{"value":"As the largest conference devoted to speech technologies, Interspeech has long been a showcase for the latest research on automatic speech recognition (ASR) from Amazon Alexa. This year, Alexa researchers had 12 ASR papers accepted at the conference.\n\n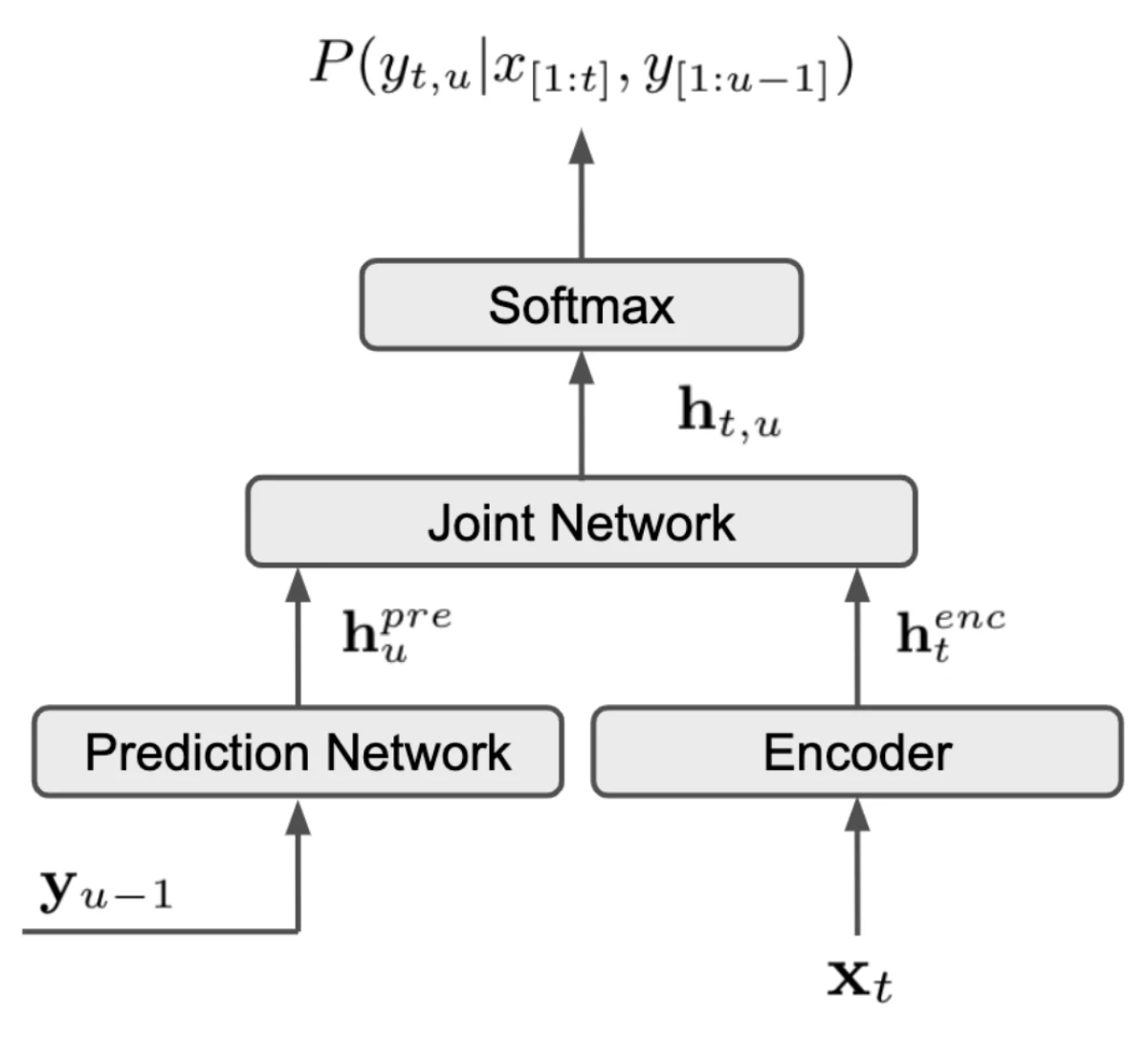\n\nThe architecture of the RNN-T ASR system. Xt indicates the current frame of the acoustic signal. Yu-1 indicates the sequence of output subwords corresponding to the preceding frames.\n\nFROM \"EFFICIENT MINIMUM WORD ERROR RATE TRAINING OF RNN-TRANSDUCER FOR END-TO-END SPEECH RECOGNITION\"\n\nOne of these, “++[Speaker identification for household scenarios with self-attention and adversarial training](https://www.amazon.science/publications/speaker-identification-for-household-scenarios-with-self-attention-and-adversarial-training)++”, reports the speech team’s recent innovations in speaker ID, or recognizing which of several possible speakers is speaking at a given time.\n\nTwo others — “++[Subword regularization: an analysis of scalability and generalization for end-to-end automatic speech recognition](https://www.amazon.science/publications/subword-regularization-an-analysis-of-scalability-and-generalization-for-end-to-end-automatic-speech-recognition)++” and “++[Efficient minimum word error rate training of RNN-transducer for end-to-end speech recognition](https://www.amazon.science/publications/efficient-minimum-word-error-rate-training-of-rnn-transducer-for-end-to-end-speech-recognition)++” —examine ways to improve the quality of speech recognizers that use an architecture know as a recurrent neural network-transducer, or RNN-T.\n\nIn his keynote address this week at Interspeech, Alexa director of ASR Shehzad Mavawalla ++[highlighted](https://www.amazon.science/blog/alexas-new-speech-recognition-abilities-showcased-at-interspeech)++ both of these areas — speaker ID and the use of RNN-Ts for ASR — as ones in which the Alexa science team has made rapid strides in recent years.\n\n\n#### **Speaker ID**\n\n\nSpeaker ID systems — which enable voice agents to personalize content to particular customers — typically rely on either recurrent neural networks or convolutional neural networks, both of which are able to track consistencies in the speech signal over short spans of time. \n\nIn “++[Speaker identification for household scenarios with self-attention and adversarial training](https://www.amazon.science/publications/speaker-identification-for-household-scenarios-with-self-attention-and-adversarial-training)++”, Amazon applied scientist Ruirui Li and colleagues at Amazon, the University of California, Los Angeles, and the University of Notre Dame instead use an attention mechanism to identify longer-range consistencies in the speech signal.\n\nIn neural networks — such as speech processors — that receive sequential inputs, attention mechanisms determine which other elements of the sequence should influence the network’s judgment about the current element. \n\nSpeech signals are typically divided into frames, which represent power concentrations at different sound frequencies over short spans of time. For a given utterance, Li and his colleagues’ model represents each frame as a weighted sum of itself and all the other frames in the utterance. The weights depend on correlations between the frequency characteristics of the frames; the greater the correlation, the greater the weight.