https://www.linkedin.com/in/abhilashakatariya/\" target=\"_blank\">Abhilasha Katariya</a></ins>, a senior research scientist on the team. New delivery stations are frequently launched, leading to several changes in the geographical area that each station covers. Stations may incorporate different types of vehicles and modify their operation hours, which also impacts how much they can deliver. Additionally, road networks are subject to alterations as well, impacting driving time.</p>\n<p>The team’s scientists must develop models that can handle the variability and complexity. To do that, they use a bottoms-up approach that starts at the zip code level. “This creates a foundation where any changes in the stations’ jurisdiction can be taken into consideration directly,” says Katariya.</p>\n<p><img src=\"https://dev-media.amazoncloud.cn/3a04ddf6df0b47e399e71be078dc0552_image.png\" alt=\"image.png\" /></p>\n<p>Left to right, Rohit Malshe, principal research scientist; Abhilasha Katariya, senior research scientist; and Natarajan Gautam, an Amazon Scholar and a professor at Texas A&M University, are all part of the Amazon Logistics Research Science team.</p>\n<p>Pure machine-learning approaches are not adequate because the team must frequently make predictions based on new scenarios, for which there is no training data available. To compensate for the lack of training data, the team develops models that combine machine learning and physics-based models that have an optimization component which helps to take into account new variables.</p>\n<p>For example, if a large van is added to an Amazon station that previously only worked with small and medium vans, there is no training data to inform the model. “But because the core of the model uses analytical and optimization components, we can still predict the shipments per route for a larger van,” says Katariya.</p>\n<p>“If you think about a machine learning model, typically interpolating is very easy. But, in our case, we typically want to extrapolate because we’re always getting more volume,” says <ins><a href=\"https://www.linkedin.com/in/natarajan-gautam-62289a65/\" target=\"_blank\">Natarajan Gautam</a></ins>, an Amazon Scholar and a professor at Texas A&M University. “Using historical data to extrapolate is generally not recommended in machine learning, because you haven’t seen those things in the past.”</p>\n<p>This is where the physics-based model comes in handy, although a pure physics-based model also wouldn’t work, notes Gautam, because there are so many simplifying assumptions that need to be made to obtain an analytically tractable model. “We want to get the best of both worlds, in some sense. We all want something that adequately represents what is observed, but we also want to be able to extrapolate when not observed.”</p>\n<p>Another strategy the team employs to deal with situations where the parameters are constantly changing is to run the same model over and over again to do a type of course correction. “Just run the model every month, so that all the parameters that are changing are learned by the model, and then you are always getting the latest and greatest picture you should expect. This way you have a good model that handles all types of situations, even the ones where no data exists,” says Malshe.</p>\n<p>The science team works very closely with people on the ground, both in station and on the road, to perfect these models. They frequently visit the delivery stations and interview the drivers whenever an opportunity arrives. “We make visits to stations and do ride-alongs so that we stay connected with how the business is evolving,” says Katariya.</p>\n<p>In one of these meetings, Gautam says, station employees said their results were different from what the models were predicting. “We went back to the drawing board, looked at the code and the data they were getting ,and took a deep dive to find what was causing the problem”.</p>\n<p>They realized the station started delivering to a new zip code, but it didn’t perform the same way the previous station did. That explained the difference between what the model was observing and the real-life data. Having a close connection with operations allowed them to identify the problem and adjust their model.</p>\n<h4><a id=\"Dealing_with_COVID19_disruptions_43\"></a><strong>Dealing with COVID-19 disruptions</strong></h4>\n<p>For big decisions like vehicle purchases, the AMZL Research Science team forecasts on a 16-month horizon. However, when the team predicted the number of vans needed for 2020, their model didn’t consider the COVID-19 pandemic. “Suddenly there was so much more package demand that all our forecasts were basically incorrect,” says Malshe.</p>\n<p><img src=\"https://dev-media.amazoncloud.cn/7862d8181e734e219b59a5bf6076e475_image.png\" alt=\"image.png\" /></p>\n<p>For big decisions like vehicle purchases, the AMZL Research Science team forecasts on a 16-month horizon.</p>\n<p>ABOUT AMAZON</p>\n<p>He says, when situations like these arise, the first thing the team does is to upgrade the forecasts to incorporate the additional volume. They also perform scenario analyses to check, for example, if the vehicles that had already been budgeted and procured would serve the purpose. Fortunately, in this case, because these decisions are made so far in advance, the team intentionally overbudgeted to account for uncertainties. “Luckily enough, the previous year, we had spent a lot of money on bigger vehicles, and they were able to absorb the additional package volume. So, when we ran these forecasts, we figured out we were in a good spot to be able to handle such changes,” says Malshe.</p>\n<p>“Another risk mitigation lever we applied is to make sure there is enough storage space in the delivery stations,” says Malshe. “We made sure we looked into every possible parameter to optimize for vehicles and their placement in various cities, and their deployment to various Delivery Service Partner companies so that they are utilized to the best of our capabilities.”</p>\n<h4><a id=\"Many_challenges_and_interesting_solutions_59\"></a><strong>‘Many challenges and interesting solutions’</strong></h4>\n<p>The electrification of Amazon’s fleet presents its own set of challenges. Some of these include how to make sure batteries in the vehicles don’t run out of charge on the road; how to optimize electricity and power consumption; and how to account for extreme weather, long trips and hilly areas. “We will keep learning on all of these items as we go forward, and each year we will come up with more innovations to overcome any barriers,” says Malshe.</p>\n<p>For Malshe, the diversity of the scientists working on the team – which includes people with various backgrounds, industries, educations, and skill sets – is what contributes to its success in tackling these unresolved challenges.</p>\n<p>“We have people on our team who are extremely data savvy. We have team members who know SQL coding in depth and some are extremely good in Python coding. Other team members have expertise in areas like machine learning, optimization, pure modeling, Monte Carlo simulations and what not,” says Malshe, who is himself a chemical engineer with experience in logistics.</p>\n<p>“Usually two to three people are working on every project. It divides and conquers various tasks and ultimately gives everyone an opportunity to do valuable work,” he says.</p>\n<p>In addition to the team’s range of expertise, Katariya says another team success factor is its ability to collaborate on a wide range of problems. “Each problem has a different challenge, some have a very simple mathematical solution, but are very heavy on the implementation side, and others may require more complex models from a mathematical perspective, but are easier to implement.”</p>\n<p>And there are many more challenges to be tackled. In fact, Gautam says, some of his peers have yet to fully grasp the challenges involved in this field of research.</p>\n<p>“A lot of people think of last mile as solving <ins><a href=\"https://www.sciencedirect.com/topics/economics-econometrics-and-finance/vehicle-routing-problem\" target=\"_blank\">a vehicle routing problem</a></ins>. But we do a lot more than that,” he says. “There are so many challenges and interesting solutions that you just can’t take it off the shelf, you really have to invent as you go along. There are tremendous opportunities to do that here and the range of challenges we get to address is what makes being involved with this team so professionally rewarding.”</p>\n<p>ABOUT THE AUTHOR</p>\n<h4><a id=\"Mariana_Lenharohttpswwwamazonscienceauthormarianalenharo_78\"></a><strong><a href=\"https://www.amazon.science/author/mariana-lenharo\" target=\"_blank\">Mariana Lenharo</a></strong></h4>\n<p>Mariana is a science and health journalist based in Brazil.</p>\n"}