{"value":"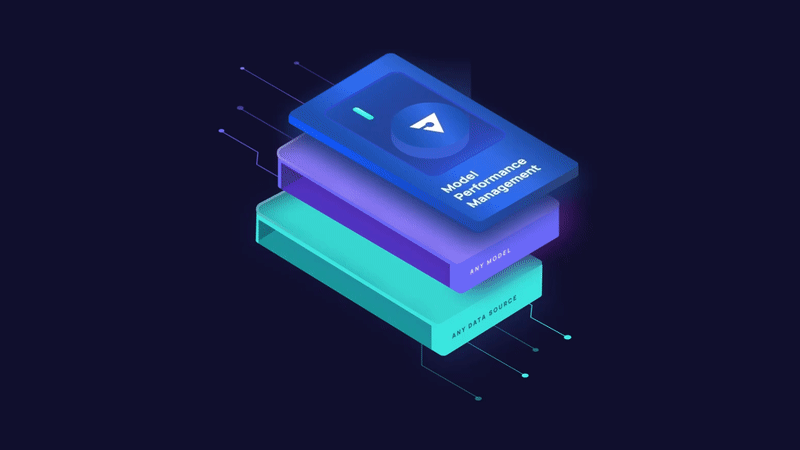\n\nFiddler's Model Performance Monitoring service is an all-in-one platform that allows customers to monitor, observe, explain, and analyze their AI systems.\n\nCREDIT: FIDDLER\n\nEditor’s Note: This interview is the latest installment ++[within a series Amazon Science is publishing](https://www.amazon.science/tag/alexa-fund)++ related to the science behind products and services from companies in which Amazon has invested. The Alexa Fund first invested in Fiddler.ai in August 2020, and then in June of this year participated in the company’s \$32 million funding round.\\n\\nGartner Group, the world’s leading research and advisory company, recently published its ++[top strategic technology trends for 2022](https://www.gartner.com/en/information-technology/insights/top-technology-trends)++. Among them is what Gartner terms “AI Engineering”, or the discipline of operationalizing updates to artificial intelligence models by “using integrated data and model and development pipelines to deliver consistent business value from AI,” and by combining “automated update pipelines with strong AI governance.”\\n\\nGartner analysts further stated that by 2025 “the 10% of enterprises that establish AI engineering best practices will generate at least three times more value from their AI efforts than the 90% of enterprises that do not.”\\n\\nThat report, and the surging interest in the topic of ++[explainable AI, or XAI](https://arxiv.org/search/?query=%22XAI%22&searchtype=all&source=header)++, is validation for Krishna Gade and his co-founders of Fiddler.ai, who started the company in 2018 with the belief that businesses needed a new kind of explainable AI service to address issues of fairness, accountability, transparency, and trust.\\n\\nThe idea behind the company’s formation emerged from Gade’s previous engineering manager role at Facebook, where he led a team that built tools to help the company’s developers find bugs, and make the company’s News Feed more transparent.\\n\\n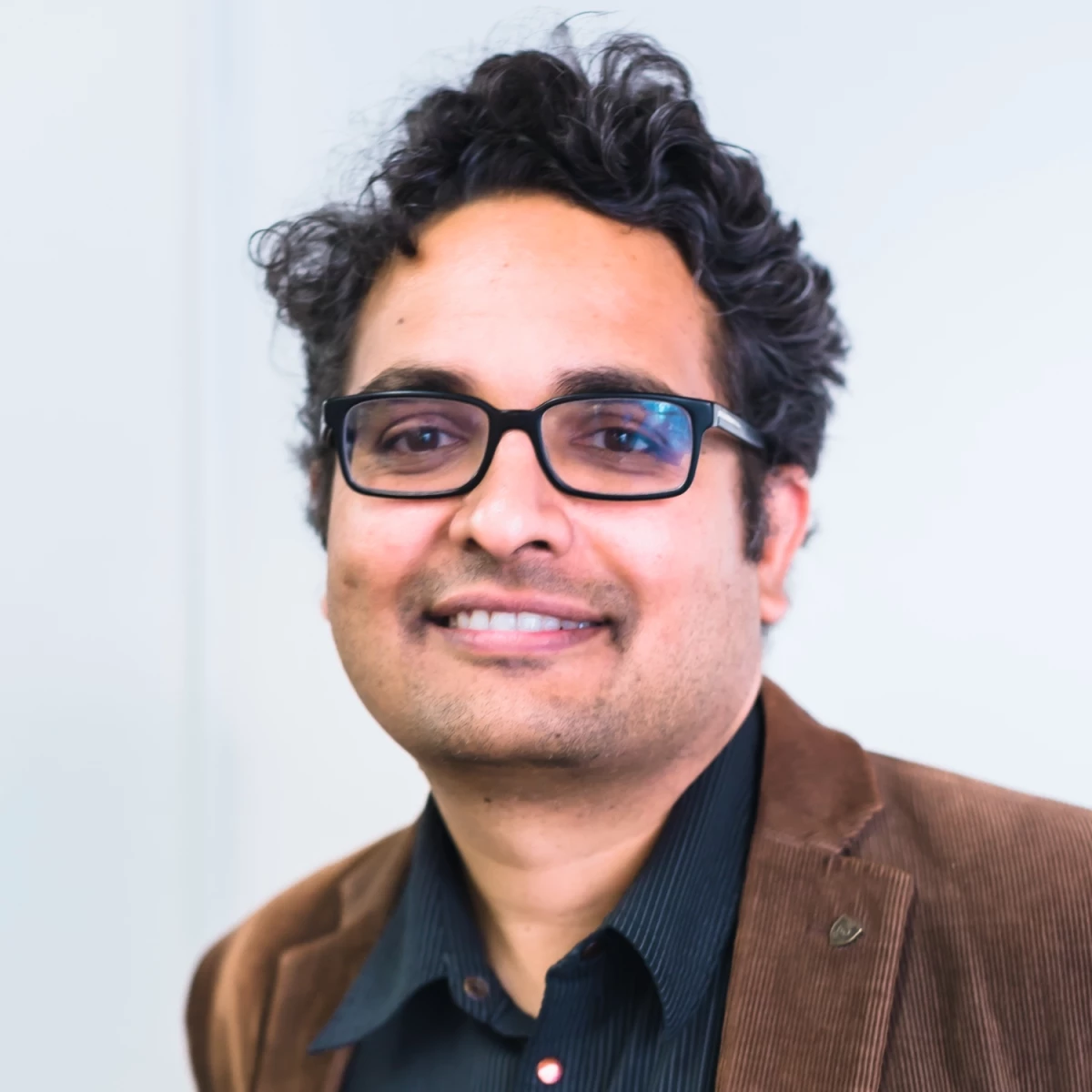\\n\\nKrishna Gade, a founder and CEO of Fiddler.ai.\\n\\nCREDIT: FIDDLER.AI\\n\\n“When I joined Facebook [in 2016], the problem we were addressing was one of having hundreds of models coming together to make decisions about how likely it would be for an individual to engage with the content, or how likely they would comment on a post, or share it. But it was very difficult to answer questions like ‘Why am I seeing this story?’ or ‘Why is this story going viral?’”.\\n\\nThat experience, Gade says, is what led him to form Fiddler.ai with his co-founders, Amit Paka and Manoj Cheenath.\\n\\n“I realized this wasn’t a problem that just Facebook had to solve, but that it was a very general machine learning workflow problem,” Gade adds. “Until that point, we had lots of tools focused on helping data scientists and machine learning engineers to build and deploy models, but people weren’t focused on what happened after the models went into production. How do you monitor them? How do you explain them? How do you know that you can continue to trust them? Our vision was to create a Tableau-like tool for machine learning that could unify the management of these ML models, instrument them, monitor them, and explain how they’re behaving to various stakeholders.”\\n\\nAmazon Science connected with Gade recently, and asked him three questions about AI’s “black box” problem, some of the biggest challenges and opportunities being addressed in the emerging field of explainable AI, and about his company’s machine learning model operations and monitoring solutions.\\n\\n\\n#### **Q. A quick search of XAI on arXiv produces a large body of research focusing on AI’s “black box” problem. How is Fiddler addressing this challenge, and how do you differentiate your approach from others?**\\n\\n\\nWith AI, you’re training a system; you’re feeding it large volumes of data, historical data, both good and bad. For example, let's say you're trying to use AI to classify fraud, or to figure out the credit risk of your customers, or which customers are likely to churn in the future.