{"value":"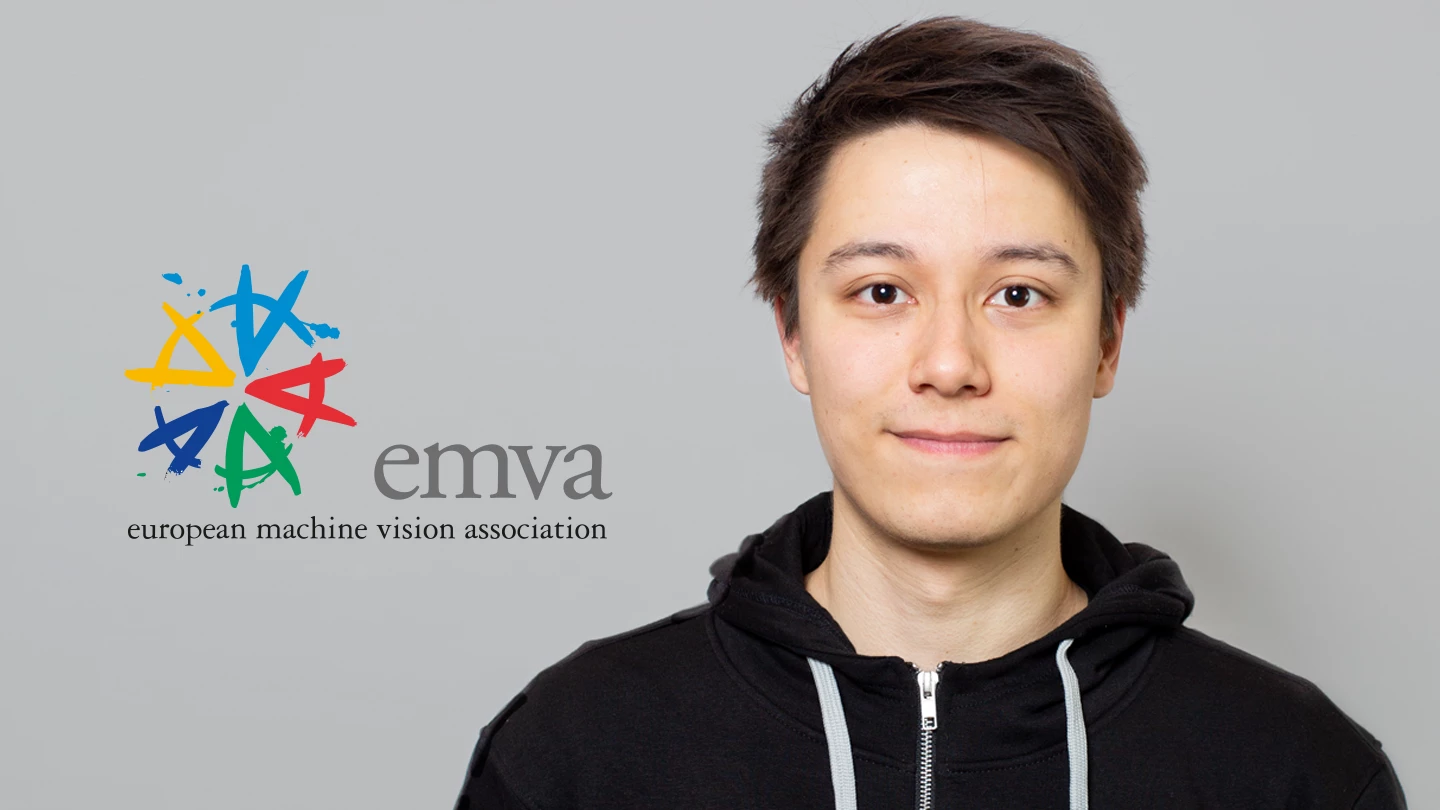\n\nKarsten Roth, a PhD student at the University of Tübingen, was awarded the EMVA Young Professional Award for work done during his [science internship at Amazon](https://www.amazon.science/tag/internships) on “[Towards Total Recall in Industrial Anomaly Detection](https://www.amazon.science/publications/towards-total-recall-in-industrial-anomaly-detection)”.\n\n[Karsten Roth](https://scholar.google.com/citations?user=93ZjIs0AAAAJ&hl=de), a PhD student at the University of Tübingen, was awarded the EMVA Young Professional Award for work done during his science internship at Amazon on “[Towards Total Recall in Industrial Anomaly Detection](https://www.amazon.science/publications/towards-total-recall-in-industrial-anomaly-detection)”. Roth received the award on May 13 during the 20th EMVA Business Conference in Brussels.\n\nThe EMVA Young Professional Award is an annual award “to honor the outstanding and innovative work of a student or a young professional in the field of machine vision or image processing.”\n\nThe paper Roth authored, along with applied scientist [Latha Pemula](https://www.linkedin.com/in/latha-pemula-9a745023/), former senior applied scientist [Joaquin Zepeda](https://www.linkedin.com/in/joaquin-zepeda-02638899/), vice president and distinguished scientist [Bernhard Schölkopf](https://www.amazon.science/author/bernhard-scholkopf), Amazon Scholar [Thomas Brox](https://www.amazon.science/academic-engagements/computer-vision-researcher-thomas-brox-enjoys-university-and-industry-roles), and senior applied scientist [Peter Gehler](https://www.amazon.science/author/peter-gehler), who was also Roth’s supervisor, addresses what is known as the cold start problem in anomaly detection.\n\n“The project was for the service that became [Amazon Lookout for Vision](https://aws.amazon.com/lookout-for-vision/), which helps businesses, usually manufacturers, spot [defects and anomalies](https://www.amazon.science/tag/anomaly-detection),” Gehler explained. Lookout for Vision utilizes few-shot learning, meaning it is possible to assess products for defects based on relatively few examples of defective and normal products. However, a challenge arises when businesses only have nominal, or non-defective, product images available.\n\nThe problem that we looked at is the cold start problem, where you don't have any negative examples or defects,” Gehler said. The challenge extends to detecting defects that are hard to capture (eg thin scratches or missing parts) as well as spotting unforeseen and unanticipated defects.\n\nAfter holding the first place on industrial cold-start anomaly detection performance for effectively > 1 year now, I’m happy to be presenting 𝐏𝐚𝐭𝐜𝐡𝐂𝐨𝐫𝐞🔎 at [#CVPR2022](https://twitter.com/hashtag/CVPR2022?src=hash&ref_src=twsrc%5Etfw)!