{"value":"On the cusp of this year’s ++[NeurIPS conference](https://www.amazon.science/conferences-and-events/neurips-2021)++, three AI luminaries and Amazon-affiliated researchers — all of whom have given the conference’s major named lecture, the Posner lecture — took the time to speak with Amazon Science about the rise of the machine learning industry, its implications for both tech and AI research, and the path forward for AI.\n\nThe participants in the conversation were ++[Michael I. Jordan](https://www.amazon.science/artificial-intelligence-the-revolution-hasnt-happened-yet)++, a Distinguished ++[Amazon Scholar](https://www.amazon.science/scholars)++ and the Pehong Chen Distinguished Professor at the University of California, Berkeley; ++[Bernhard Schölkopf](https://www.amazon.science/latest-news/bernhard-scholkopf-wins-german-ai-innovation-award)++, an Amazon vice president and distinguished scientist and the director of the empirical-inference program at the Max Planck Institute for Intelligent Systems in Tübingen; and ++[Michael Kearns](https://www.amazon.science/latest-news/3-questions-with-michael-kearns-designing-socially-aware-algorithms-and-models)++, an Amazon Scholar and a professor in the Department of Computer and Information Science at the University of Pennsylvania.\n\n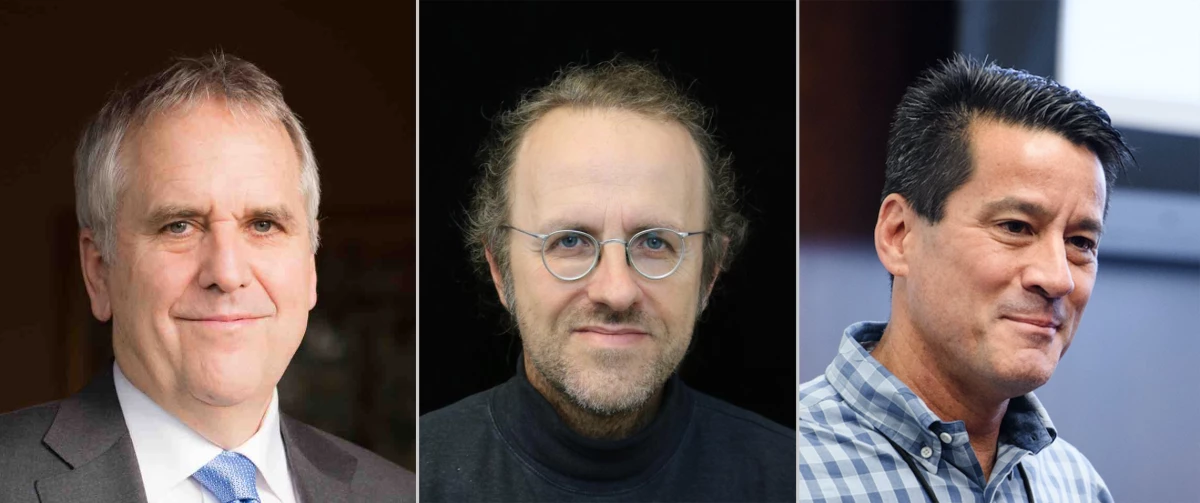\n\nJordan, Schölkopf, Kearns\n\nJordan argued that AI research should focus, not on the “imitation game” proposed by Alan Turing, but on the “complementarity game”.\n\n“I do not want autonomous, self-driving cars, just like I don't want autonomous, self-flying planes,” Jordan said. “I want them federated and talking to each other and sending high-level information back and forth and making plans together. … It's not just a car; it's a whole transportation system that gets people and packages around the world and should be thought of at that level. Really, we're building, like, a system that brings food into a city. We're building the entire system; we're not just bringing one piece of bread into the city autonomously, whatever that might mean.”\n\n“The goal is to federate; the goal is to build complementary systems that interact with each other, interact well with humans,” Jordan continued. “This style of thinking I see more in industry than I see in academia. In industry, you solve a problem, and you bring in people from all these different points of view, and you think through the problem and the consequences a little bit. Because if you build a product that fails on one of these dimensions, it's not going to work. So you do see more of this dialogue there. And I think that’s another way to go, to get our industry-academic connections to fire up some of these challenges and to push each other on both sides.”\n\nWhen Schölkopf gave his Posner lecture in 2011, before the deep-learning revolution, he was already concerned with the question of how machine learning models can incorporate causal reasoning.\n\n\n#### **This style of thinking I see more in industry than I see in academia. In industry, you solve a problem, and you bring in people from all these different points of view, and you think through the problem and the consequences.**\n\n\nMichael I. Jordan\n\n“Machine learning ultimately is based on statistical dependencies, and we usually don't ask where they actually come from,” Schölkopf said. “If two quantities are statistically dependent, it means that either one of them causes the other one, or there's something else that has caused both of them. And so in that sense, causality is a concept that describes the dependencies in the system on a more fundamental level that produces statistical dependencies on the surface. Oftentimes, it's enough if we work at the surface and just learn from these dependencies. But basically, it turns out that it's only enough as long as we're in this setting where nothing changes. Once things start changing, it's actually helpful to think about the causality.”\n\nIn most current work on causal reasoning in machine learning, Schölkopf explained, models attempt to determine causal relationships between variables specified in advance — say, the ++[prices of dairy products]()++ in a particular region. One fruitful path forward for causal-reasoning research, he argued, is models that learn, not only the causal relationships between variables but the variables themselves.\n\n“We have to develop this field of causal representation learning,” Schölkopf said. “How do we identify the useful variables in high-dimensional data? I think that's going to be interesting because current representation learning is really mostly about just learning statistical representations, which are useful for prediction but maybe not much more.”\n\nPicking up from Jordan’s contention that AI researchers need to think more about the place of AI agents in a larger social ecosystem, Kearns discussed the role that the scientific community should play in the regulation of AI.\n\n\n#### **Until we get regulation that is more in the language of algorithms itself, I think the gap between well-intentioned regulations and actual enforceability will remain very, very wide.