\\n\\n<video src=\\"https://dev-media.amazoncloud.cn/6972fe9f69ba4e70a4986499ae934f3d_Rec%200009.mp4\\" class=\\"manvaVedio\\" controls=\\"controls\\" style=\\"width:160px;height:160px\\"></video>\\n\\n**Fiddler.ai CEO Krishna Gade talks explainable AI**\\n\\nIn this process you’re feeding the system this data and you're building a system that encodes patterns in the data into some sort of a structure. That structure is called the model architecture. It could be a neural network, a decision tree or a random forest; there are so many different model architectures that are available.\\n\\nYou then use this structure to attempt to predict the future. The problem with this approach is that these structures are artifacts that become more and more complex over time. Twenty years ago when financial services companies were assessing credit risk, they were building mostly linear models where you could see the weights of the equation and actually read and interpret them.\\n\\nWhereas today’s machine learning and deep learning models are not human interpretable (sometimes simply because of their complexity) in the sense that you cannot understand how the structure is coming together to arrive at its prediction. This is where explainability becomes important because now you've got a black box system that could actually be highly accurate but is not human-readable. Without human understanding of how the model works, there is no way to fully trust the results which should make stakeholders uneasy. This is where explainability is adding business value to companies so that they can bridge this human-machine trust gap.\\n\\n\\n#### **Without human understanding of how the model works, there is no way to fully trust the results which should make stakeholders uneasy.**\\n\\n\\nKrishna Gade\\n\\nWe’ve devised our explainable AI user experience to cater to different model types to ensure explanations allow for the various factors that go into making predictions. Perhaps you have a credit underwriting model that is predicting the risk of a particular loan. These types of models typically are ingesting attributes like the amount of the loan request, the income of the person that's requesting the loan, their FICO score, tenure of employment, and many other inputs.\\n\\nThese attributes go into the model as inputs and the model outputs a probability of how risky you are for approving this loan. The model could be any type, it could be a traditional machine learning model, or a deep learning model. We visualize explanations in context of the inputs so a data scientist can understand which predictive features have the most impact on results.\\n\\nWe provide ways for you to understand that this particular loan risk probability is, for example, 30 percent, and here are the reasons why — these inputs are contributing positively by this magnitude, these inputs are contributing negatively by this magnitude. It is like a detective plot figuring out root-cause, and the practitioner can interactively fiddle with the value and weighting of inputs — hence the name Fiddler.\\n\\nSo you can ask questions like ‘Okay, the loan risk probability right now is 30% because the customer is asking for \$10,000 loan. What if the customer asked for an \$8,000 loan? Would the loan risk go down? What if the customer was making \$10,000 more in income? Or what if the customer’s FICO score was 10 points higher’? You can ask these counterfactual questions by fiddling with inputs and you'll get real-time explanations in an interactive manner so you can understand not only why the model is making its predictions, but also what would happen if the person requesting the loan had a different profile. You can actually provide the human in the loop with decision support.\n\nWe provide a pluggable service which is differentiated from other monolithic, rigid products. Our customers can develop their AI systems however they want. They can build their own, use third-party, or open-source solutions. Or they can bring their models together with ours, which is what we call BYOM, or bring your own model, and we’ll help them explain it. We then visualize these explanations in various ways so they can show it not only to the technical people who built the models, but also to business stakeholders, or regulatory compliance stakeholders.\n\n\n#### **Q. What do you consider to be some of the biggest opportunities and challenges being addressed within the field of explainable AI today?