{"value":"Alexa launched in 2014, and in the more than six years since, we’ve been making good on our promise to make Alexa smarter every day. In addition to foundational improvements in Alexa’s core AI technologies, such as [speech recognition](https://www.amazon.science/tag/asr) and [natural-language-understanding](https://www.amazon.science/tag/nlu) systems, Alexa scientists have developed technologies that continue to delight our customers, such as [whispered speech](https://www.amazon.science/blog/whisper-to-alexa-and-shell-whisper-back) and Alexa’s new [live translation](https://www.amazon.science/blog/how-alexas-new-live-translation-for-conversations-works) service.\n\n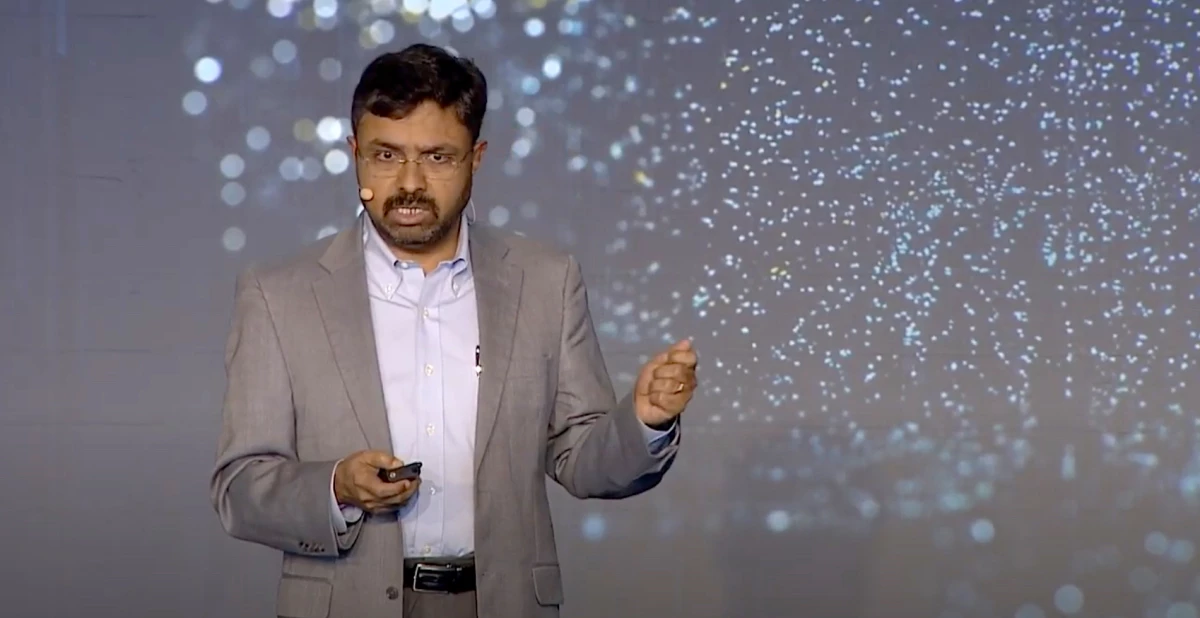\n\nPrem Natarajan, Alexa AI vice president of natural understanding, at a conference in 2018.\n\nBut some of the technologies we’ve begun to introduce, together with others we’re now investigating, are harbingers of a step change in Alexa’s development — and in the field of AI itself. Collectively, these technologies will bring a new level of generalizability and autonomy to both the Alexa voice service and the tools available to Alexa developers, ushering in what I like to think of as a new “age of self” in artificial intelligence, an age in which AI systems such as Alexa become more self-aware and more self-learning, and in which they lend themselves to self-service by experienced developers and even end users.\n\nBy self-awareness, I mean the ability to maintain an awareness of ambient state (e.g., time of day, thermostat readings, and recent actions) and to employ commonsense reasoning to make inferences that reflect that awareness and prior/world knowledge. Alexa [hunches](https://www.youtube.com/watch?v=whahElqS5eA) can already recognize anomalies in customers’ daily routines and suggest corrections — noticing that a light was left on at night and offering to turn it off, for instance. Powered by commonsense reasoning, self-awareness goes further: for instance, if a customer turns on the television five minutes before the kids’ soccer practice is scheduled to end, an AI of the future might infer that the customer needs a reminder about pickup.\n\nSelf-learning is Alexa’s ability to improve and expand its capabilities without human intervention. And like self-awareness, self-learning employs reasoning: for example, does the customer’s response to an action indicate dissatisfaction with that action? Similarly, when a customer issues an unfamiliar command, a truly self-learning Alexa would be able to infer what it might mean — perhaps by searching the web or exploring a knowledge base — and suggest possibilities.\n\n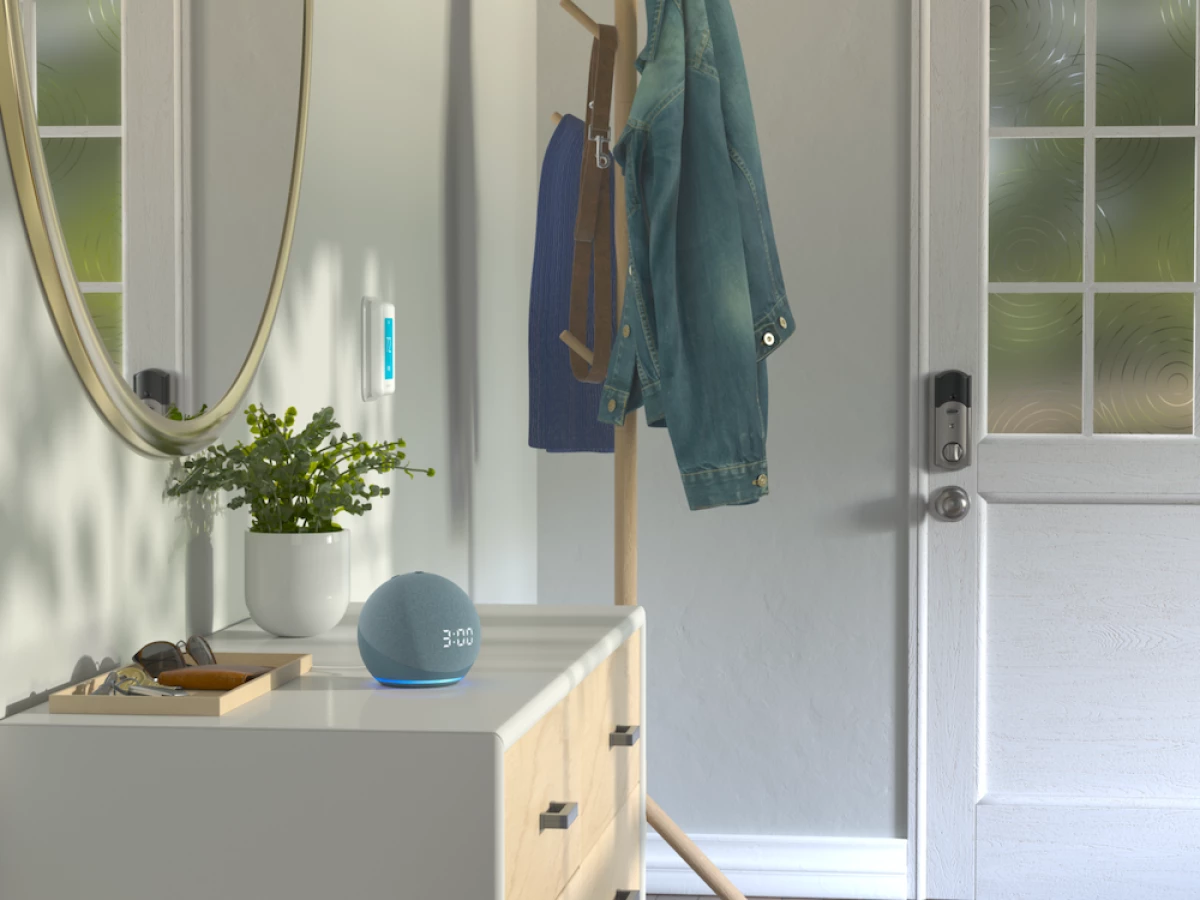\n\nIn the \"age of self\", AIs will be able to infer customers’ implicit intentions from observable temporal patterns, such as interactions with smart-home devices like thermostats, door locks, and lights.\n\nSelf-service means, essentially, the democratization of AI. Alexa customers with no programming experience should be able to customize Alexa’s services and even create new Alexa capabilities, and skill developers without machine learning experience should be able to build complex yet robust conversational skills. Colloquially, these are the conversational-AI equivalents of no-code and low-code development environments.\n\nTo be clear, the age of self is not yet upon us, and its dawning will require the maturation of technologies still under development, at Amazon and elsewhere. But some of Alexa’s recently launched capabilities herald a lightening in the Eastern sky.