{"value":"[Epos Now](https://www.eposnow.com/au/) provides point of sale and payment solutions to over 40,000 hospitality and retailers across 71 countries. Their mission is to help businesses of all sizes reach their full potential through the power of cloud technology, with solutions that are affordable, efficient, and accessible. Their solutions allow businesses to leverage actionable insights, manage their business from anywhere, and reach customers both in-store and online.\n\nEpos Now currently provides real-time and near-real-time reports and dashboards to their merchants on top of their operational database (Microsoft SQL Server). With a growing customer base and new data needs, the team started to see some issues in the current platform.\n\nFirst, they observed performance degradation for serving the reporting requirements from the same OLTP database with the current data model. A few metrics that needed to be delivered in real time (seconds after a transaction was complete) and a few metrics that needed to be reflected in the dashboard in near-real-time (minutes) took several attempts to load in the dashboard.\n\nThis started to cause operational issues for their merchants. The end consumers of reports couldn’t access the dashboard in a timely manner.\n\nCost and scalability also became a major problem because one single database instance was trying to serve many different use cases.\n\nEpos Now needed a strategic solution to address these issues. Additionally, they didn’t have a dedicated data platform for doing machine learning and advanced analytics use cases, so they decided on two parallel strategies to resolve their data problems and better serve merchants:\n\n- The first was to rearchitect the near-real-time reporting feature by moving it to a dedicated [Amazon Aurora PostgreSQL-Compatible Edition](https://aws.amazon.com/cn/rds/aurora/) database, with a specific reporting data model to serve to end consumers. This will improve performance, uptime, and cost.\n- The second was to build out a new data platform for reporting, dashboards, and advanced analytics. This will enable use cases for internal data analysts and data scientists to experiment and create multiple data products, ultimately exposing these insights to end customers.\n\nIn this post, we discuss how Epos Now designed the overall solution with support from the [Amazon Web Services Data Lab](https://aws.amazon.com/cn/aws-data-lab/). Having developed a strong strategic relationship with Amazon Web Services over the last 3 years, Epos Now opted to take advantage of the Amazon Web Services Data lab program to speed up the process of building a reliable, performant, and cost-effective data platform. The Amazon Web Services Data Lab program offers accelerated, joint-engineering engagements between customers and Amazon Web Services technical resources to create tangible deliverables that accelerate data and analytics modernization initiatives.\n\nWorking with an Amazon Web Services Data Lab Architect, Epos Now commenced weekly cadence calls to come up with a high-level architecture. After the objective, success criteria, and stretch goals were clearly defined, the final step was to draft a detailed task list for the upcoming 3-day build phase.\n\n\n#### **Overview of solution**\n\n\nAs part of the 3-day build exercise, Epos Now built the following solution with the ongoing support of their Amazon Web Services Data Lab Architect.