**\n\n\nSo today there are four problems that are introduced when you put machine learning models into production.\n\nOne is the black box aspect that I talked about earlier. Most models are becoming increasingly complex. It is hard to know how they work and that creates a mistrust in how to use it and how to assure customers your AI solutions are fair.\n\nNumber two is model performance in terms of accuracy, fairness, and data quality. Unlike traditional software performance, model building is not static. Traditional software will behave the same way whenever you interact with it. But machine learning model performance can go up and down. This is called model drift. Teams who developed these models realized this more acutely during the pandemic, finding that they had trained their models on the pre-pandemic data, and now the pandemic had completely changed user behavior.\n\nOn an e-commerce site, for example, customers were asking for different types of things, toilet paper being one of those early examples. We had all kinds of varying factors — people losing jobs, working from home, and the lack of travel — any one of which would impact pricing algorithms for the airlines.\n\n\n#### **Most models are becoming increasingly complex. It is hard to know how they work and that creates a mistrust in how to use it and how to assure customers your AI solutions are fair. **\n\n\nKrishna Gade\n\nModel drift has always been there, but the pandemic showed us how much impact drift can have. This dramatic, mass-drift event is an opportunity for businesses that realize they not only need monitoring at the high level of business metrics, but they also need monitoring at the model level because it is too late to recover by the time issues show up in the business metrics. Having early warning systems for how your AI product is behaving has become essential for agility — identifying when and how model drift is happening has become table-stakes.\n\nThird is bias. As you know, some of these models have a direct impact on customers’ lives. For example, getting a loan approved or not, getting a job, getting a clinical diagnosis. Any of these events can change a person’s life, so a model going wrong, and going wrong in a big way for a certain sector of society, be it demographic, ethnicity, or gender or other factors can be really harmful to people. And that can seriously damage a company’s reputation and customer trust.\n\nWe’ve seen examples where a new credit card is launched and customers complain about gender discrimination where husbands and wives are getting 10x differences in credit limits, even though they have similar incomes and FICO scores. And when customers complain, customer support representatives might say ‘Oh, it’s just the algorithm, we don’t know how it works.’ We can’t abdicate our responsibility to an algorithm. Detecting bias earlier in the lifecycle of models and continuously monitoring for bias is super critical in many industries and high-stakes use cases.\n\nThe fourth aspect is governance and compliance. There is a lot of news these days about AI and the need for regulation. There is likely regulation coming, or in certain countries it already has come. Businesses now have to focus on how to make their models compliant. For example, regulation is top of mind in some sectors like financial services where there already are well defined regulations for how to build compliant models.\n\nThese are the four factors creating an opportunity for Fiddler to help our customers address these challenges, and they’re all linked by a common goal to build trust, both for those building the models, and for customers knowing they can believe in the integrity of our customers’ products.\n\n\n#### **Q. Fiddler provides machine learning operations and monitoring solutions. Can you explain some of the science behind these solutions, and how customers are utilizing them to accelerate model deployment?**\n\n\nThere are two main use cases for which customers turn to Fiddler. The first is pre-production model validation. So even before customers put the model into production, they need to understand how it is working: from an explainability standpoint, from a bias perspective, from understanding data imbalance issues, and so on.