\n\n#### **Self-awareness**\n\nIn 2018, we launched Alexa hunches for the smart home, with Alexa suggesting actions to take in response to anomalous sensor data. By early 2021, the science has advanced adequately for us to launch an opt-in service in which Alexa can take action immediately and automatically. In the meantime, we’ve also been working to expand hunches to Alexa services other than the smart home.\n\n#### **\"Technologies will bring a new level of generalizability and autonomy to both the Alexa voice service and the tools available to Alexa developers, ushering in what I like to think of as a new 'age of self' in artificial intelligence.\"**\n\nBut commonsense reasoning requires something more — the ability to infer customers’ implicit intentions from observable temporal patterns. For instance, what does it mean if the customer turns down the thermostat, turns out the lights, locks the front door, and opens the garage? What if the customer initiates an interaction with a query like “Alexa, what’s playing at Rolling Hills Cine Plaza?”\n\nIn 2020, we took steps toward commonsense reasoning with a new Alexa function that can [infer a customer’s latent goal](https://www.amazon.science/blog/alexa-gets-better-at-predicting-customers-goals)— the ultimate aim that lies behind a sequence of requests. When a customer asks for the weather at the beach, for instance, Alexa might use that query, in combination with other contextual information, to infer that the customer may be interested in a trip to the beach. Alexa could then offer the current driving time to the beach.\n\nTo retrieve that information, Alexa has to know to map the location of the weather request to the destination variable in the route-planning function. This illustrates another aspect of self-awareness: the ability to track information across contexts.\n\nThat ability is at the core of the [night-out experience](https://www.amazon.science/blog/amazon-unveils-novel-alexa-dialog-modeling-for-natural-cross-skill-conversations) we’ve developed, which engages the customer in a multiturn conversation to plan a complete night out, from buying movie tickets to making restaurant and ride-share reservations. The night-out experience tracks times and locations across skills, revising them on the fly as customers evaluate different options. To build the experience, we leveraged the machinery of [Alexa Conversations](https://developer.amazon.com/en-US/docs/alexa/conversations/about-alexa-conversations.html), a service that enables developers to quickly and easily create dialogue-driven skills, and we drew on our [growing body of research](https://www.amazon.science/blog/new-alexa-research-on-task-oriented-dialogue-systems) on dialogue [state tracking](https://www.amazon.science/blog/turning-dialogue-tracking-into-a-reading-comprehension-problem).\n\nSelf-awareness, however, includes an understanding not only of the conversational context but also of the customer’s physical context. In 2020, we demonstrated [natural turn-taking](https://www.amazon.science/blog/change-to-alexa-wake-word-process-adds-natural-turn-taking) on Alexa-enabled devices with cameras. When multiple speakers are engaging with Alexa, Alexa can use visual cues to distinguish between speech the customers are directing at each other and speech they’re directing at Alexa. In ongoing work, we’re working to expand this functionality to devices without cameras, by [relying solely on acoustic and linguistic signals](https://www.amazon.science/blog/how-alexa-knows-when-youre-talking-to-her).