{"value":"he annual meeting of the ACM Special Interest Group on Information Retrieval (SIGIR) begins next week, and Barbara Poblete, an [Amazon Visiting Academic](https://www.amazon.science/visiting-academics) and associate professor of computer science at the University of Chile, is a cochair of both the Doctoral Consortium and the Diversity, Equity and Inclusion Committee.\n\n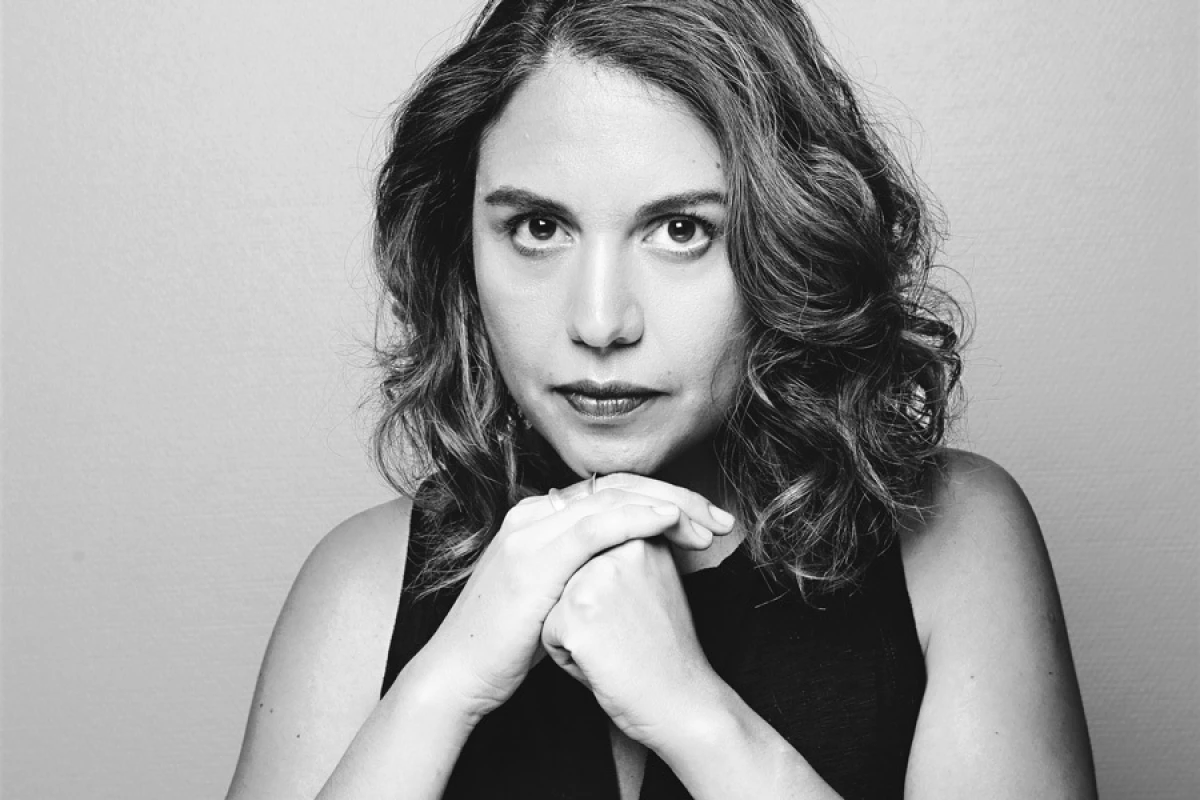\n\nBarbara Poblete, an associate professor of computer science at the University of Chile, and an Amazon Visiting Academic\n\nPoblete, who first attended the conference as a graduate student in 2006, has been a member of the Diversity, Equity, and Inclusion (DEI) Committee since its creation in 2019.\n\n“It’s critical for the SIGIR conferences and the SIGIR community as a whole to be as inclusive as possible,” she says. “Everybody should feel welcome and be treated with respect and dignity. The cochairs represent different communities: I have worked on a lot of women-in-computer-science initiatives — [Chile Women in Computing](https://chilewic.cl/) is an event I organize — and I also represent South America. My two cochairs have experience in diversity-related initiatives in South Africa and Europe. We have created a set of guidelines for the SIGIR conferences, which is shared with the organizers. This ‘inclusivity checklist’, as we call it, helps make these conferences more inclusive.”\n\n#### **Hate speech detection**\n\nPoblete was a natural choice for SIGIR’s DEI Committee as much of her research focuses on extending the benefits of machine learning to new communities and making members of online communities feel safer and more welcome.\n\n“I work in hate speech detection, and I have been focusing on the multilingual aspect because we have found that prior work is mostly centered on the English language,” she says. “This creates a gap for South America and for other countries where English is not the primary language.”\n\nIn this context, Poblete says, the chief technical challenge is to leverage English-language resources in order to build models for non-English linguistic communities with comparatively little training data.\n\n“It's not always easy,” Poblete says. “For example, the hate speech detection problem varies from country to country. Even between my country, Chile, and Argentina, the hate speech vocabularies are different. It’s not just linguistic adaptation; there’s cultural adaptation as well.”\n\n#### **\"Even between my country, Chile, and Argentina, the hate speech vocabularies are different. It’s not just linguistic adaptation; there’s cultural adaptation as well.\"**\n\nThe natural way to try to adapt English-language models to other languages, Poblete explains, is to use multilingual embeddings, in which related words in different languages are mapped to the same regions of a representational space. But, she says, “they don’t usually work that well for this kind of problem.”\n\n“One thing we do is dataset enrichment,” Poblete says. “So, for example, I have my dataset in Spanish, and I will add labeled English data to that to see if I can improve my classifier by adding the multilingual data. Or we try to create specific embeddings for certain domains, such as hate speech. Or we try to train embeddings that are biased towards a particular kind of problem.”