\n\nFiddler offers its customers many insights that can help them understand more about how the model they've created is going to work. For example, customers in the banking sector may use Fiddler for model validation to understand the risks of those models even before they’re deployed.\n\nThe second use case is post-production model monitoring. So now a business deploys a model into production – how is that model behaving? With Fiddler, users can set up alerts for when things go wrong so their machine learning engineers or data scientists can diagnose what’s happening.\n\nLet’s say there’s model drift, or there are data-quality issues coming into your pipelines, and the accuracy of your model is going down. You can now figure out what's going on and then fix those issues. Any business or team that is deploying machine learning models needs to understand what is going on.\n\n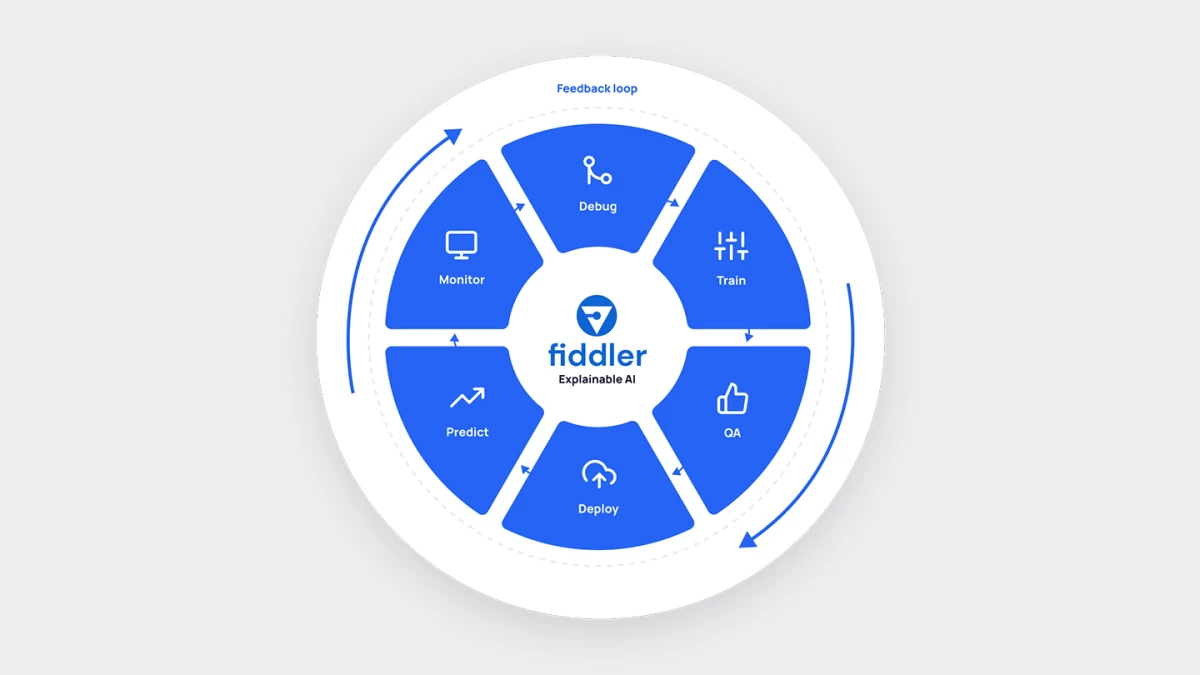\n\nFiddler CEO Krishna Gade says there are two main reasons customers turn to Fiddler: The first is pre-production model validation, the second is post-production model monitoring.\n\nCREDIT: FIDDLER\n\nWe are seeing traction, in particular, within a couple of sectors. One is digital-native companies that need to quickly deploy models and proactively monitor models. They need to observe how their models are performing in production, and how they're affecting their business metrics.\n\nWhen it comes to financial services it’s interesting because they have experienced increased regulation, particularly since 2008. Even before they were starting to use machine learning models, they were building handcrafted quantitative models. In 2008 we had the economic crisis, bank bail outs, and the Fed institutionalized the SR 11-7 regulation, which mandated risk management of every bank model with stricter requirements for high-risk models like credit risk. So model risk management is a process that every bank in the United States, Europe and elsewhere must follow.\n\nToday, the quantitative models that banks use are being replaced or complemented by machine learning models due to the availability of a lot more data, specialized talent, and the tools to build more machine learning and deep learning models. Unfortunately, the governance approaches used to minimize risk and validate models in the past are no longer applicable for today’s more sophisticated and complex models.\n\nThe whole pre-production model validation — understanding all the risks around models — and then post production model monitoring, which combined is called model risk management, is leading banks to look to Fiddler and others to help them address these challenges.\n\nAll of this comes together with our model management platform (MPM); it is a unified platform that provides a common language, metrics, and centralized controls that are required for operationalizing ML/AI with trust.\n\nOur pluggable service allows our customers to bring a variety of models. They can be trained on structured data sets or unstructured data sets, tabular data or text or image data, and they can be visualized for both technical and non-technical people at scale. Our customers can run their models wherever they want. They can use our managed cloud service, but they can also run it within their own environments, whether that’s a data center or their favorite cloud provider of choice. So the plugability of our solution, and the fact that we’re cloud and model agnostic is what differentiates our product.\n\nABOUT THE AUTHOR\n\n#### **Staff writer**\n\n","render":"<p><img src=\\"https://dev-media.amazoncloud.cn/c2bb277286e849eb88f1487bcacae743_111.gif\\" alt=\\"111.gif\\" /></p>\n<p>Fiddler’s Model Performance Monitoring service is an all-in-one platform that allows customers to monitor, observe, explain, and analyze their AI systems.</p>\n<p>CREDIT: FIDDLER</p>\n<p>Editor’s Note: This interview is the latest installment <ins><a href=\\"https://www.amazon.science/tag/alexa-fund\\" target=\\"_blank\\">within a series Amazon Science is publishing</a></ins> related to the science behind products and services from companies in which Amazon has invested. The Alexa Fund first invested in Fiddler.ai in August 2020, and then in June of this year participated in the company’s \$32 million funding round.