\n\n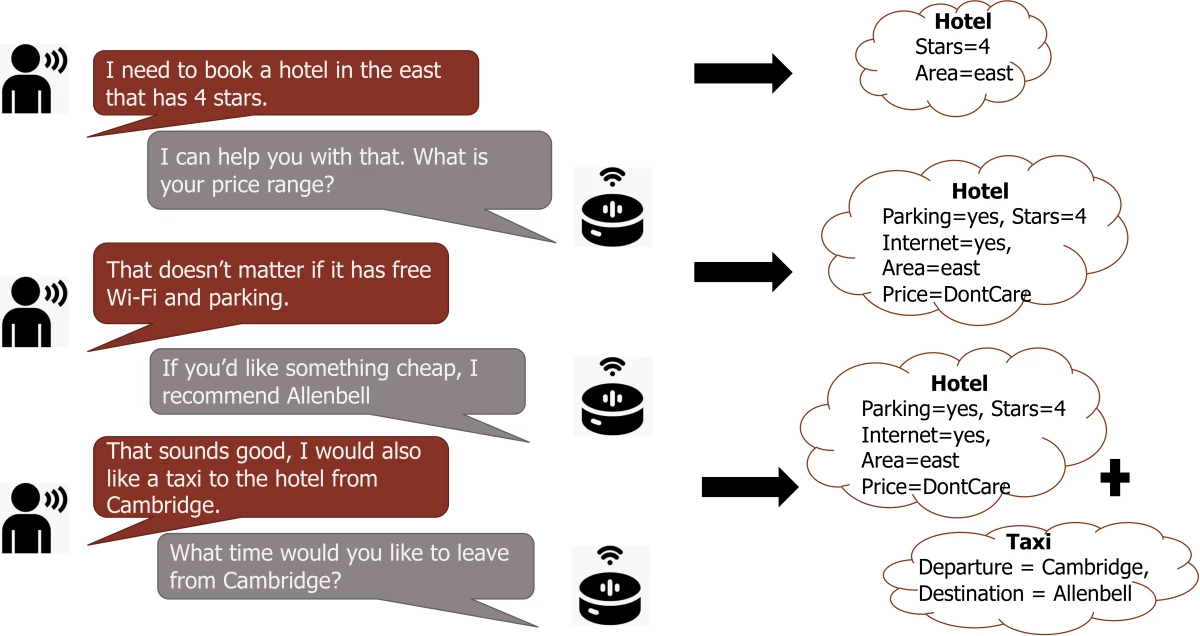\n\nDialogue states at several successive dialogue turns\n\nFinally, self-awareness also entails the capacity for self-explanation. Today, most machine learning models are black boxes; even their creators have no idea how they’re doing what they do. That uncertainty has turned explainable or interpretable AI into a popular research topic.\n\nAmazon [actively publishes](https://www.amazon.science/tag/explainable-AI) on explainable-AI topics. In addition, the Alexa Fund, an Amazon venture capital investment program, invested in [fiddler](https://www.fiddler.ai/).ai, a startup that uses techniques based on [the game-theoretical concept of Shapley values](https://www.youtube.com/watch?v=dm1gTKiVqBE&t=465s) to do explainable AI.\n\n#### **Self-learning**\n\nHistorically, the AI development cycle has involved collection of data, annotation of that data, and retraining of models on the newly annotated data — all of which add up to a laborious process.\n\nIn 2019, we launched Alexa’s [self-learning](https://www.amazon.science/blog/how-we-taught-alexa-to-correct-her-own-defects) system, which automatically learns to correct errors — both customer errors and errors in Alexa’s language-understanding models — without human involvement. The system relies on implicit signals that a request was improperly handled, as when a customer interrupts a response and rephrases the same request.\n\n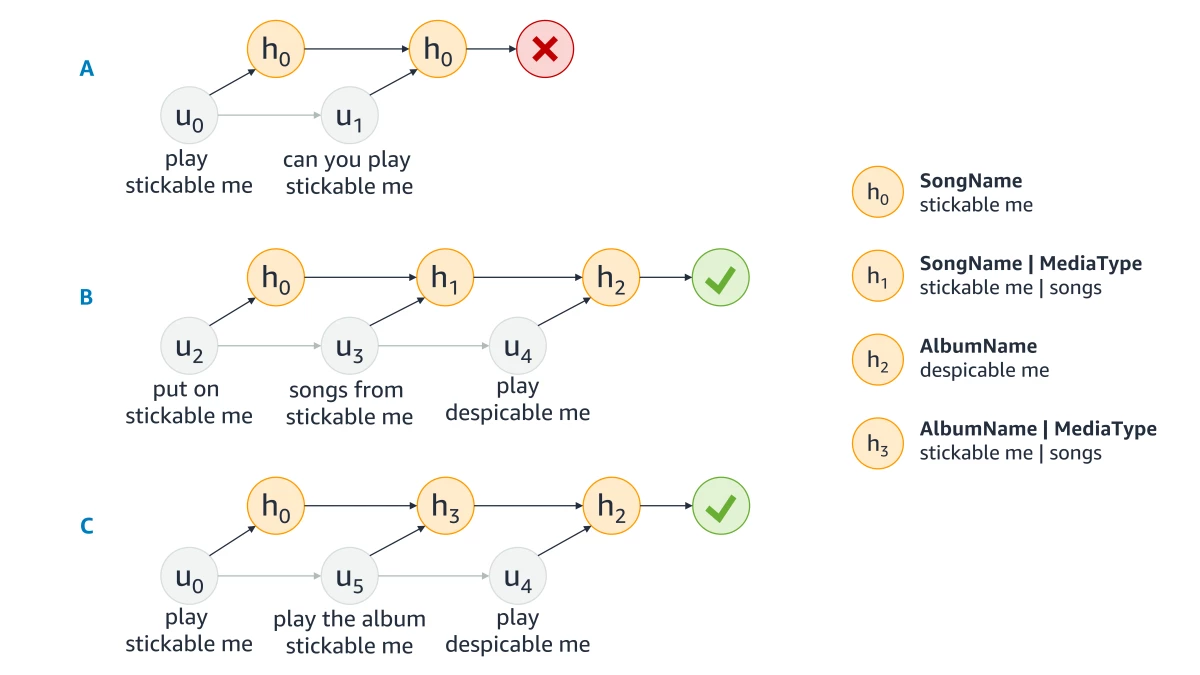\n\nAlexa's self-learning system models customer interactions with Alexa as sequences of states; different customer utterances (u0, u1, u2) can correspond to the same state (h0). The final state of a sequence, known as the \"absorbing state\", indicates the success (checkmark) or failure (X) of a transaction.\nSTACY REILLY\n\nCurrently, that fully automatic system is correcting 15% of defects. But those are defects that occur across a spectrum of users; only when enough people implicitly identify the same flaw does the system address it. We are working to adapt the same machinery to individual customers’ preferences — so that, for instance, Alexa can learn that when a particular customer asks for the song “Wow”, she means not the Post Malone hit from 2019 but the 1978 Kate Bush song.\n\nCustomers today also have the option of explicitly teaching Alexa their preferences. In the fall of 2020, we launched [interactive teaching by customers](https://www.amazon.science/blog/new-alexa-features-interactive-teaching-by-customers), a capability that enables customers to instruct Alexa how they want certain requests to be handled. For instance, the customer can teach Alexa that the command “reading mode” means lights turned all the way up, while “movie mode” means only twenty percent up.\n\n#### **Self-service**\n\nInteractive teaching is also an early example of how Alexa is enabling more self-service. It extends prior Alexa features, like [blueprints](https://blueprints.amazon.com/), which let customers build their own simple skills from preexisting templates, and [routines](https://www.amazon.com/alexa-routines/b?ie=UTF8&node=21442922011&tag=googhydr-20&hvadid=485647946139&hvpos=&hvexid=&hvnetw=g&hvrand=17766641030068630847&hvpone=&hvptwo=&hvqmt=e&hvdev=c&hvdvcmdl=&hvlocint=&hvlocphy=1018405&hvtargid=kwd-365377961441&ref=pd_sl_1yrrvfse6f_e), which let customers chain together sequences of actions under individual commands.\n\nIn March 2021, we announced the [public release](https://www.amazon.science/latest-news/art-institute-of-chicago-alexa-conversations-art-museum-skill) of Alexa Conversations, which allows developers to create dialogue-driven skills by uploading sample dialogues. Alexa Conversations’ [sophisticated machine learning models](https://www.amazon.science/blog/science-innovations-power-alexa-conversations-dialogue-management) use those dialogues as templates for generating larger corpora of synthetic training data. From that data, Alexa Conversations automatically trains a machine learning model.