\n\nThis representation has the advantage of capturing the distinctive properties of a speaker’s voice conveyed by each frame but suppressing accidental properties that are unique to individual frames and less characteristic of the speaker’s voice as a whole. \n\nThese representations pass to a neural network that, during training, learns which of these properties are the best indicators of a speaker’s identity. Finally, the sequential outputs of this network — one for each frame — are averaged together to produce a snapshot of the utterance as a whole. These snapshots are compared to stored profiles to determine the speaker’s identity.\n\nLi and his colleagues also used a few other tricks to make their system more reliable, such as adversarial training.\n\nIn tests, the researchers compared their system to four prior systems and found that its speaker identifications were more accurate across the board. Compared to the best-performing of the four baselines, the system reduced the identification error rate by about 12% on speakers whose utterances were included in the model training data and by about 30% on newly encountered speakers.\n\n\n#### **The RNN-T architecture**\n\n\nAnother pair of papers examine ways to improve the quality of speech recognizers that use the increasingly popular recurrent-neural-network-transducer architecture, or RNN-T. An RNN-T processes a sequence of inputs in order, so that the output corresponding to each input factors in both the inputs and outputs that preceded it. \n\nIn the ASR application, the RNN-T takes in frames of an acoustic speech signal and outputs text — a sequence of subwords, or word components. For instance, the output corresponding to the spoken word “subword” might be the subwords “sub” and “_word”. \n\n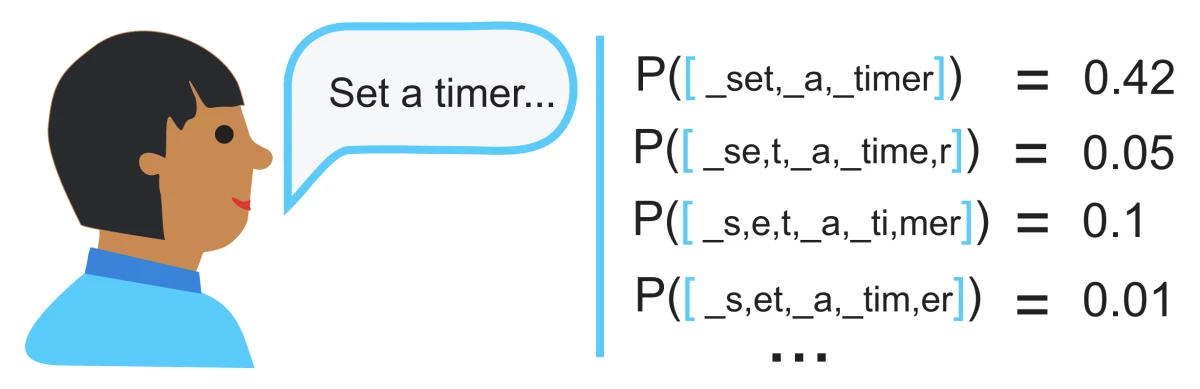\n\nA series of possible subword segmentations of the speech input, with the probability of each.\n\nFROM “SUBWORD REGULARIZATION: AN ANALYSIS OF SCALABILITY AND GENERALIZATION FOR END-TO-END AUTOMATIC SPEECH RECOGNITION”\n\nTraining the model to output subwords keeps the network size small. It also enables the model to deal with unfamiliar inputs, which it may be able to break into familiar components.\n\nIn the RNN-T architecture we consider, the input at time t — the current frame of the input speech — passes to an encoder network, which extracts acoustic features useful for speech recognition. At the same time, the current, incomplete sequence of output subwords passes to a prediction network, whose output indicates likely semantic properties of the next subword in the sequence.\n\nThese two representations — the encoding of the current frame and the likely semantic properties of the next subword — pass to another network, which on the basis of both representations determines the next word in the output sequence.\n\n\n#### **New wrinkles**\n\n\n“++[Subword regularization: an analysis of scalability and generalization for end-to-end automatic speech recognition](https://www.amazon.science/publications/subword-regularization-an-analysis-of-scalability-and-generalization-for-end-to-end-automatic-speech-recognition)++”, by applied scientist Egor Lakomkin and his Amazon colleagues, investigates the regularization of subwords in the model, or the enforcement of greater consistency in how words are segmented into subwords. In experiments, the researchers show that using multiple segmentations of the same speech transcription during training can reduce the ASR error rate by 8.4% in a model trained on 5,000 hours of speech data.