</p>\n<p>Gartner Group, the world’s leading research and advisory company, recently published its <ins><a href=\\"https://www.gartner.com/en/information-technology/insights/top-technology-trends\\" target=\\"_blank\\">top strategic technology trends for 2022</a></ins>. Among them is what Gartner terms “AI Engineering”, or the discipline of operationalizing updates to artificial intelligence models by “using integrated data and model and development pipelines to deliver consistent business value from AI,” and by combining “automated update pipelines with strong AI governance.”</p>\n<p>Gartner analysts further stated that by 2025 “the 10% of enterprises that establish AI engineering best practices will generate at least three times more value from their AI efforts than the 90% of enterprises that do not.”</p>\n<p>That report, and the surging interest in the topic of <ins><a href=\\"https://arxiv.org/search/?query=%22XAI%22&searchtype=all&source=header\\" target=\\"_blank\\">explainable AI, or XAI</a></ins>, is validation for Krishna Gade and his co-founders of Fiddler.ai, who started the company in 2018 with the belief that businesses needed a new kind of explainable AI service to address issues of fairness, accountability, transparency, and trust.</p>\n<p>The idea behind the company’s formation emerged from Gade’s previous engineering manager role at Facebook, where he led a team that built tools to help the company’s developers find bugs, and make the company’s News Feed more transparent.</p>\n<p><img src=\\"https://dev-media.amazoncloud.cn/94b1aac8559c4f51a63f86becdb333ae_image.png\\" alt=\\"image.png\\" /></p>\n<p>Krishna Gade, a founder and CEO of Fiddler.ai.</p>\n<p>CREDIT: FIDDLER.AI</p>\n<p>“When I joined Facebook [in 2016], the problem we were addressing was one of having hundreds of models coming together to make decisions about how likely it would be for an individual to engage with the content, or how likely they would comment on a post, or share it. But it was very difficult to answer questions like ‘Why am I seeing this story?’ or ‘Why is this story going viral?’”.</p>\n<p>That experience, Gade says, is what led him to form Fiddler.ai with his co-founders, Amit Paka and Manoj Cheenath.</p>\n<p>“I realized this wasn’t a problem that just Facebook had to solve, but that it was a very general machine learning workflow problem,” Gade adds. “Until that point, we had lots of tools focused on helping data scientists and machine learning engineers to build and deploy models, but people weren’t focused on what happened after the models went into production. How do you monitor them? How do you explain them? How do you know that you can continue to trust them? Our vision was to create a Tableau-like tool for machine learning that could unify the management of these ML models, instrument them, monitor them, and explain how they’re behaving to various stakeholders.”</p>\n<p>Amazon Science connected with Gade recently, and asked him three questions about AI’s “black box” problem, some of the biggest challenges and opportunities being addressed in the emerging field of explainable AI, and about his company’s machine learning model operations and monitoring solutions.</p>\n<h4><a id=\\"Q_A_quick_search_of_XAI_on_arXiv_produces_a_large_body_of_research_focusing_on_AIs_black_box_problem_How_is_Fiddler_addressing_this_challenge_and_how_do_you_differentiate_your_approach_from_others_31\\"></a><strong>Q. A quick search of XAI on arXiv produces a large body of research focusing on AI’s “black box” problem. How is Fiddler addressing this challenge, and how do you differentiate your approach from others?</strong></h4>\\n<p>With AI, you’re training a system; you’re feeding it large volumes of data, historical data, both good and bad. For example, let’s say you’re trying to use AI to classify fraud, or to figure out the credit risk of your customers, or which customers are likely to churn in the future.</p>\n<p><video src=\\"https://dev-media.amazoncloud.cn/6972fe9f69ba4e70a4986499ae934f3d_Rec%200009.mp4\\" controls=\\"controls\\"></video></p>\\n<p><strong>Fiddler.ai CEO Krishna Gade talks explainable AI</strong></p>\\n<p>In this process you’re feeding the system this data and you’re building a system that encodes patterns in the data into some sort of a structure. That structure is called the model architecture. It could be a neural network, a decision tree or a random forest; there are so many different model architectures that are available.