\n\nAlexa Conversations does, however, require the developer to specify the set of entities that the new model should act upon and an application programming interface for the skill. So while it requires little familiarity with machine learning, it assumes some programming experience. \n\nWe are steadily chipping away at even that requirement, by making development for Alexa easier and more intuitive. As Alexa’s repertory of skills grows, for instance, entities are frequently reused, and we already have systems that can [inform developers about entity types](https://www.amazon.science/blog/new-ai-system-helps-accelerate-alexa-skill-development) that they might not have thought to add to their skills. This is a step toward a self-service model in which developers no longer have to provide exhaustive lists of entities — or, in some cases, any entities at all.\n\n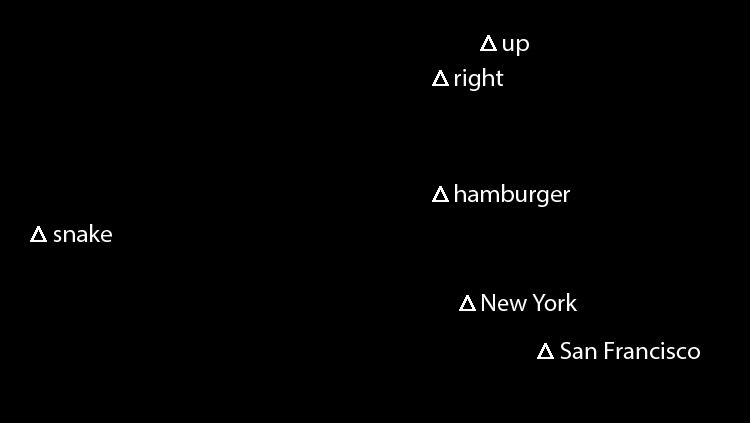\n\nAn Alexa feature known as catalogue value suggestions suggests entity names to skill developers on the basis of their \"embeddings\", or locations in a representational space. If the embeddings of values (such as bird, dog, or cat) for a particular entity type are close enough (dotted circles) to their averages (solid circle and square), the system suggests new entity names; otherwise, it concludes that suggestions would be unproductive.\nANIMATION BY [NICK LITTLE](https://www.nicholaslittleillustration.com/)\n\nAnother technique that makes it easier to build machine learning models is [few-shot learning](https://www.amazon.science/tag/few-shot-learning), in which an existing model is generalized to a related task using only a handful of new training examples. This is an active area of research at Alexa: earlier this year, for example, we [presented a paper](https://www.amazon.science/blog/learning-new-language-understanding-tasks-from-just-a-few-examples) at the Spoken Language Technologies conference that described a new approach to few-shot learning for natural-language-understanding tasks. Compared to its predecessors, our approach reduced the error rate on certain natural-language-understanding tasks by up to 12.4%, when each model was trained on only 10 examples.\n\nThese advances, along with the others [reported on Amazon Science](https://www.amazon.science/tag/alexa), demonstrate that the Alexa AI team continues to accelerate its pace of invention. More exciting announcements lie just over the horizon. I’ll be stopping back here every once in a while to update you on Alexa’s journey into the age of self.\n","render":"<p>Alexa launched in 2014, and in the more than six years since, we’ve been making good on our promise to make Alexa smarter every day. In addition to foundational improvements in Alexa’s core AI technologies, such as <a href=\"https://www.amazon.science/tag/asr\" target=\"_blank\">speech recognition</a> and <a href=\"https://www.amazon.science/tag/nlu\" target=\"_blank\">natural-language-understanding</a> systems, Alexa scientists have developed technologies that continue to delight our customers, such as <a href=\"https://www.amazon.science/blog/whisper-to-alexa-and-shell-whisper-back\" target=\"_blank\">whispered speech</a> and Alexa’s new <a href=\"https://www.amazon.science/blog/how-alexas-new-live-translation-for-conversations-works\" target=\"_blank\">live translation</a> service.