\n\n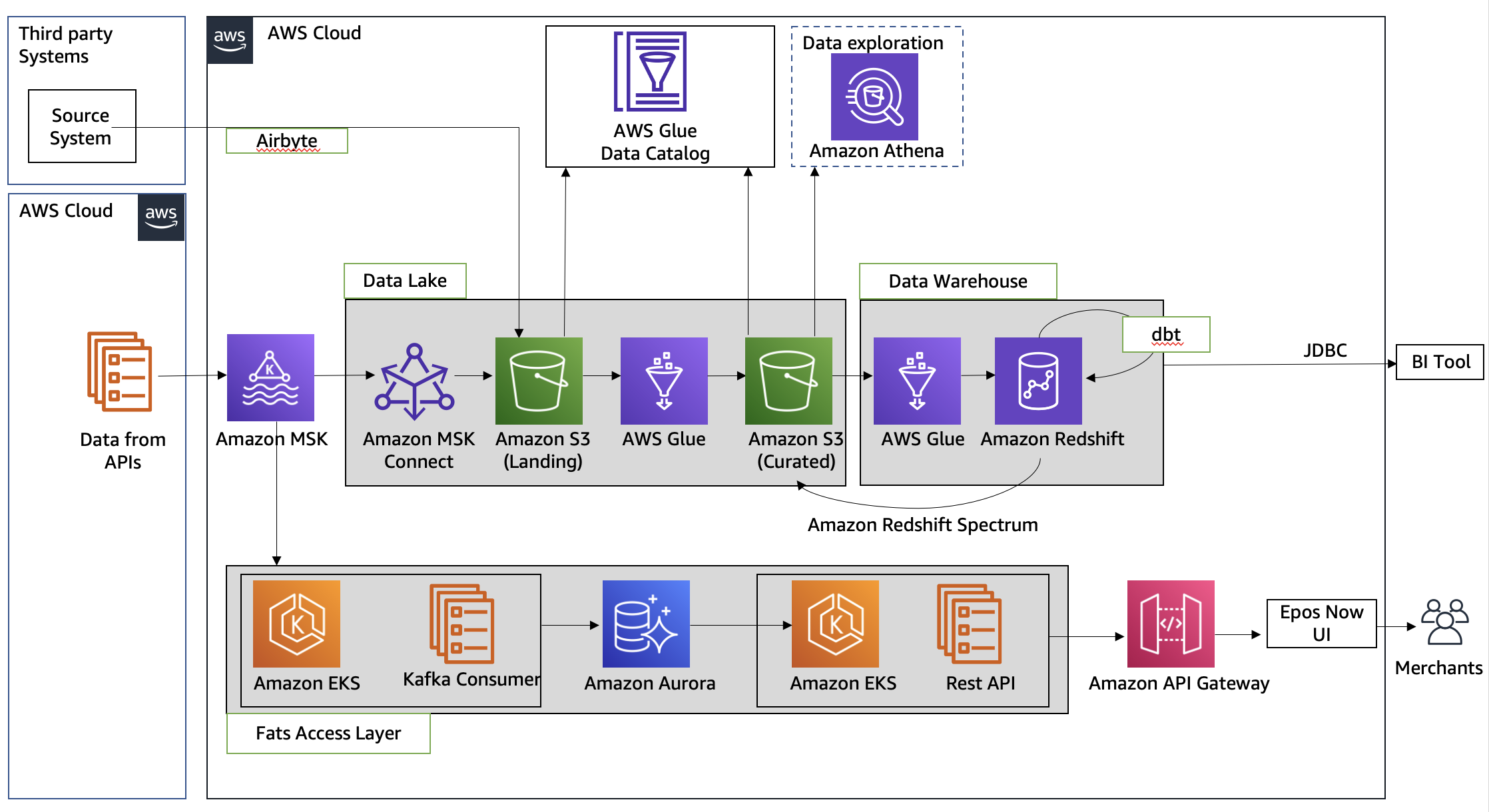\n\nThe platform consists of an end-to-end data pipeline with three main components:\n\n- **Data lake** – As a central source of truth\n- **Data warehouse** – For analytics and reporting needs\n- **Fast access layer** – To serve near-real-time reports to merchants\n\nWe chose three different storage solutions:\n\n- [Amazon Simple Storage Service](https://aws.amazon.com/cn/s3/) ([Amazon S3](https://aws.amazon.com/cn/s3/?trk=cndc-detail)) for raw data landing and a curated data layer to build the foundation of the data lake\n- [Amazon Redshift](https://aws.amazon.com/cn/redshift/?trk=cndc-detail) to create a federated data warehouse with conformed dimensions and star schemas for consumption by Microsoft Power BI, running on Amazon Web Services \n- Aurora PostgreSQL to store all the data for near-real-time reporting as a fast access layer\n\nIn the following sections, we go into each component and supporting services in more detail.\n\n\n#### **Data lake**\n\n\nThe first component of the data pipeline involved ingesting the data from an[ Amazon Managed Streaming for Apache Kafka](https://aws.amazon.com/cn/msk/) (Amazon MSK) topic using [Amazon MSK Connect](https://aws.amazon.com/cn/msk/features/msk-connect/) to land the data into an S3 bucket (landing zone). The Epos Now team used the Confluent [Amazon S3](https://aws.amazon.com/cn/s3/?trk=cndc-detail) sink connector to sink the data to [Amazon S3](https://aws.amazon.com/cn/s3/?trk=cndc-detail). To make the sink process more resilient, Epos Now added the required configuration for dead-letter queues to redirect the bad messages to another topic. The following code is a sample configuration for a dead-letter queue in Amazon MSK Connect:\n\n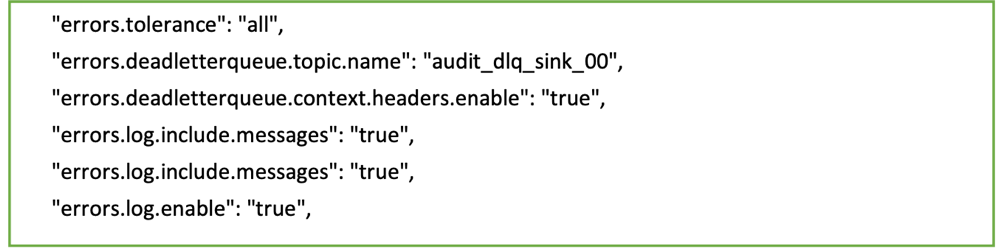\n\nBecause Epos Now was ingesting from multiple data sources, they used Airbyte to transfer the data to a landing zone in batches. A subsequent [Amazon Web Services Glue](https://aws.amazon.com/cn/glue/?whats-new-cards.sort-by=item.additionalFields.postDateTime&whats-new-cards.sort-order=desc) job reads the data from the landing bucket , performs data transformation, and moves the data to a curated zone of [Amazon S3](https://aws.amazon.com/cn/s3/?trk=cndc-detail) in optimal format and layout. This curated layer then became the source of truth for all other use cases. Then Epos Now used an Amazon Web Services Glue crawler to update the Amazon Web Services Glue Data Catalog. This was augmented by the use of [Amazon Athena](https://aws.amazon.com/cn/athena/?whats-new-cards.sort-by=item.additionalFields.postDateTime&whats-new-cards.sort-order=desc) for doing data analysis. To optimize for cost, Epos Now defined an optimal [data retention policy](https://docs.aws.amazon.com/AmazonS3/latest/userguide/how-to-set-lifecycle-configuration-intro.html) on different layers of the data lake to save money as well as keep the dataset relevant.