\n\n#### **Disaster detection**\n\nPoblete is well known for her work on social-media analysis: at [the Web Conference 2021](https://www.amazon.science/conferences-and-events/the-web-conference-2021), in April, for instance, she and her colleagues won a test-of-time award for their 2011 paper “[Information credibility on Twitter](https://dl.acm.org/doi/10.1145/1963405.1963500)”. In more recent work, her group at the University of Chile has begun to apply techniques of social-media analysis to problems in the field of crisis informatics\n\n“We use social-media data to try to improve tools for disaster detection and collection of information,” Poblete explains. “Earthquakes and floods happen a lot in Chile, so there's a lot of interest in that. And it's kind of a similar problem: how can we use resources from other languages for our language? How can we create universal tools that anybody could use, that don't require a lot of resources?”\n\nPoblete’s group has developed a website, called twicalli.cl, that uses machine learning models to automatically process tweets in order to gauge the perceived intensity of earthquakes.\n\n“This is used by the National Seismology Center here in Chile,” Poblete says. “It’s used also by the navy, and a lot of emergency offices depend on this. We have a lot of seismographs in Chile — this is a very advanced field in Chile — but they cannot really tell how people felt the earthquake. This is important information because you could have two earthquakes with the same magnitude in different places, but they will be felt differently depending on how deep the earthquake was or the kind of terrain. \n\n“For areas where you have a large population, and they're tweeting, we can estimate that in 30 minutes. And that used to sometimes take days and require experts to be in the place where the earthquake happened. When you're in crisis management, the first minutes are super important. The information you gather in these first minutes will change how you respond to the emergency and how fast help will arrive.”\n\nPoblete is speaking from her home in Santiago, using Amazon’s Chime videoconferencing service, and suddenly, the onscreen image of her home office begins to jitter.\n\n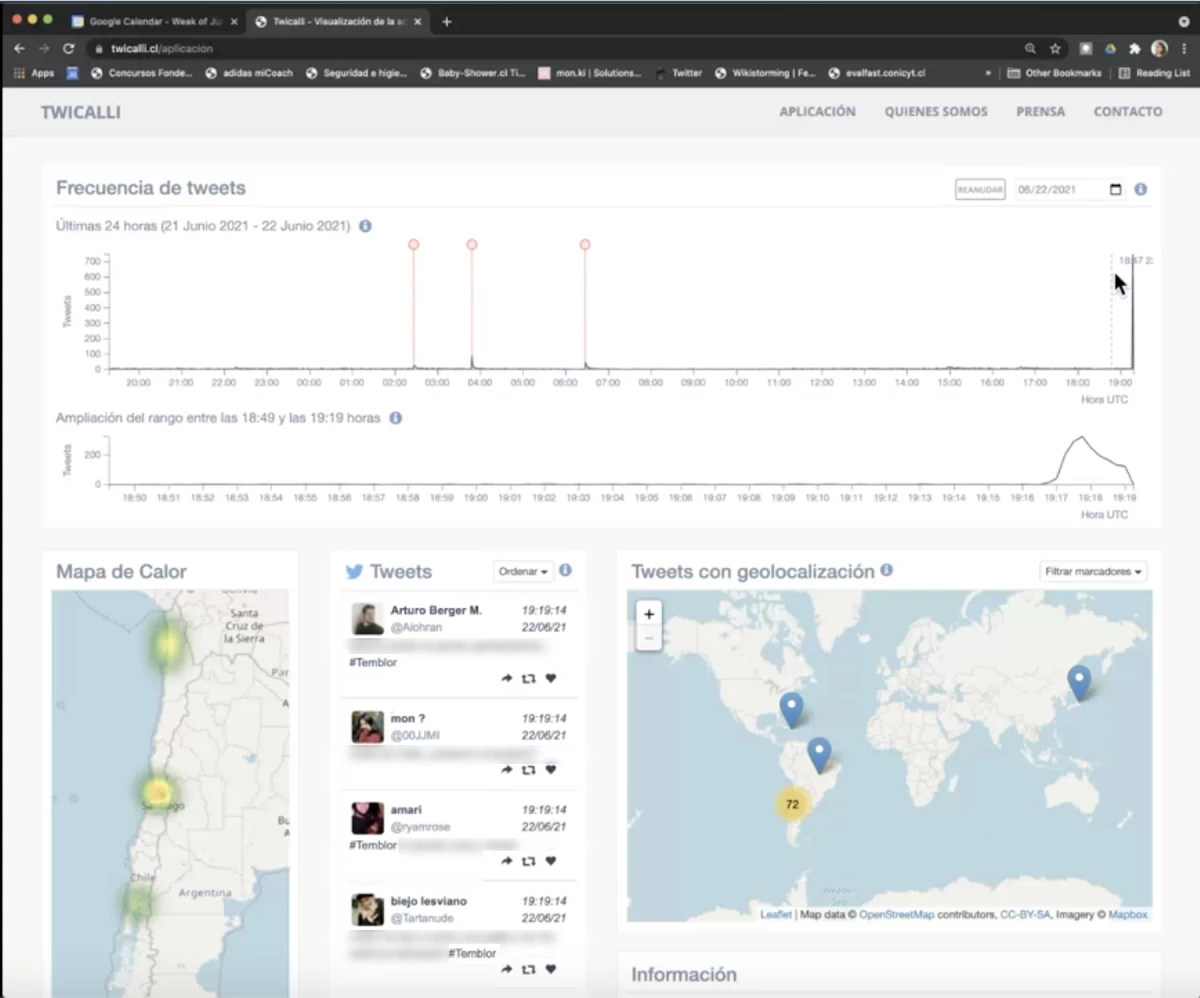\n\nBarbara Poblete’s real-time screen share, using [Amazon Chime](https://aws.amazon.com/cn/chime/?trk=cndc-detail), of the twicalli.cl dashboard. The top timeline indicates the frequency of tweets that use earthquake-related language; the lower timeline indicates a zoom-in of the most recent activity.\n\n“Oh look, there's an earthquake here right now,” she says. “Let me just find twicalli to see if it actually detected this.” She shares her screen through Chime. “This is the seismograph, and these are earthquakes we had before. In this portion of the graph, you can see tweets spike with people talking about this. That way, people at the seismology center know that people actually felt this earthquake.”