\n\nPaper: [https://t.co/f63qqHMQim](https://t.co/f63qqHMQim)\nRepo: [https://t.co/dzqCO6GenS](https://t.co/dzqCO6GenS)\n[@AmazonScience](https://twitter.com/AmazonScience?ref_src=twsrc%5Etfw)\n\n1/4 [pic.twitter.com/tP5heaVdwE](https://t.co/tP5heaVdwE)\n\n— Karsten Roth @CVPR2022 (@confusezius) [June 15, 2022](https://twitter.com/confusezius/status/1537106083579322371?ref_src=twsrc%5Etfw)\n\n“Our research was motivated by a real and practical challenge,” Roth explained. “The solution we developed had to be one of practical nature; driven to optimize performance and usability before caring about the ‘academic wow-factor’”.\n\nThe team identified a solution to the challenge by utilizing images of non-defective items. “There were many variants that we studied,” Gehler said. “The best option was based on building an image library of good examples and then testing nearness against this library.” Gehler noted that the question then becomes how ‘nearness’ is defined.\n\n“Being near to an example depends on how you take the image and how you encode the image,” he said. He added that, drawing from principles of deep learning architecture, the solution is to “turn an image into a series of vectors, and then you compare those vectors — and there's multiple ways of doing this at multiple stages.” By comparing products against non-defective examples and determining how closely or how far apart from that ideal the product is, defects can be flagged — even without actual examples of defects.\n\nThe team’s solution, PatchCore, achieved an image-level anomaly detection (AUROC) score of up to 99.6% on the widely used MVTec AD benchmark, “more than halving the error compared to the next best competitor.” Roth presented PatchCore this week at [CVPR 2022](https://www.amazon.science/conferences-and-events/cvpr-2022).\n\nGehler said Roth’s role was vital. “Karsten produced so many results each week that we didn’t have time to cover all of them in our hour-long weekly meeting.”\n\n“Receiving this year’s EMVA Young Professional Award provides further validation for the relevance and importance of this line of research, and I am delighted to have been awarded such a high-profile award,” Roth said.\n\nHe added that he is indebted to the team he interned under at Amazon.\n\n“A somewhat unexpected, but very welcome consequence of conducting research at Amazon were significant improvements in the quality of my code and the overall documentation of my research progress,” he said, “with particular thanks to Peter who made the whole research internship a superb experience.”\n\nABOUT THE AUTHOR\n\n#### **Staff writer**","render":"<p><img src=\\"https://dev-media.amazoncloud.cn/2cf4572ff8a045a39ce382f348f564a7_image.png\\" alt=\\"image.png\\" /></p>\n<p>Karsten Roth, a PhD student at the University of Tübingen, was awarded the EMVA Young Professional Award for work done during his <a href=\\"https://www.amazon.science/tag/internships\\" target=\\"_blank\\">science internship at Amazon</a> on “<a href=\\"https://www.amazon.science/publications/towards-total-recall-in-industrial-anomaly-detection\\" target=\\"_blank\\">Towards Total Recall in Industrial Anomaly Detection</a>”.