\n\n“++[Efficient minimum word error rate training of RNN-transducer for end-to-end speech recognition](https://www.amazon.science/publications/efficient-minimum-word-error-rate-training-of-rnn-transducer-for-end-to-end-speech-recognition)++”, by applied scientist Jinxi Guo and six of his Amazon colleagues, investigates a novel loss function — an evaluation criterion during training — for such RNN-T ASR systems. In experiments, it reduced the systems’ error rates by 3.6% to 9.2%.\n\nFor each input, RNN-Ts output multiple possible solutions — or hypotheses — ranked according to probability. In ASR applications, RNN-Ts are typically trained to maximize the probabilities they assign the correct transcriptions of the input speech.\n\nBut trained speech recognizers are judged, by contrast, according to their word error rates, or the rate at which they make mistakes — misinterpretations, omissions, or erroneous insertions. Jinxi Guo and his colleagues investigated efficient ways to directly train an RNN-T ASR system to minimize word error rate.\n\nThat means, for each training example, minimizing the expected word errors of the most probable hypotheses. But computing the probabilities of those hypotheses isn’t as straightforward as it may sound.\n\nThat’s because the exact same sequence of output subwords can align with the sequence of input frames in different ways: one output sequence, for instance, might identify the same subword as having begun one frame earlier or later than another output sequence does. Computing the probability of a hypothesis requires summing the probabilities of all its alignments.\n\nThe brute-force solution to this problem would be computationally impractical. But Guo and his colleagues propose using the forward-backward algorithm, which exploits the overlaps between alignments, storing intermediate computations that can be re-used. The result is a computationally efficient algorithm that enables a 3.6% to 9.2% reduction in error rates for various RNN-T models.\n\nThe other Amazon ASR papers at this year’s Interspeech are\n\n**++[DiPCo - Dinner Party Corpus](https://www.amazon.science/publications/dipco-dinner-party-corpus)++**\nMaarten Van Segbroeck, Zaid Ahmed, Ksenia Kutsenko, Cirenia Huerta, Tinh Nguyen, Björn Hoffmeister, Jan Trmal, Maurizio Omologo, Roland Maas\n\n**++[End-to-end neural transformer based spoken language understanding](https://www.amazon.science/publications/end-to-end-neural-transformer-based-spoken-language-understanding)++**\nMartin Radfar, Athanasios Mouchtaris, Siegfried Kunzmann\n\n**++[Improving speech recognition of compound-rich languages](https://www.amazon.science/publications/improving-speech-recognition-of-compound-rich-languages)++**\nPrabhat Pandey, Volker Leutnant, Simon Wiesler, Jahn Heymann, Daniel Willett\n\n**++[Improved training strategies for end-to-end speech recognition in digital voice assistants](https://www.amazon.science/publications/improved-training-strategies-for-end-to-end-speech-recognition-in-digital-voice-assistants)++**\nHitesh Tulsiani, Ashtosh Sapru, Harish Arsikere, Surabhi Punjabi, Sri Garimella\n\n**++[Leveraging unlabeled speech for sequence discriminative training of acoustic models](https://www.amazon.science/publications/leveraging-unlabeled-speech-for-sequence-discriminative-training-of-acoustic-models)++**\nAshtosh Sapru, Sri Garimella\n\n**++[Quantization aware training with absolute-cosine regularization for automatic speech recognition](https://www.amazon.science/publications/quantization-aware-training-with-absolute-cosine-regularization-for-automatic-speech-recognition)++**\nHieu Duy Nguyen, Anastasios Alexandridis, Athanasios