**\n\n\nMichael Kearns\n\n“One slightly controversial opinion is that I really think algorithmic regulation needs to look much more algorithmic itself,” Kearns said. “At the end of the day, we're essentially building artifacts that are out in the world, making decisions, and will make decisions or predictions on any input you give them. The whole point of algorithms and machine learning is that you don't have to explicitly specify what you're going to do in every single corner case. But the model will do something in every corner case. And until we get regulation that really is more in the language of algorithms itself, I think the gap between well-intentioned regulations and actual enforceability will remain very, very wide.”\n\nAlthough he added that “I'll admit that I don't know how we'll close that gap,” Kearns did point to recent work on game theory as possibly pointing a way forward.\n\n“One framework that's emerged in recent years for essentially enforcing fairness constraints in the training of the model is very explicitly game theoretic, in which you basically design your algorithm in a way that sets it up as a two-player game, where one of the players is a learner of the traditional variety who generally is just concerned with predictive accuracy, and the other player you can think of as a regulator, who is there to enforce the fairness constraints,” Kearns said. “One thing that's interesting about that approach, though, is you could even imagine kind of ripping the regulator out of the code itself and actually having it be a literal regulator. So the same framework for algorithm design could be thought of as a crude model for what might actually be the real-world back-and-forth between, let's say, a tech regulator whose goal is to enforce anti-discrimination laws in predictive models and the regulatees.”\n\nA handful of excerpts, however, give only the flavor of what was a wide-ranging and stimulating conversation. Please watch the video to learn more about these distinguished scientists’ thoughts on the past and future of their field.\n\nABOUT THE AUTHOR\n\n#### **[Larry Hardesty](https://www.amazon.science/author/larry-hardesty)**\n\nLarry Hardesty is the editor of the Amazon Science blog. Previously, he was a senior editor at MIT Technology Review and the computer science writer at the MIT News Office.","render":"<p>On the cusp of this year’s <ins><a href=\\"https://www.amazon.science/conferences-and-events/neurips-2021\\" target=\\"_blank\\">NeurIPS conference</a></ins>, three AI luminaries and Amazon-affiliated researchers — all of whom have given the conference’s major named lecture, the Posner lecture — took the time to speak with Amazon Science about the rise of the machine learning industry, its implications for both tech and AI research, and the path forward for AI.</p>\n<p>The participants in the conversation were <ins><a href=\\"https://www.amazon.science/artificial-intelligence-the-revolution-hasnt-happened-yet\\" target=\\"_blank\\">Michael I. Jordan</a></ins>, a Distinguished <ins><a href=\\"https://www.amazon.science/scholars\\" target=\\"_blank\\">Amazon Scholar</a></ins> and the Pehong Chen Distinguished Professor at the University of California, Berkeley; <ins><a href=\\"https://www.amazon.science/latest-news/bernhard-scholkopf-wins-german-ai-innovation-award\\" target=\\"_blank\\">Bernhard Schölkopf</a></ins>, an Amazon vice president and distinguished scientist and the director of the empirical-inference program at the Max Planck Institute for Intelligent Systems in Tübingen; and <ins><a href=\\"https://www.amazon.science/latest-news/3-questions-with-michael-kearns-designing-socially-aware-algorithms-and-models\\" target=\\"_blank\\">Michael Kearns</a></ins>, an Amazon Scholar and a professor in the Department of Computer and Information Science at the University of Pennsylvania.</p>\n<p><img src=\\"https://dev-media.amazoncloud.cn/787f49a9111842c3b7d2830259239a68_image.png\\" alt=\\"image.png\\" /></p>\n<p>Jordan, Schölkopf, Kearns</p>\n<p>Jordan argued that AI research should focus, not on the “imitation game” proposed by Alan Turing, but on the “complementarity game”.</p>\n<p>“I do not want autonomous, self-driving cars, just like I don’t want autonomous, self-flying planes,” Jordan said. “I want them federated and talking to each other and sending high-level information back and forth and making plans together. … It’s not just a car; it’s a whole transportation system that gets people and packages around the world and should be thought of at that level. Really, we’re building, like, a system that brings food into a city. We’re building the entire system; we’re not just bringing one piece of bread into the city autonomously, whatever that might mean.”</p>\n<p>“The goal is to federate; the goal is to build complementary systems that interact with each other, interact well with humans,” Jordan continued. “This style of thinking I see more in industry than I see in academia. In industry, you solve a problem, and you bring in people from all these different points of view, and you think through the problem and the consequences a little bit. Because if you build a product that fails on one of these dimensions, it’s not going to work. So you do see more of this dialogue there. And I think that’s another way to go, to get our industry-academic connections to fire up some of these challenges and to push each other on both sides.”