{"value":"We are excited to announce that [Amazon Prime Air](https://www.amazon.com/Amazon-Prime-Air/b?ie=UTF8&node=8037720011), in collaboration with Amazon Web Services (AWS), is hosting the [First Workshop on Airborne Object Tracking](https://zontakm9.github.io/aot-iccvw21/) (AOT) at the [International Conference on Computer Vision ](https://www.amazon.science/conferences-and-events/iccv-2021)(ICCV) 2021. The workshop is organized around a new challenge based on a [large and unique dataset](https://www.aicrowd.com/challenges/airborne-object-tracking-challenge#dataset) that Amazon Prime Air has released — the Airborne-Object Tracking Dataset, which benchmarks airborne-object detection and tracking to increase the safety of the airspace.\n\n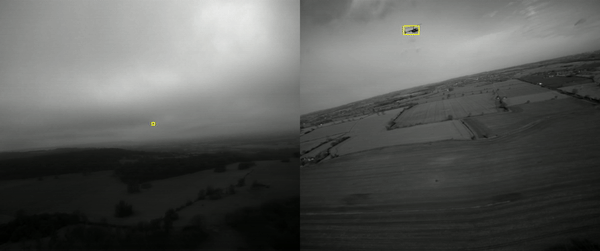\n\nSample data from the Airborne Object Tracking dataset. The yellow bounding boxes around airborne objects are the ground truth for learning and testing.\n\n[The challenge](https://www.aicrowd.com/challenges/airborne-object-tracking-challenge), which launched in April, is to track airborne objects across successive frames of video, with the ultimate goal of obstacle avoidance.\n\nThe top six finishers in the challenge will give short talks during the workshop. Amazon Prime Air has also sponsored $50,000 in prizes for challenge participants, including $15,000 to the first-place finisher and a $2,500 prize for the “most creative” safety solution, as determined by the judges.\n\nThe workshop, which will be held virtually on October 11, 2021, will also feature talks by invited speakers, including Amazon researchers (Amir Navot) and academics (Laura Leal-Taixé from TU Munich, Pascal Fua from EPFL, and Andreas Geiger from the Tübingen Institute).\n\n#### **Sense and avoid**\n\nIn recent years, applications of drones have grown to include infrastructure inspection, emergency response support, agricultural and environmental surveys, and package delivery, among others. Against this backdrop, the safe operation of drones requires fully autonomous and robust sense-and-avoid systems. By sharing our dataset, defining the problem and evaluation criteria, and hosting a challenge, we hope to expose the computer vision community to a relatively fresh area of autonomous flight applications, while emphasizing the real-world requirements for safe autonomous flight. \n\nTo generate the flight sequences in the AOT data set, two aircraft were equipped with sensors and flew planned encounters. Their trajectories were designed to create a wide distribution of distances, closing velocities, and approach angles. In addition to the so-called planned aircraft, AOT contains other unplanned airborne objects. The images have been manually annotated to indicate the locations of the airborne objects.\n\nAirborne objects usually appear quite small at the distances that are relevant for early detection: 0.01% of the image size on average, down to a few pixels in area. In total, AOT includes close to 164 hours of flight data: 4,943 flight sequences of around 120 seconds each, collected at 10 Hz in diverse conditions.\n\nParticipants in the challenge may choose to solve either or both of two challenges. One is encounter-level detection, which means successfully tracking an airborne object for three seconds — before the object is within 300 meters of the drone. The tracker must also have a false-alarm rate no higher than one false alarm every two hours.\n\nThe other challenge is frame-level object detection. The goal is to maximize the ratio between the number of the detected airborne objects, on a per-frame basis, and all the airborne objects that should be detected. For this challenge, there is a budget of false identifications for each frame.\n\nThe workshop’s organizers envision this workshop as the first in a series of annual workshops, which will both catalyze and publicize research on autonomous flight — ultimately leading to even safer skies for drones. We invite you to challenge the baseline and submit your results and papers by July 15.\n\n#### **Important dates**\n\n[Revised 7/13/21]\n\n- **~~July 15~~ August 2**: ~~Final submissions deadline and~~ Paper submission deadline\n- **July 20**: Posting of private leaderboard \n- **~~July 25**: Announcement of winners ~~\n- **August 5 10**: Notification to authors \n- **August ~~15~~ 17**: Camera-ready-papers deadline\n- **September 1**: Challenge submission deadline\n\nABOUT THE AUTHOR\n#### **[Maria Zontak](https://www.amazon.science/author/maria-zontak)**\nMaria Zontak is an applied scientist with Amazon Web Services.\n#### **[Yuri Federigi](https://www.amazon.science/author/yuri-federigi)**\nYuri Federigi is a program manager with Amazon Prime Air.","render":"<p>We are excited to announce that <a href=\"https://www.amazon.com/Amazon-Prime-Air/b?ie=UTF8&node=8037720011\" target=\"_blank\">Amazon Prime Air</a>, in collaboration with Amazon Web Services (AWS), is hosting the <a href=\"https://zontakm9.github.io/aot-iccvw21/\" target=\"_blank\">First Workshop on Airborne Object Tracking</a> (AOT) at the <a href=\"https://www.amazon.science/conferences-and-events/iccv-2021\" target=\"_blank\">International Conference on Computer Vision </a>(ICCV) 2021. The workshop is organized around a new challenge based on a <a href=\"https://www.aicrowd.com/challenges/airborne-object-tracking-challenge#dataset\" target=\"_blank\">large and unique dataset</a> that Amazon Prime Air has released — the Airborne-Object Tracking Dataset, which benchmarks airborne-object detection and tracking to increase the safety of the airspace.