</p>\n<p><img src=\"https://dev-media.amazoncloud.cn/e9115b02b9014cb5b4a69509053dd521_image.png\" alt=\"image.png\" /></p>\n<p>Prem Natarajan, Alexa AI vice president of natural understanding, at a conference in 2018.</p>\n<p>But some of the technologies we’ve begun to introduce, together with others we’re now investigating, are harbingers of a step change in Alexa’s development — and in the field of AI itself. Collectively, these technologies will bring a new level of generalizability and autonomy to both the Alexa voice service and the tools available to Alexa developers, ushering in what I like to think of as a new “age of self” in artificial intelligence, an age in which AI systems such as Alexa become more self-aware and more self-learning, and in which they lend themselves to self-service by experienced developers and even end users.</p>\n<p>By self-awareness, I mean the ability to maintain an awareness of ambient state (e.g., time of day, thermostat readings, and recent actions) and to employ commonsense reasoning to make inferences that reflect that awareness and prior/world knowledge. Alexa <a href=\"https://www.youtube.com/watch?v=whahElqS5eA\" target=\"_blank\">hunches</a> can already recognize anomalies in customers’ daily routines and suggest corrections — noticing that a light was left on at night and offering to turn it off, for instance. Powered by commonsense reasoning, self-awareness goes further: for instance, if a customer turns on the television five minutes before the kids’ soccer practice is scheduled to end, an AI of the future might infer that the customer needs a reminder about pickup.</p>\n<p>Self-learning is Alexa’s ability to improve and expand its capabilities without human intervention. And like self-awareness, self-learning employs reasoning: for example, does the customer’s response to an action indicate dissatisfaction with that action? Similarly, when a customer issues an unfamiliar command, a truly self-learning Alexa would be able to infer what it might mean — perhaps by searching the web or exploring a knowledge base — and suggest possibilities.</p>\n<p><img src=\"https://dev-media.amazoncloud.cn/552e6a1b86f74b7bb3381d140937ca03_image.png\" alt=\"image.png\" /></p>\n<p>In the “age of self”, AIs will be able to infer customers’ implicit intentions from observable temporal patterns, such as interactions with smart-home devices like thermostats, door locks, and lights.</p>\n<p>Self-service means, essentially, the democratization of AI. Alexa customers with no programming experience should be able to customize Alexa’s services and even create new Alexa capabilities, and skill developers without machine learning experience should be able to build complex yet robust conversational skills. Colloquially, these are the conversational-AI equivalents of no-code and low-code development environments.</p>\n<p>To be clear, the age of self is not yet upon us, and its dawning will require the maturation of technologies still under development, at Amazon and elsewhere. But some of Alexa’s recently launched capabilities herald a lightening in the Eastern sky.</p>\n<h4><a id=\"Selfawareness_20\"></a><strong>Self-awareness</strong></h4>\n<p>In 2018, we launched Alexa hunches for the smart home, with Alexa suggesting actions to take in response to anomalous sensor data. By early 2021, the science has advanced adequately for us to launch an opt-in service in which Alexa can take action immediately and automatically. In the meantime, we’ve also been working to expand hunches to Alexa services other than the smart home.