</p>\\n<p><a href=\\"https://scholar.google.com/citations?user=93ZjIs0AAAAJ&hl=de\\" target=\\"_blank\\">Karsten Roth</a>, a PhD student at the University of Tübingen, was awarded the EMVA Young Professional Award for work done during his science internship at Amazon on “<a href=\\"https://www.amazon.science/publications/towards-total-recall-in-industrial-anomaly-detection\\" target=\\"_blank\\">Towards Total Recall in Industrial Anomaly Detection</a>”. Roth received the award on May 13 during the 20th EMVA Business Conference in Brussels.</p>\\n<p>The EMVA Young Professional Award is an annual award “to honor the outstanding and innovative work of a student or a young professional in the field of machine vision or image processing.”</p>\n<p>The paper Roth authored, along with applied scientist <a href=\\"https://www.linkedin.com/in/latha-pemula-9a745023/\\" target=\\"_blank\\">Latha Pemula</a>, former senior applied scientist <a href=\\"https://www.linkedin.com/in/joaquin-zepeda-02638899/\\" target=\\"_blank\\">Joaquin Zepeda</a>, vice president and distinguished scientist <a href=\\"https://www.amazon.science/author/bernhard-scholkopf\\" target=\\"_blank\\">Bernhard Schölkopf</a>, Amazon Scholar <a href=\\"https://www.amazon.science/academic-engagements/computer-vision-researcher-thomas-brox-enjoys-university-and-industry-roles\\" target=\\"_blank\\">Thomas Brox</a>, and senior applied scientist <a href=\\"https://www.amazon.science/author/peter-gehler\\" target=\\"_blank\\">Peter Gehler</a>, who was also Roth’s supervisor, addresses what is known as the cold start problem in anomaly detection.</p>\\n<p>“The project was for the service that became <a href=\\"https://aws.amazon.com/lookout-for-vision/\\" target=\\"_blank\\">Amazon Lookout for Vision</a>, which helps businesses, usually manufacturers, spot <a href=\\"https://www.amazon.science/tag/anomaly-detection\\" target=\\"_blank\\">defects and anomalies</a>,” Gehler explained. Lookout for Vision utilizes few-shot learning, meaning it is possible to assess products for defects based on relatively few examples of defective and normal products. However, a challenge arises when businesses only have nominal, or non-defective, product images available.</p>\\n<p>The problem that we looked at is the cold start problem, where you don’t have any negative examples or defects,” Gehler said. The challenge extends to detecting defects that are hard to capture (eg thin scratches or missing parts) as well as spotting unforeseen and unanticipated defects.</p>\n<p>After holding the first place on industrial cold-start anomaly detection performance for effectively > 1 year now, I’m happy to be presenting 𝐏𝐚𝐭𝐜𝐡𝐂𝐨𝐫𝐞🔎 at <a href=\\"https://twitter.com/hashtag/CVPR2022?src=hash&ref_src=twsrc%5Etfw\\" target=\\"_blank\\">#CVPR2022</a>!