</p>\n<p>When Schölkopf gave his Posner lecture in 2011, before the deep-learning revolution, he was already concerned with the question of how machine learning models can incorporate causal reasoning.</p>\n<h4><a id=\\"This_style_of_thinking_I_see_more_in_industry_than_I_see_in_academia_In_industry_you_solve_a_problem_and_you_bring_in_people_from_all_these_different_points_of_view_and_you_think_through_the_problem_and_the_consequences_17\\"></a><strong>This style of thinking I see more in industry than I see in academia. In industry, you solve a problem, and you bring in people from all these different points of view, and you think through the problem and the consequences.</strong></h4>\\n<p>Michael I. Jordan</p>\n<p>“Machine learning ultimately is based on statistical dependencies, and we usually don’t ask where they actually come from,” Schölkopf said. “If two quantities are statistically dependent, it means that either one of them causes the other one, or there’s something else that has caused both of them. And so in that sense, causality is a concept that describes the dependencies in the system on a more fundamental level that produces statistical dependencies on the surface. Oftentimes, it’s enough if we work at the surface and just learn from these dependencies. But basically, it turns out that it’s only enough as long as we’re in this setting where nothing changes. Once things start changing, it’s actually helpful to think about the causality.”</p>\n<p>In most current work on causal reasoning in machine learning, Schölkopf explained, models attempt to determine causal relationships between variables specified in advance — say, the <ins><a href=\\"\\" target=\\"_blank\\">prices of dairy products</a></ins> in a particular region. One fruitful path forward for causal-reasoning research, he argued, is models that learn, not only the causal relationships between variables but the variables themselves.</p>\n<p>“We have to develop this field of causal representation learning,” Schölkopf said. “How do we identify the useful variables in high-dimensional data? I think that’s going to be interesting because current representation learning is really mostly about just learning statistical representations, which are useful for prediction but maybe not much more.”</p>\n<p>Picking up from Jordan’s contention that AI researchers need to think more about the place of AI agents in a larger social ecosystem, Kearns discussed the role that the scientific community should play in the regulation of AI.</p>\n<h4><a id=\\"Until_we_get_regulation_that_is_more_in_the_language_of_algorithms_itself_I_think_the_gap_between_wellintentioned_regulations_and_actual_enforceability_will_remain_very_very_wide_31\\"></a><strong>Until we get regulation that is more in the language of algorithms itself, I think the gap between well-intentioned regulations and actual enforceability will remain very, very wide.</strong></h4>\\n<p>Michael Kearns</p>\n<p>“One slightly controversial opinion is that I really think algorithmic regulation needs to look much more algorithmic itself,” Kearns said. “At the end of the day, we’re essentially building artifacts that are out in the world, making decisions, and will make decisions or predictions on any input you give them. The whole point of algorithms and machine learning is that you don’t have to explicitly specify what you’re going to do in every single corner case. But the model will do something in every corner case. And until we get regulation that really is more in the language of algorithms itself, I think the gap between well-intentioned regulations and actual enforceability will remain very, very wide.”</p>\n<p>Although he added that “I’ll admit that I don’t know how we’ll close that gap,” Kearns did point to recent work on game theory as possibly pointing a way forward.</p>\n<p>“One framework that’s emerged in recent years for essentially enforcing fairness constraints in the training of the model is very explicitly game theoretic, in which you basically design your algorithm in a way that sets it up as a two-player game, where one of the players is a learner of the traditional variety who generally is just concerned with predictive accuracy, and the other player you can think of as a regulator, who is there to enforce the fairness constraints,” Kearns said. “One thing that’s interesting about that approach, though, is you could even imagine kind of ripping the regulator out of the code itself and actually having it be a literal regulator. So the same framework for algorithm design could be thought of as a crude model for what might actually be the real-world back-and-forth between, let’s say, a tech regulator whose goal is to enforce anti-discrimination laws in predictive models and the regulatees.”</p>\n<p>A handful of excerpts, however, give only the flavor of what was a wide-ranging and stimulating conversation. Please watch the video to learn more about these distinguished scientists’ thoughts on the past and future of their field.</p>\n<p>ABOUT THE AUTHOR</p>\n<h4><a id=\\"Larry_Hardestyhttpswwwamazonscienceauthorlarryhardesty_46\\"></a><strong><a href=\\"https://www.amazon.science/author/larry-hardesty\\" target=\\"_blank\\">Larry Hardesty</a></strong></h4>\n<p>Larry Hardesty is the editor of the Amazon Science blog. Previously, he was a senior editor at MIT Technology Review and the computer science writer at the MIT News Office.</p>\n"}