</p>\n<h4><a id=\"Technologies_will_bring_a_new_level_of_generalizability_and_autonomy_to_both_the_Alexa_voice_service_and_the_tools_available_to_Alexa_developers_ushering_in_what_I_like_to_think_of_as_a_new_age_of_self_in_artificial_intelligence_24\"></a><strong>"Technologies will bring a new level of generalizability and autonomy to both the Alexa voice service and the tools available to Alexa developers, ushering in what I like to think of as a new ‘age of self’ in artificial intelligence."</strong></h4>\n<p>But commonsense reasoning requires something more — the ability to infer customers’ implicit intentions from observable temporal patterns. For instance, what does it mean if the customer turns down the thermostat, turns out the lights, locks the front door, and opens the garage? What if the customer initiates an interaction with a query like “Alexa, what’s playing at Rolling Hills Cine Plaza?”</p>\n<p>In 2020, we took steps toward commonsense reasoning with a new Alexa function that can <a href=\"https://www.amazon.science/blog/alexa-gets-better-at-predicting-customers-goals\" target=\"_blank\">infer a customer’s latent goal</a>— the ultimate aim that lies behind a sequence of requests. When a customer asks for the weather at the beach, for instance, Alexa might use that query, in combination with other contextual information, to infer that the customer may be interested in a trip to the beach. Alexa could then offer the current driving time to the beach.</p>\n<p>To retrieve that information, Alexa has to know to map the location of the weather request to the destination variable in the route-planning function. This illustrates another aspect of self-awareness: the ability to track information across contexts.</p>\n<p>That ability is at the core of the <a href=\"https://www.amazon.science/blog/amazon-unveils-novel-alexa-dialog-modeling-for-natural-cross-skill-conversations\" target=\"_blank\">night-out experience</a> we’ve developed, which engages the customer in a multiturn conversation to plan a complete night out, from buying movie tickets to making restaurant and ride-share reservations. The night-out experience tracks times and locations across skills, revising them on the fly as customers evaluate different options. To build the experience, we leveraged the machinery of <a href=\"https://developer.amazon.com/en-US/docs/alexa/conversations/about-alexa-conversations.html\" target=\"_blank\">Alexa Conversations</a>, a service that enables developers to quickly and easily create dialogue-driven skills, and we drew on our <a href=\"https://www.amazon.science/blog/new-alexa-research-on-task-oriented-dialogue-systems\" target=\"_blank\">growing body of research</a> on dialogue <a href=\"https://www.amazon.science/blog/turning-dialogue-tracking-into-a-reading-comprehension-problem\" target=\"_blank\">state tracking</a>.</p>\n<p>Self-awareness, however, includes an understanding not only of the conversational context but also of the customer’s physical context. In 2020, we demonstrated <a href=\"https://www.amazon.science/blog/change-to-alexa-wake-word-process-adds-natural-turn-taking\" target=\"_blank\">natural turn-taking</a> on Alexa-enabled devices with cameras. When multiple speakers are engaging with Alexa, Alexa can use visual cues to distinguish between speech the customers are directing at each other and speech they’re directing at Alexa. In ongoing work, we’re working to expand this functionality to devices without cameras, by <a href=\"https://www.amazon.science/blog/how-alexa-knows-when-youre-talking-to-her\" target=\"_blank\">relying solely on acoustic and linguistic signals</a>.