Mouchtaris\n\n**++[Rescore in a flash: Compact, cache efficient hashing data structures for N-gram language models](https://www.amazon.science/publications/rescore-in-a-flash-compact-cache-efficient-hashing-data-structures-for-n-gram-language-models)++**\nGrant P. Strimel, Ariya Rastrow, Gautam Tiwari, Adrien Pierard, Jon Webb\n\n**++[Semantic complexity in end-to-end spoken language understanding](https://www.amazon.science/publications/semantic-complexity-in-end-to-end-spoken-language-understanding)++**\nJoseph McKenna, Samridhi Choudhary, Michael Saxon, Grant P. Strimel, Athanasios Mouchtaris\n\n**++[Speech to semantics: Improve ASR and NLU jointly via all-neural interfaces](https://www.amazon.science/publications/speech-to-semantics-improve-asr-and-nlu-jointly-via-all-neural-interfaces)++**\nMilind Rao, Anirudh Raju, Pranav Dheram, Bach Bui, Ariya Rastrow\n\nABOUT THE AUTHOR\n\n#### **[Bjorn Hoffmeister](https://www.amazon.science/author/bjorn-hoffmeister)**\n\nBjörn Hoffmeister is the director of applied science for Alexa Speech.","render":"<p>As the largest conference devoted to speech technologies, Interspeech has long been a showcase for the latest research on automatic speech recognition (ASR) from Amazon Alexa. This year, Alexa researchers had 12 ASR papers accepted at the conference.</p>\n<p><img src=\\"https://dev-media.amazoncloud.cn/2d4e6d88ac2c40058ab3873bd7c7255c_image.png\\" alt=\\"image.png\\" /></p>\n<p>The architecture of the RNN-T ASR system. Xt indicates the current frame of the acoustic signal. Yu-1 indicates the sequence of output subwords corresponding to the preceding frames.</p>\n<p>FROM “EFFICIENT MINIMUM WORD ERROR RATE TRAINING OF RNN-TRANSDUCER FOR END-TO-END SPEECH RECOGNITION”</p>\n<p>One of these, “<ins><a href=\\"https://www.amazon.science/publications/speaker-identification-for-household-scenarios-with-self-attention-and-adversarial-training\\" target=\\"_blank\\">Speaker identification for household scenarios with self-attention and adversarial training</a></ins>”, reports the speech team’s recent innovations in speaker ID, or recognizing which of several possible speakers is speaking at a given time.</p>\n<p>Two others — “<ins><a href=\\"https://www.amazon.science/publications/subword-regularization-an-analysis-of-scalability-and-generalization-for-end-to-end-automatic-speech-recognition\\" target=\\"_blank\\">Subword regularization: an analysis of scalability and generalization for end-to-end automatic speech recognition</a></ins>” and “<ins><a href=\\"https://www.amazon.science/publications/efficient-minimum-word-error-rate-training-of-rnn-transducer-for-end-to-end-speech-recognition\\" target=\\"_blank\\">Efficient minimum word error rate training of RNN-transducer for end-to-end speech recognition</a></ins>” —examine ways to improve the quality of speech recognizers that use an architecture know as a recurrent neural network-transducer, or RNN-T.</p>\n<p>In his keynote address this week at Interspeech, Alexa director of ASR Shehzad Mavawalla <ins><a href=\\"https://www.amazon.science/blog/alexas-new-speech-recognition-abilities-showcased-at-interspeech\\" target=\\"_blank\\">highlighted</a></ins> both of these areas — speaker ID and the use of RNN-Ts for ASR — as ones in which the Alexa science team has made rapid strides in recent years.</p>\n<h4><a id=\\"Speaker_ID_15\\"></a><strong>Speaker ID</strong></h4>\\n<p>Speaker ID systems — which enable voice agents to personalize content to particular customers — typically rely on either recurrent neural networks or convolutional neural networks, both of which are able to track consistencies in the speech signal over short spans of time.