</p>\n<p>You then use this structure to attempt to predict the future. The problem with this approach is that these structures are artifacts that become more and more complex over time. Twenty years ago when financial services companies were assessing credit risk, they were building mostly linear models where you could see the weights of the equation and actually read and interpret them.</p>\n<p>Whereas today’s machine learning and deep learning models are not human interpretable (sometimes simply because of their complexity) in the sense that you cannot understand how the structure is coming together to arrive at its prediction. This is where explainability becomes important because now you’ve got a black box system that could actually be highly accurate but is not human-readable. Without human understanding of how the model works, there is no way to fully trust the results which should make stakeholders uneasy. This is where explainability is adding business value to companies so that they can bridge this human-machine trust gap.</p>\n<h4><a id=\\"Without_human_understanding_of_how_the_model_works_there_is_no_way_to_fully_trust_the_results_which_should_make_stakeholders_uneasy_47\\"></a><strong>Without human understanding of how the model works, there is no way to fully trust the results which should make stakeholders uneasy.</strong></h4>\\n<p>Krishna Gade</p>\n<p>We’ve devised our explainable AI user experience to cater to different model types to ensure explanations allow for the various factors that go into making predictions. Perhaps you have a credit underwriting model that is predicting the risk of a particular loan. These types of models typically are ingesting attributes like the amount of the loan request, the income of the person that’s requesting the loan, their FICO score, tenure of employment, and many other inputs.</p>\n<p>These attributes go into the model as inputs and the model outputs a probability of how risky you are for approving this loan. The model could be any type, it could be a traditional machine learning model, or a deep learning model. We visualize explanations in context of the inputs so a data scientist can understand which predictive features have the most impact on results.</p>\n<p>We provide ways for you to understand that this particular loan risk probability is, for example, 30 percent, and here are the reasons why — these inputs are contributing positively by this magnitude, these inputs are contributing negatively by this magnitude. It is like a detective plot figuring out root-cause, and the practitioner can interactively fiddle with the value and weighting of inputs — hence the name Fiddler.</p>\n<p>So you can ask questions like ‘Okay, the loan risk probability right now is 30% because the customer is asking for \$10,000 loan. What if the customer asked for an \$8,000 loan? Would the loan risk go down? What if the customer was making \$10,000 more in income? Or what if the customer’s FICO score was 10 points higher’? You can ask these counterfactual questions by fiddling with inputs and you’ll get real-time explanations in an interactive manner so you can understand not only why the model is making its predictions, but also what would happen if the person requesting the loan had a different profile. You can actually provide the human in the loop with decision support.</p>\n<p>We provide a pluggable service which is differentiated from other monolithic, rigid products. Our customers can develop their AI systems however they want. They can build their own, use third-party, or open-source solutions. Or they can bring their models together with ours, which is what we call BYOM, or bring your own model, and we’ll help them explain it. We then visualize these explanations in various ways so they can show it not only to the technical people who built the models, but also to business stakeholders, or regulatory compliance stakeholders.</p>\n<h4><a id=\\"Q_What_do_you_consider_to_be_some_of_the_biggest_opportunities_and_challenges_being_addressed_within_the_field_of_explainable_AI_today_63\\"></a><strong>Q. What do you consider to be some of the biggest opportunities and challenges being addressed within the field of explainable AI today?