</p>\n<p><img src=\"https://dev-media.amazoncloud.cn/24e236a7202149bba549cc78bd60aefb_image.png\" alt=\"image.png\" /></p>\n<p>Dialogue states at several successive dialogue turns</p>\n<p>Finally, self-awareness also entails the capacity for self-explanation. Today, most machine learning models are black boxes; even their creators have no idea how they’re doing what they do. That uncertainty has turned explainable or interpretable AI into a popular research topic.</p>\n<p>Amazon <a href=\"https://www.amazon.science/tag/explainable-AI\" target=\"_blank\">actively publishes</a> on explainable-AI topics. In addition, the Alexa Fund, an Amazon venture capital investment program, invested in <a href=\"https://www.fiddler.ai/\" target=\"_blank\">fiddler</a>.ai, a startup that uses techniques based on <a href=\"https://www.youtube.com/watch?v=dm1gTKiVqBE&t=465s\" target=\"_blank\">the game-theoretical concept of Shapley values</a> to do explainable AI.</p>\n<h4><a id=\"Selflearning_44\"></a><strong>Self-learning</strong></h4>\n<p>Historically, the AI development cycle has involved collection of data, annotation of that data, and retraining of models on the newly annotated data — all of which add up to a laborious process.</p>\n<p>In 2019, we launched Alexa’s <a href=\"https://www.amazon.science/blog/how-we-taught-alexa-to-correct-her-own-defects\" target=\"_blank\">self-learning</a> system, which automatically learns to correct errors — both customer errors and errors in Alexa’s language-understanding models — without human involvement. The system relies on implicit signals that a request was improperly handled, as when a customer interrupts a response and rephrases the same request.</p>\n<p><img src=\"https://dev-media.amazoncloud.cn/e7602fed8ac04f9fa4695b33d1e3e02d_image.png\" alt=\"image.png\" /></p>\n<p>Alexa’s self-learning system models customer interactions with Alexa as sequences of states; different customer utterances (u0, u1, u2) can correspond to the same state (h0). The final state of a sequence, known as the “absorbing state”, indicates the success (checkmark) or failure (X) of a transaction.<br />\nSTACY REILLY</p>\n<p>Currently, that fully automatic system is correcting 15% of defects. But those are defects that occur across a spectrum of users; only when enough people implicitly identify the same flaw does the system address it. We are working to adapt the same machinery to individual customers’ preferences — so that, for instance, Alexa can learn that when a particular customer asks for the song “Wow”, she means not the Post Malone hit from 2019 but the 1978 Kate Bush song.</p>\n<p>Customers today also have the option of explicitly teaching Alexa their preferences. In the fall of 2020, we launched <a href=\"https://www.amazon.science/blog/new-alexa-features-interactive-teaching-by-customers\" target=\"_blank\">interactive teaching by customers</a>, a capability that enables customers to instruct Alexa how they want certain requests to be handled. For instance, the customer can teach Alexa that the command “reading mode” means lights turned all the way up, while “movie mode” means only twenty percent up.</p>\n<h4><a id=\"Selfservice_59\"></a><strong>Self-service</strong></h4>\n<p>Interactive teaching is also an early example of how Alexa is enabling more self-service. It extends prior Alexa features, like <a href=\"https://blueprints.amazon.com/\" target=\"_blank\">blueprints</a>, which let customers build their own simple skills from preexisting templates, and <a href=\"https://www.amazon.com/alexa-routines/b?ie=UTF8&node=21442922011&tag=googhydr-20&hvadid=485647946139&hvpos=&hvexid=&hvnetw=g&hvrand=17766641030068630847&hvpone=&hvptwo=&hvqmt=e&hvdev=c&hvdvcmdl=&hvlocint=&hvlocphy=1018405&hvtargid=kwd-365377961441&ref=pd_sl_1yrrvfse6f_e\" target=\"_blank\">routines</a>, which let customers chain together sequences of actions under individual commands.