\n\n\n#### **Data warehouse**\n\n\nAfter the data lake foundation was established, Epos Now used a subsequent Amazon Web Services Glue job to load the data from the S3 curated layer to [Amazon Redshift](https://aws.amazon.com/cn/redshift/?trk=cndc-detail). We used [Amazon Redshift](https://aws.amazon.com/cn/redshift/?trk=cndc-detail) to make the data queryable in both [Amazon Redshift](https://aws.amazon.com/cn/redshift/?trk=cndc-detail) (internal tables) and [Amazon Redshift Spectrum](https://docs.aws.amazon.com/redshift/latest/dg/c-getting-started-using-spectrum.html). The team then used dbt as an extract, load, and transform (ELT) engine to create the target data model and store it in target tables and views for internal business intelligence reporting. The Epos Now team wanted to use their SQL knowledge to do all ELT operations in [Amazon Redshift](https://aws.amazon.com/cn/redshift/?trk=cndc-detail), so they chose dbt to perform all the joins, aggregations, and other transformations after the data was loaded into the staging tables in [Amazon Redshift](https://aws.amazon.com/cn/redshift/?trk=cndc-detail). Epos Now is currently using Power BI for reporting, which was migrated to the Amazon Web Services Cloud and connected to [Amazon Redshift](https://aws.amazon.com/cn/redshift/?trk=cndc-detail) clusters running inside Epos Now’s VPC.\n\n\n#### **Fast access layer**\n\n\nTo build the fast access layer to deliver the metrics to Epos Now’s retail and hospitality merchants in near-real time, we decided to create a separate pipeline. This required developing a microservice running a Kafka consumer job to subscribe to the same Kafka topic in an [Amazon Elastic Kubernetes Service](https://aws.amazon.com/cn/eks/) ([Amazon EKS](https://aws.amazon.com/cn/eks/?trk=cndc-detail)) cluster. The microservice received the messages, conducted the transformations, and wrote the data to a target data model hosted on Aurora PostgreSQL. This data was delivered to the UI layer through an API also hosted on [Amazon EKS](https://aws.amazon.com/cn/eks/?trk=cndc-detail), exposed through [Amazon API Gateway](https://aws.amazon.com/cn/api-gateway/).\n\n\n#### **Outcome**\n\n\nThe Epos Now team is currently building both the fast access layer and a centralized lakehouse architecture-based data platform on [Amazon S3](https://aws.amazon.com/cn/s3/?trk=cndc-detail) and [Amazon Redshift](https://aws.amazon.com/cn/redshift/?trk=cndc-detail) for advanced analytics use cases. The new data platform is best positioned to address scalability issues and support new use cases. The Epos Now team has also started offloading some of the real-time reporting requirements to the new target data model hosted in Aurora. The team has a clear strategy around the choice of different storage solutions for the right access patterns: [Amazon S3](https://aws.amazon.com/cn/s3/?trk=cndc-detail) stores all the raw data, and Aurora hosts all the metrics to serve real-time and near-real-time reporting requirements. The Epos Now team will also enhance the overall solution by applying data retention policies in different layers of the data platform. This will address the platform cost without losing any historical datasets. The data model and structure (data partitioning, columnar file format) we designed greatly improved query performance and overall platform stability.\n\n\n#### **Conclusion**\n\n\nEpos Now revolutionized their data analytics capabilities, taking advantage of the breadth and depth of the Amazon Web Services Cloud. They’re now able to serve insights to internal business users, and scale their data platform in a reliable, performant, and cost-effective manner.