</p>\\n<p>Paper: <a href=\\"https://t.co/f63qqHMQim\\" target=\\"_blank\\">https://t.co/f63qqHMQim</a><br />\\nRepo: <a href=\\"https://t.co/dzqCO6GenS\\" target=\\"_blank\\">https://t.co/dzqCO6GenS</a><br />\\n<a href=\\"https://twitter.com/AmazonScience?ref_src=twsrc%5Etfw\\" target=\\"_blank\\">@AmazonScience</a></p>\\n<p>1/4 <a href=\\"https://t.co/tP5heaVdwE\\" target=\\"_blank\\">pic.twitter.com/tP5heaVdwE</a></p>\\n<p>— Karsten Roth @CVPR2022 (@confusezius) <a href=\\"https://twitter.com/confusezius/status/1537106083579322371?ref_src=twsrc%5Etfw\\" target=\\"_blank\\">June 15, 2022</a></p>\\n<p>“Our research was motivated by a real and practical challenge,” Roth explained. “The solution we developed had to be one of practical nature; driven to optimize performance and usability before caring about the ‘academic wow-factor’”.</p>\n<p>The team identified a solution to the challenge by utilizing images of non-defective items. “There were many variants that we studied,” Gehler said. “The best option was based on building an image library of good examples and then testing nearness against this library.” Gehler noted that the question then becomes how ‘nearness’ is defined.</p>\n<p>“Being near to an example depends on how you take the image and how you encode the image,” he said. He added that, drawing from principles of deep learning architecture, the solution is to “turn an image into a series of vectors, and then you compare those vectors — and there’s multiple ways of doing this at multiple stages.” By comparing products against non-defective examples and determining how closely or how far apart from that ideal the product is, defects can be flagged — even without actual examples of defects.</p>\n<p>The team’s solution, PatchCore, achieved an image-level anomaly detection (AUROC) score of up to 99.6% on the widely used MVTec AD benchmark, “more than halving the error compared to the next best competitor.” Roth presented PatchCore this week at <a href=\\"https://www.amazon.science/conferences-and-events/cvpr-2022\\" target=\\"_blank\\">CVPR 2022</a>.</p>\\n<p>Gehler said Roth’s role was vital. “Karsten produced so many results each week that we didn’t have time to cover all of them in our hour-long weekly meeting.”</p>\n<p>“Receiving this year’s EMVA Young Professional Award provides further validation for the relevance and importance of this line of research, and I am delighted to have been awarded such a high-profile award,” Roth said.</p>\n<p>He added that he is indebted to the team he interned under at Amazon.</p>\n<p>“A somewhat unexpected, but very welcome consequence of conducting research at Amazon were significant improvements in the quality of my code and the overall documentation of my research progress,” he said, “with particular thanks to Peter who made the whole research internship a superb experience.”</p>\n<p>ABOUT THE AUTHOR</p>\n<h4><a id=\\"Staff_writer_42\\"></a><strong>Staff writer</strong></h4>\n"}