</strong></h4>\\n<p>So today there are four problems that are introduced when you put machine learning models into production.</p>\n<p>One is the black box aspect that I talked about earlier. Most models are becoming increasingly complex. It is hard to know how they work and that creates a mistrust in how to use it and how to assure customers your AI solutions are fair.</p>\n<p>Number two is model performance in terms of accuracy, fairness, and data quality. Unlike traditional software performance, model building is not static. Traditional software will behave the same way whenever you interact with it. But machine learning model performance can go up and down. This is called model drift. Teams who developed these models realized this more acutely during the pandemic, finding that they had trained their models on the pre-pandemic data, and now the pandemic had completely changed user behavior.</p>\n<p>On an e-commerce site, for example, customers were asking for different types of things, toilet paper being one of those early examples. We had all kinds of varying factors — people losing jobs, working from home, and the lack of travel — any one of which would impact pricing algorithms for the airlines.</p>\n<h4><a id=\\"Most_models_are_becoming_increasingly_complex_It_is_hard_to_know_how_they_work_and_that_creates_a_mistrust_in_how_to_use_it_and_how_to_assure_customers_your_AI_solutions_are_fair__75\\"></a>**Most models are becoming increasingly complex. It is hard to know how they work and that creates a mistrust in how to use it and how to assure customers your AI solutions are fair. **</h4>\\n<p>Krishna Gade</p>\n<p>Model drift has always been there, but the pandemic showed us how much impact drift can have. This dramatic, mass-drift event is an opportunity for businesses that realize they not only need monitoring at the high level of business metrics, but they also need monitoring at the model level because it is too late to recover by the time issues show up in the business metrics. Having early warning systems for how your AI product is behaving has become essential for agility — identifying when and how model drift is happening has become table-stakes.</p>\n<p>Third is bias. As you know, some of these models have a direct impact on customers’ lives. For example, getting a loan approved or not, getting a job, getting a clinical diagnosis. Any of these events can change a person’s life, so a model going wrong, and going wrong in a big way for a certain sector of society, be it demographic, ethnicity, or gender or other factors can be really harmful to people. And that can seriously damage a company’s reputation and customer trust.</p>\n<p>We’ve seen examples where a new credit card is launched and customers complain about gender discrimination where husbands and wives are getting 10x differences in credit limits, even though they have similar incomes and FICO scores. And when customers complain, customer support representatives might say ‘Oh, it’s just the algorithm, we don’t know how it works.’ We can’t abdicate our responsibility to an algorithm. Detecting bias earlier in the lifecycle of models and continuously monitoring for bias is super critical in many industries and high-stakes use cases.</p>\n<p>The fourth aspect is governance and compliance. There is a lot of news these days about AI and the need for regulation. There is likely regulation coming, or in certain countries it already has come. Businesses now have to focus on how to make their models compliant. For example, regulation is top of mind in some sectors like financial services where there already are well defined regulations for how to build compliant models.</p>\n<p>These are the four factors creating an opportunity for Fiddler to help our customers address these challenges, and they’re all linked by a common goal to build trust, both for those building the models, and for customers knowing they can believe in the integrity of our customers’ products.