\n\nThe Amazon Web Services Data Lab engagement enabled Epos Now to move from idea to proof of concept in 3 days using several previously unfamiliar Amazon Web Services analytics services, including Amazon Web Services Glue, Amazon MSK, [Amazon Redshift](https://aws.amazon.com/cn/redshift/?trk=cndc-detail), and [Amazon API Gateway](https://aws.amazon.com/cn/api-gateway/?trk=cndc-detail).\n\nEpos Now is currently in the process of implementing the full data lake architecture, with a rollout to customers planned for late 2022. Once live, they will deliver on their strategic goal to provide real-time transactional data and put insights directly in the hands of their merchants.\n\n\n#### **About the Authors**\n\n\n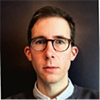\n\n**Jason Downing** is VP of Data and Insights at Epos Now. He is responsible for the Epos Now data platform and product direction. He specializes in product management across a range of industries, including POS systems, mobile money, payments, and eWallets.\n\n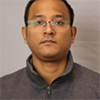\n\n**Debadatta Mohapatra** is an Amazon Web Services Data Lab Architect. He has extensive experience across big data, data science, and IoT, across consulting and industrials. He is an advocate of cloud-native data platforms and the value they can drive for customers across industries.","render":"<p><a href=\\"https://www.eposnow.com/au/\\" target=\\"_blank\\">Epos Now</a> provides point of sale and payment solutions to over 40,000 hospitality and retailers across 71 countries. Their mission is to help businesses of all sizes reach their full potential through the power of cloud technology, with solutions that are affordable, efficient, and accessible. Their solutions allow businesses to leverage actionable insights, manage their business from anywhere, and reach customers both in-store and online.</p>\\n<p>Epos Now currently provides real-time and near-real-time reports and dashboards to their merchants on top of their operational database (Microsoft SQL Server). With a growing customer base and new data needs, the team started to see some issues in the current platform.</p>\n<p>First, they observed performance degradation for serving the reporting requirements from the same OLTP database with the current data model. A few metrics that needed to be delivered in real time (seconds after a transaction was complete) and a few metrics that needed to be reflected in the dashboard in near-real-time (minutes) took several attempts to load in the dashboard.</p>\n<p>This started to cause operational issues for their merchants. The end consumers of reports couldn’t access the dashboard in a timely manner.</p>\n<p>Cost and scalability also became a major problem because one single database instance was trying to serve many different use cases.</p>\n<p>Epos Now needed a strategic solution to address these issues. Additionally, they didn’t have a dedicated data platform for doing machine learning and advanced analytics use cases, so they decided on two parallel strategies to resolve their data problems and better serve merchants:</p>\n<ul>\\n<li>The first was to rearchitect the near-real-time reporting feature by moving it to a dedicated <a href=\\"https://aws.amazon.com/cn/rds/aurora/\\" target=\\"_blank\\">Amazon Aurora PostgreSQL-Compatible Edition</a> database, with a specific reporting data model to serve to end consumers. This will improve performance, uptime, and cost.