</p>\n<p>In March 2021, we announced the <a href=\"https://www.amazon.science/latest-news/art-institute-of-chicago-alexa-conversations-art-museum-skill\" target=\"_blank\">public release</a> of Alexa Conversations, which allows developers to create dialogue-driven skills by uploading sample dialogues. Alexa Conversations’ <a href=\"https://www.amazon.science/blog/science-innovations-power-alexa-conversations-dialogue-management\" target=\"_blank\">sophisticated machine learning models</a> use those dialogues as templates for generating larger corpora of synthetic training data. From that data, Alexa Conversations automatically trains a machine learning model.</p>\n<p>Alexa Conversations does, however, require the developer to specify the set of entities that the new model should act upon and an application programming interface for the skill. So while it requires little familiarity with machine learning, it assumes some programming experience.</p>\n<p>We are steadily chipping away at even that requirement, by making development for Alexa easier and more intuitive. As Alexa’s repertory of skills grows, for instance, entities are frequently reused, and we already have systems that can <a href=\"https://www.amazon.science/blog/new-ai-system-helps-accelerate-alexa-skill-development\" target=\"_blank\">inform developers about entity types</a> that they might not have thought to add to their skills. This is a step toward a self-service model in which developers no longer have to provide exhaustive lists of entities — or, in some cases, any entities at all.</p>\n<p><img src=\"https://dev-media.amazoncloud.cn/14da50af593d47b8bd1fb8b40789f6eb_%E4%B8%8B%E8%BD%BD.gif\" alt=\"下载.gif\" /></p>\n<p>An Alexa feature known as catalogue value suggestions suggests entity names to skill developers on the basis of their “embeddings”, or locations in a representational space. If the embeddings of values (such as bird, dog, or cat) for a particular entity type are close enough (dotted circles) to their averages (solid circle and square), the system suggests new entity names; otherwise, it concludes that suggestions would be unproductive.<br />\nANIMATION BY <a href=\"https://www.nicholaslittleillustration.com/\" target=\"_blank\">NICK LITTLE</a></p>\n<p>Another technique that makes it easier to build machine learning models is <a href=\"https://www.amazon.science/tag/few-shot-learning\" target=\"_blank\">few-shot learning</a>, in which an existing model is generalized to a related task using only a handful of new training examples. This is an active area of research at Alexa: earlier this year, for example, we <a href=\"https://www.amazon.science/blog/learning-new-language-understanding-tasks-from-just-a-few-examples\" target=\"_blank\">presented a paper</a> at the Spoken Language Technologies conference that described a new approach to few-shot learning for natural-language-understanding tasks. Compared to its predecessors, our approach reduced the error rate on certain natural-language-understanding tasks by up to 12.4%, when each model was trained on only 10 examples.</p>\n<p>These advances, along with the others <a href=\"https://www.amazon.science/tag/alexa\" target=\"_blank\">reported on Amazon Science</a>, demonstrate that the Alexa AI team continues to accelerate its pace of invention. More exciting announcements lie just over the horizon. I’ll be stopping back here every once in a while to update you on Alexa’s journey into the age of self.</p>\n"}