</p>\n<h4><a id=\\"Q_Fiddler_provides_machine_learning_operations_and_monitoring_solutions_Can_you_explain_some_of_the_science_behind_these_solutions_and_how_customers_are_utilizing_them_to_accelerate_model_deployment_91\\"></a><strong>Q. Fiddler provides machine learning operations and monitoring solutions. Can you explain some of the science behind these solutions, and how customers are utilizing them to accelerate model deployment?</strong></h4>\\n<p>There are two main use cases for which customers turn to Fiddler. The first is pre-production model validation. So even before customers put the model into production, they need to understand how it is working: from an explainability standpoint, from a bias perspective, from understanding data imbalance issues, and so on.</p>\n<p>Fiddler offers its customers many insights that can help them understand more about how the model they’ve created is going to work. For example, customers in the banking sector may use Fiddler for model validation to understand the risks of those models even before they’re deployed.</p>\n<p>The second use case is post-production model monitoring. So now a business deploys a model into production – how is that model behaving? With Fiddler, users can set up alerts for when things go wrong so their machine learning engineers or data scientists can diagnose what’s happening.</p>\n<p>Let’s say there’s model drift, or there are data-quality issues coming into your pipelines, and the accuracy of your model is going down. You can now figure out what’s going on and then fix those issues. Any business or team that is deploying machine learning models needs to understand what is going on.</p>\n<p><img src=\\"https://dev-media.amazoncloud.cn/84a890b03d594001b7aa3e3eba1a12ad_image.png\\" alt=\\"image.png\\" /></p>\n<p>Fiddler CEO Krishna Gade says there are two main reasons customers turn to Fiddler: The first is pre-production model validation, the second is post-production model monitoring.</p>\n<p>CREDIT: FIDDLER</p>\n<p>We are seeing traction, in particular, within a couple of sectors. One is digital-native companies that need to quickly deploy models and proactively monitor models. They need to observe how their models are performing in production, and how they’re affecting their business metrics.</p>\n<p>When it comes to financial services it’s interesting because they have experienced increased regulation, particularly since 2008. Even before they were starting to use machine learning models, they were building handcrafted quantitative models. In 2008 we had the economic crisis, bank bail outs, and the Fed institutionalized the SR 11-7 regulation, which mandated risk management of every bank model with stricter requirements for high-risk models like credit risk. So model risk management is a process that every bank in the United States, Europe and elsewhere must follow.</p>\n<p>Today, the quantitative models that banks use are being replaced or complemented by machine learning models due to the availability of a lot more data, specialized talent, and the tools to build more machine learning and deep learning models. Unfortunately, the governance approaches used to minimize risk and validate models in the past are no longer applicable for today’s more sophisticated and complex models.</p>\n<p>The whole pre-production model validation — understanding all the risks around models — and then post production model monitoring, which combined is called model risk management, is leading banks to look to Fiddler and others to help them address these challenges.</p>\n<p>All of this comes together with our model management platform (MPM); it is a unified platform that provides a common language, metrics, and centralized controls that are required for operationalizing ML/AI with trust.</p>\n<p>Our pluggable service allows our customers to bring a variety of models. They can be trained on structured data sets or unstructured data sets, tabular data or text or image data, and they can be visualized for both technical and non-technical people at scale. Our customers can run their models wherever they want. They can use our managed cloud service, but they can also run it within their own environments, whether that’s a data center or their favorite cloud provider of choice. So the plugability of our solution, and the fact that we’re cloud and model agnostic is what differentiates our product.</p>\n<p>ABOUT THE AUTHOR</p>\n<h4><a id=\\"Staff_writer_122\\"></a><strong>Staff writer</strong></h4>\n"}