</li>\\n<li>The second was to build out a new data platform for reporting, dashboards, and advanced analytics. This will enable use cases for internal data analysts and data scientists to experiment and create multiple data products, ultimately exposing these insights to end customers.</li>\n</ul>\\n<p>In this post, we discuss how Epos Now designed the overall solution with support from the <a href=\\"https://aws.amazon.com/cn/aws-data-lab/\\" target=\\"_blank\\">Amazon Web Services Data Lab</a>. Having developed a strong strategic relationship with Amazon Web Services over the last 3 years, Epos Now opted to take advantage of the Amazon Web Services Data lab program to speed up the process of building a reliable, performant, and cost-effective data platform. The Amazon Web Services Data Lab program offers accelerated, joint-engineering engagements between customers and Amazon Web Services technical resources to create tangible deliverables that accelerate data and analytics modernization initiatives.</p>\\n<p>Working with an Amazon Web Services Data Lab Architect, Epos Now commenced weekly cadence calls to come up with a high-level architecture. After the objective, success criteria, and stretch goals were clearly defined, the final step was to draft a detailed task list for the upcoming 3-day build phase.</p>\n<h4><a id=\\"Overview_of_solution_20\\"></a><strong>Overview of solution</strong></h4>\\n<p>As part of the 3-day build exercise, Epos Now built the following solution with the ongoing support of their Amazon Web Services Data Lab Architect.</p>\n<p><img src=\\"https://dev-media.amazoncloud.cn/951f915c7f804c6baa557247bfe4536c_image.png\\" alt=\\"image.png\\" /></p>\n<p>The platform consists of an end-to-end data pipeline with three main components:</p>\n<ul>\\n<li><strong>Data lake</strong> – As a central source of truth</li>\\n<li><strong>Data warehouse</strong> – For analytics and reporting needs</li>\\n<li><strong>Fast access layer</strong> – To serve near-real-time reports to merchants</li>\\n</ul>\n<p>We chose three different storage solutions:</p>\n<ul>\\n<li><a href=\\"https://aws.amazon.com/cn/s3/\\" target=\\"_blank\\">Amazon Simple Storage Service</a> ([Amazon S3](https://aws.amazon.com/cn/s3/?trk=cndc-detail)) for raw data landing and a curated data layer to build the foundation of the data lake</li>\\n<li>Amazon Redshift to create a federated data warehouse with conformed dimensions and star schemas for consumption by Microsoft Power BI, running on Amazon Web Services</li>\n<li>Aurora PostgreSQL to store all the data for near-real-time reporting as a fast access layer</li>\n</ul>\\n<p>In the following sections, we go into each component and supporting services in more detail.</p>\n<h4><a id=\\"Data_lake_42\\"></a><strong>Data lake</strong></h4>\\n<p>The first component of the data pipeline involved ingesting the data from an<a href=\\"https://aws.amazon.com/cn/msk/\\" target=\\"_blank\\"> Amazon Managed Streaming for Apache Kafka</a> (Amazon MSK) topic using <a href=\\"https://aws.amazon.com/cn/msk/features/msk-connect/\\" target=\\"_blank\\">Amazon MSK Connect</a> to land the data into an S3 bucket (landing zone). The Epos Now team used the Confluent [Amazon S3](https://aws.amazon.com/cn/s3/?trk=cndc-detail) sink connector to sink the data to [Amazon S3](https://aws.amazon.com/cn/s3/?trk=cndc-detail). To make the