</p>\n<p>In “<ins><a href=\\"https://www.amazon.science/publications/speaker-identification-for-household-scenarios-with-self-attention-and-adversarial-training\\" target=\\"_blank\\">Speaker identification for household scenarios with self-attention and adversarial training</a></ins>”, Amazon applied scientist Ruirui Li and colleagues at Amazon, the University of California, Los Angeles, and the University of Notre Dame instead use an attention mechanism to identify longer-range consistencies in the speech signal.</p>\n<p>In neural networks — such as speech processors — that receive sequential inputs, attention mechanisms determine which other elements of the sequence should influence the network’s judgment about the current element.</p>\n<p>Speech signals are typically divided into frames, which represent power concentrations at different sound frequencies over short spans of time. For a given utterance, Li and his colleagues’ model represents each frame as a weighted sum of itself and all the other frames in the utterance. The weights depend on correlations between the frequency characteristics of the frames; the greater the correlation, the greater the weight.</p>\n<p>This representation has the advantage of capturing the distinctive properties of a speaker’s voice conveyed by each frame but suppressing accidental properties that are unique to individual frames and less characteristic of the speaker’s voice as a whole.</p>\n<p>These representations pass to a neural network that, during training, learns which of these properties are the best indicators of a speaker’s identity. Finally, the sequential outputs of this network — one for each frame — are averaged together to produce a snapshot of the utterance as a whole. These snapshots are compared to stored profiles to determine the speaker’s identity.</p>\n<p>Li and his colleagues also used a few other tricks to make their system more reliable, such as adversarial training.</p>\n<p>In tests, the researchers compared their system to four prior systems and found that its speaker identifications were more accurate across the board. Compared to the best-performing of the four baselines, the system reduced the identification error rate by about 12% on speakers whose utterances were included in the model training data and by about 30% on newly encountered speakers.</p>\n<h4><a id=\\"The_RNNT_architecture_35\\"></a><strong>The RNN-T architecture</strong></h4>\\n<p>Another pair of papers examine ways to improve the quality of speech recognizers that use the increasingly popular recurrent-neural-network-transducer architecture, or RNN-T. An RNN-T processes a sequence of inputs in order, so that the output corresponding to each input factors in both the inputs and outputs that preceded it.</p>\n<p>In the ASR application, the RNN-T takes in frames of an acoustic speech signal and outputs text — a sequence of subwords, or word components. For instance, the output corresponding to the spoken word “subword” might be the subwords “sub” and “_word”.</p>\n<p><img src=\\"https://dev-media.amazoncloud.cn/96ed6026bb42490cb2c1e0544399a55e_image.png\\" alt=\\"image.png\\" /></p>\n<p>A series of possible subword segmentations of the speech input, with the probability of each.</p>\n<p>FROM “SUBWORD REGULARIZATION: AN ANALYSIS OF SCALABILITY AND GENERALIZATION FOR END-TO-END AUTOMATIC SPEECH RECOGNITION”</p>\n<p>Training the model to output subwords keeps the network size small. It also enables the model to deal with unfamiliar inputs, which it may be able to break into familiar components.