</p>\n<p><img src=\"https://dev-media.amazoncloud.cn/7752add724b34f799c01b30b5a261b78_%E4%B8%8B%E8%BD%BD.gif\" alt=\"下载.gif\" /></p>\n<p>Sample data from the Airborne Object Tracking dataset. The yellow bounding boxes around airborne objects are the ground truth for learning and testing.</p>\n<p><a href=\"https://www.aicrowd.com/challenges/airborne-object-tracking-challenge\" target=\"_blank\">The challenge</a>, which launched in April, is to track airborne objects across successive frames of video, with the ultimate goal of obstacle avoidance.</p>\n<p>The top six finishers in the challenge will give short talks during the workshop. Amazon Prime Air has also sponsored $50,000 in prizes for challenge participants, including $15,000 to the first-place finisher and a $2,500 prize for the “most creative” safety solution, as determined by the judges.</p>\n<p>The workshop, which will be held virtually on October 11, 2021, will also feature talks by invited speakers, including Amazon researchers (Amir Navot) and academics (Laura Leal-Taixé from TU Munich, Pascal Fua from EPFL, and Andreas Geiger from the Tübingen Institute).</p>\n<h4><a id=\"Sense_and_avoid_12\"></a><strong>Sense and avoid</strong></h4>\n<p>In recent years, applications of drones have grown to include infrastructure inspection, emergency response support, agricultural and environmental surveys, and package delivery, among others. Against this backdrop, the safe operation of drones requires fully autonomous and robust sense-and-avoid systems. By sharing our dataset, defining the problem and evaluation criteria, and hosting a challenge, we hope to expose the computer vision community to a relatively fresh area of autonomous flight applications, while emphasizing the real-world requirements for safe autonomous flight.</p>\n<p>To generate the flight sequences in the AOT data set, two aircraft were equipped with sensors and flew planned encounters. Their trajectories were designed to create a wide distribution of distances, closing velocities, and approach angles. In addition to the so-called planned aircraft, AOT contains other unplanned airborne objects. The images have been manually annotated to indicate the locations of the airborne objects.</p>\n<p>Airborne objects usually appear quite small at the distances that are relevant for early detection: 0.01% of the image size on average, down to a few pixels in area. In total, AOT includes close to 164 hours of flight data: 4,943 flight sequences of around 120 seconds each, collected at 10 Hz in diverse conditions.</p>\n<p>Participants in the challenge may choose to solve either or both of two challenges. One is encounter-level detection, which means successfully tracking an airborne object for three seconds — before the object is within 300 meters of the drone. The tracker must also have a false-alarm rate no higher than one false alarm every two hours.</p>\n<p>The other challenge is frame-level object detection. The goal is to maximize the ratio between the number of the detected airborne objects, on a per-frame basis, and all the airborne objects that should be detected. For this challenge, there is a budget of false identifications for each frame.</p>\n<p>The workshop’s organizers envision this workshop as the first in a series of annual workshops, which will both catalyze and publicize research on autonomous flight — ultimately leading to even safer skies for drones. We invite you to challenge the baseline and submit your results and papers by July 15.</p>\n<h4><a id=\"Important_dates_26\"></a><strong>Important dates</strong></h4>\n<p>[Revised 7/13/21]</p>\n<ul>\n<li><strong><s>July 15</s> August 2</strong>: <s>Final submissions deadline and</s> Paper submission deadline</li>\n<li><strong>July 20</strong>: Posting of private leaderboard</li>\n<li><strong>~~July 25</strong>: Announcement of winners ~~</li>\n<li><strong>August 5 10</strong>: Notification to authors</li>\n<li><strong>August <s>15</s> 17</strong>: Camera-ready-papers deadline</li>\n<li><strong>September 1</strong>: Challenge submission deadline</li>\n</ul>\n<p>ABOUT THE AUTHOR</p>\n<h4><a id=\"Maria_Zontakhttpswwwamazonscienceauthormariazontak_38\"></a><strong><a href=\"https://www.amazon.science/author/maria-zontak\" target=\"_blank\">Maria Zontak</a></strong></h4>\n<p>Maria Zontak is an applied scientist with Amazon Web Services.</p>\n<h4><a id=\"Yuri_Federigihttpswwwamazonscienceauthoryurifederigi_40\"></a><strong><a href=\"https://www.amazon.science/author/yuri-federigi\" target=\"_blank\">Yuri Federigi</a></strong></h4>\n<p>Yuri Federigi is a program manager with Amazon Prime Air.</p>\n"}