sink process more resilient, Epos Now added the required configuration for dead-letter queues to redirect the bad messages to another topic. The following code is a sample configuration for a dead-letter queue in Amazon MSK Connect:</p>\\n<p><img src=\\"https://dev-media.amazoncloud.cn/5453863e446a48f5bb2505adff7f3602_image.png\\" alt=\\"image.png\\" /></p>\n<p>Because Epos Now was ingesting from multiple data sources, they used Airbyte to transfer the data to a landing zone in batches. A subsequent <a href=\\"https://aws.amazon.com/cn/glue/?whats-new-cards.sort-by=item.additionalFields.postDateTime&whats-new-cards.sort-order=desc\\" target=\\"_blank\\">Amazon Web Services Glue</a> job reads the data from the landing bucket , performs data transformation, and moves the data to a curated zone of [Amazon S3](https://aws.amazon.com/cn/s3/?trk=cndc-detail) in optimal format and layout. This curated layer then became the source of truth for all other use cases. Then Epos Now used an Amazon Web Services Glue crawler to update the Amazon Web Services Glue Data Catalog. This was augmented by the use of <a href=\\"https://aws.amazon.com/cn/athena/?whats-new-cards.sort-by=item.additionalFields.postDateTime&whats-new-cards.sort-order=desc\\" target=\\"_blank\\">Amazon Athena</a> for doing data analysis. To optimize for cost, Epos Now defined an optimal <a href=\\"https://docs.aws.amazon.com/AmazonS3/latest/userguide/how-to-set-lifecycle-configuration-intro.html\\" target=\\"_blank\\">data retention policy</a> on different layers of the data lake to save money as well as keep the dataset relevant.</p>\\n<h4><a id=\\"Data_warehouse_52\\"></a><strong>Data warehouse</strong></h4>\\n<p>After the data lake foundation was established, Epos Now used a subsequent Amazon Web Services Glue job to load the data from the S3 curated layer to Amazon Redshift. We used Amazon Redshift to make the data queryable in both Amazon Redshift (internal tables) and <a href=\\"https://docs.aws.amazon.com/redshift/latest/dg/c-getting-started-using-spectrum.html\\" target=\\"_blank\\">Amazon Redshift Spectrum</a>. The team then used dbt as an extract, load, and transform (ELT) engine to create the target data model and store it in target tables and views for internal business intelligence reporting. The Epos Now team wanted to use their SQL knowledge to do all ELT operations in [Amazon Redshift](https://aws.amazon.com/cn/redshift/?trk=cndc-detail), so they chose dbt to perform all the joins, aggregations, and other transformations after the data was loaded into the staging tables in [Amazon Redshift](https://aws.amazon.com/cn/redshift/?trk=cndc-detail). Epos Now is currently using Power BI for reporting, which was migrated to the Amazon Web Services Cloud and connected to [Amazon Redshift](https://aws.amazon.com/cn/redshift/?trk=cndc-detail) clusters running inside Epos Now’s VPC.</p>\\n<h4><a id=\\"Fast_access_layer_58\\"></a><strong>Fast access layer</strong></h4>\\n<p>To build the fast access layer to deliver the metrics to Epos Now’s retail and hospitality merchants in near-real time, we decided to create a separate pipeline. This required developing a microservice running a Kafka consumer job to subscribe to the same Kafka topic in an <a href=\\"https://aws.amazon.com/cn/eks/\\" target=\\"_blank\\">Amazon Elastic Kubernetes Service</a> ([Amazon EKS](https://aws.amazon.com/cn/eks/?trk=cndc-detail)) cluster. The microservice received the messages, conducted the transformations, and wrote the data to a target data model hosted on Aurora PostgreSQL. This data was delivered to the UI layer through an API also hosted on [Amazon EKS](https://aws.amazon.com/cn/eks/?trk=cndc-detail), exposed through <a href=\\"https://aws.amazon.com/cn/api-gateway/\\" target=\\"_blank\\">Amazon API Gateway</a>.