</p>\n<p>In the RNN-T architecture we consider, the input at time t — the current frame of the input speech — passes to an encoder network, which extracts acoustic features useful for speech recognition. At the same time, the current, incomplete sequence of output subwords passes to a prediction network, whose output indicates likely semantic properties of the next subword in the sequence.</p>\n<p>These two representations — the encoding of the current frame and the likely semantic properties of the next subword — pass to another network, which on the basis of both representations determines the next word in the output sequence.</p>\n<h4><a id=\\"New_wrinkles_55\\"></a><strong>New wrinkles</strong></h4>\\n<p>“<ins><a href=\\"https://www.amazon.science/publications/subword-regularization-an-analysis-of-scalability-and-generalization-for-end-to-end-automatic-speech-recognition\\" target=\\"_blank\\">Subword regularization: an analysis of scalability and generalization for end-to-end automatic speech recognition</a></ins>”, by applied scientist Egor Lakomkin and his Amazon colleagues, investigates the regularization of subwords in the model, or the enforcement of greater consistency in how words are segmented into subwords. In experiments, the researchers show that using multiple segmentations of the same speech transcription during training can reduce the ASR error rate by 8.4% in a model trained on 5,000 hours of speech data.</p>\n<p>“<ins><a href=\\"https://www.amazon.science/publications/efficient-minimum-word-error-rate-training-of-rnn-transducer-for-end-to-end-speech-recognition\\" target=\\"_blank\\">Efficient minimum word error rate training of RNN-transducer for end-to-end speech recognition</a></ins>”, by applied scientist Jinxi Guo and six of his Amazon colleagues, investigates a novel loss function — an evaluation criterion during training — for such RNN-T ASR systems. In experiments, it reduced the systems’ error rates by 3.6% to 9.2%.</p>\n<p>For each input, RNN-Ts output multiple possible solutions — or hypotheses — ranked according to probability. In ASR applications, RNN-Ts are typically trained to maximize the probabilities they assign the correct transcriptions of the input speech.</p>\n<p>But trained speech recognizers are judged, by contrast, according to their word error rates, or the rate at which they make mistakes — misinterpretations, omissions, or erroneous insertions. Jinxi Guo and his colleagues investigated efficient ways to directly train an RNN-T ASR system to minimize word error rate.</p>\n<p>That means, for each training example, minimizing the expected word errors of the most probable hypotheses. But computing the probabilities of those hypotheses isn’t as straightforward as it may sound.</p>\n<p>That’s because the exact same sequence of output subwords can align with the sequence of input frames in different ways: one output sequence, for instance, might identify the same subword as having begun one frame earlier or later than another output sequence does. Computing the probability of a hypothesis requires summing the probabilities of all its alignments.</p>\n<p>The brute-force solution to this problem would be computationally impractical. But Guo and his colleagues propose using the forward-backward algorithm, which exploits the overlaps between alignments, storing intermediate computations that can be re-used. The result is a computationally efficient algorithm that enables a 3.6% to 9.2% reduction in error rates for various RNN-T models.</p>\n<p>The other Amazon ASR papers at this year’s Interspeech are</p>\n<p><strong><ins><a href=\\"https://www.amazon.science/publications/dipco-dinner-party-corpus\\" target=\\"_blank\\">DiPCo - Dinner Party Corpus</a></ins></strong><br />\\nMaarten Van Segbroeck, Zaid Ahmed, Ksenia Kutsenko, Cirenia Huerta, Tinh Nguyen, Björn Hoffmeister, Jan Trmal, Maurizio Omologo, Roland Maas</p>\n<p><strong><ins><a href=\\"https://www.amazon.science/publications/end-to-end-neural-transformer-based-spoken-language-understanding\\" target=\\"_blank\\">End-to-end neural transformer based spoken language understanding</a></ins></strong><br />\\nMartin Radfar, Athanasios Mouchtaris, Siegfried Kunzmann</p>\n<p><strong><ins><a href=\\"https://www.amazon.science/publications/improving-speech-recognition-of-compound-rich-languages\\" target=\\"_blank\\">Improving speech recognition of compound-rich languages</a></ins></strong><br />\\nPrabhat Pandey, Volker Leutnant, Simon Wiesler, Jahn Heymann, Daniel Willett</p>\n<p><strong><ins><a href=\\"https://www.amazon.science/publications/improved-training-strategies-for-end-to-end-speech-recognition-in-digital-voice-assistants\\" target=\\"_blank\\">Improved training strategies for end-to-end speech recognition in digital voice assistants</a></ins></strong><br />\\nHitesh Tulsiani, Ashtosh Sapru, Harish Arsikere, Surabhi Punjabi, Sri Garimella</p>\n<p><strong><ins><a href=\\"https://www.amazon.science/publications/leveraging-unlabeled-speech-for-sequence-discriminative-training-of-acoustic-models\\" target=\\"_blank\\">Leveraging unlabeled speech for sequence discriminative training of acoustic models</a></ins></strong><br />\\nAshtosh Sapru, Sri Garimella</p>\n<p><strong><ins><a href=\\"https://www.amazon.science/publications/quantization-aware-training-with-absolute-cosine-regularization-for-automatic-speech-recognition\\" target=\\"_blank\\">Quantization aware training with absolute-cosine regularization for automatic speech recognition</a></ins></strong><br />\\nHieu Duy Nguyen, Anastasios Alexandridis, Athanasios Mouchtaris</p>\n<p><strong><ins><a href=\\"https://www.amazon.science/publications/rescore-in-a-flash-compact-cache-efficient-hashing-data-structures-for-n-gram-language-models\\" target=\\"_blank\\">Rescore in a flash: Compact, cache efficient hashing data structures for N-gram language models</a></ins></strong><br />\\nGrant P. Strimel, Ariya Rastrow, Gautam Tiwari, Adrien Pierard, Jon Webb</p>\n<p><strong><ins><a href=\\"https://www.amazon.science/publications/semantic-complexity-in-end-to-end-spoken-language-understanding\\" target=\\"_blank\\">Semantic complexity in end-to-end spoken language understanding</a></ins></strong><br />\\nJoseph McKenna, Samridhi Choudhary, Michael Saxon, Grant P. Strimel, Athanasios Mouchtaris</p>\n<p><strong><ins><a href=\\"https://www.amazon.science/publications/speech-to-semantics-improve-asr-and-nlu-jointly-via-all-neural-interfaces\\" target=\\"_blank\\">Speech to semantics: Improve ASR and NLU jointly via all-neural interfaces</a></ins></strong><br />\\nMilind Rao, Anirudh Raju, Pranav Dheram, Bach Bui, Ariya Rastrow</p>\n<p>ABOUT THE AUTHOR</p>\n<h4><a id=\\"Bjorn_Hoffmeisterhttpswwwamazonscienceauthorbjornhoffmeister_103\\"></a><strong><a href=\\"https://www.amazon.science/author/bjorn-hoffmeister\\" target=\\"_blank\\">Bjorn Hoffmeister</a></strong></h4>\n<p>Björn Hoffmeister is the director of applied science for Alexa Speech.</p>\n"}