</p>\\n<h4><a id=\\"Outcome_64\\"></a><strong>Outcome</strong></h4>\\n<p>The Epos Now team is currently building both the fast access layer and a centralized lakehouse architecture-based data platform on Amazon S3 and Amazon Redshift for advanced analytics use cases. The new data platform is best positioned to address scalability issues and support new use cases. The Epos Now team has also started offloading some of the real-time reporting requirements to the new target data model hosted in Aurora. The team has a clear strategy around the choice of different storage solutions for the right access patterns: Amazon S3 stores all the raw data, and Aurora hosts all the metrics to serve real-time and near-real-time reporting requirements. The Epos Now team will also enhance the overall solution by applying data retention policies in different layers of the data platform. This will address the platform cost without losing any historical datasets. The data model and structure (data partitioning, columnar file format) we designed greatly improved query performance and overall platform stability.</p>\n<h4><a id=\\"Conclusion_70\\"></a><strong>Conclusion</strong></h4>\\n<p>Epos Now revolutionized their data analytics capabilities, taking advantage of the breadth and depth of the Amazon Web Services Cloud. They’re now able to serve insights to internal business users, and scale their data platform in a reliable, performant, and cost-effective manner.</p>\n<p>The Amazon Web Services Data Lab engagement enabled Epos Now to move from idea to proof of concept in 3 days using several previously unfamiliar Amazon Web Services analytics services, including Amazon Web Services Glue, Amazon MSK, Amazon Redshift, and Amazon API Gateway.</p>\n<p>Epos Now is currently in the process of implementing the full data lake architecture, with a rollout to customers planned for late 2022. Once live, they will deliver on their strategic goal to provide real-time transactional data and put insights directly in the hands of their merchants.</p>\n<h4><a id=\\"About_the_Authors_80\\"></a><strong>About the Authors</strong></h4>\\n<p><img src=\\"https://dev-media.amazoncloud.cn/cb0dee3ecb25477d8541c7eec254782f_image.png\\" alt=\\"image.png\\" /></p>\n<p><strong>Jason Downing</strong> is VP of Data and Insights at Epos Now. He is responsible for the Epos Now data platform and product direction. He specializes in product management across a range of industries, including POS systems, mobile money, payments, and eWallets.</p>\\n<p><img src=\\"https://dev-media.amazoncloud.cn/184146fab79145b985646d773b51e727_image.png\\" alt=\\"image.png\\" /></p>\n<p><strong>Debadatta Mohapatra</strong> is an Amazon Web Services Data Lab Architect. He has extensive experience across big data, data science, and IoT, across consulting and industrials. He is an advocate of cloud-native data platforms and the value they can drive for customers across industries.</p>\n"}