\n\n“The problem we're working on right now,” Poblete says, “is to detect messages that are relevant to a crisis versus those that are noise. You want to separate the messages that are coming from the place of the event from other people who are just mentioning these things. When you have a hashtag that is popular, like the Nepal earthquake, you get a lot of messages that have nothing to do with it that are just mentioning the same hashtag. To tell those two things apart, we train disaster-specific word embeddings for that problem. And we're testing if we can enhance the information that we have in Spanish for earthquakes in Chile with earthquakes from other countries. And not only cross-language learning but also cross-domain. Can I can learn from earthquakes to detect hurricanes or floods or something new that never happened before? Because that is also part of emergency preparedness.”","render":"<p>he annual meeting of the ACM Special Interest Group on Information Retrieval (SIGIR) begins next week, and Barbara Poblete, an <a href=\\"https://www.amazon.science/visiting-academics\\" target=\\"_blank\\">Amazon Visiting Academic</a> and associate professor of computer science at the University of Chile, is a cochair of both the Doctoral Consortium and the Diversity, Equity and Inclusion Committee.</p>\\n<p><img src=\\"https://dev-media.amazoncloud.cn/4056655bf22e441ab8be61f172b04321_image.png\\" alt=\\"image.png\\" /></p>\n<p>Barbara Poblete, an associate professor of computer science at the University of Chile, and an Amazon Visiting Academic</p>\n<p>Poblete, who first attended the conference as a graduate student in 2006, has been a member of the Diversity, Equity, and Inclusion (DEI) Committee since its creation in 2019.</p>\n<p>“It’s critical for the SIGIR conferences and the SIGIR community as a whole to be as inclusive as possible,” she says. “Everybody should feel welcome and be treated with respect and dignity. The cochairs represent different communities: I have worked on a lot of women-in-computer-science initiatives — <a href=\\"https://chilewic.cl/\\" target=\\"_blank\\">Chile Women in Computing</a> is an event I organize — and I also represent South America. My two cochairs have experience in diversity-related initiatives in South Africa and Europe. We have created a set of guidelines for the SIGIR conferences, which is shared with the organizers. This ‘inclusivity checklist’, as we call it, helps make these conferences more inclusive.”</p>\\n<h4><a id=\\"Hate_speech_detection_10\\"></a><strong>Hate speech detection</strong></h4>\\n<p>Poblete was a natural choice for SIGIR’s DEI Committee as much of her research focuses on extending the benefits of machine learning to new communities and making members of online communities feel safer and more welcome.</p>\n<p>“I work in hate speech detection, and I have been focusing on the multilingual aspect because we have found that prior work is mostly centered on the English language,” she says. “This creates a gap for South America and for other countries where English is not the primary language.”</p>\n<p>In this context, Poblete says, the chief technical challenge is to leverage English-language resources in order to build models for non-English linguistic communities with comparatively little training data.</p>\n<p>“It’s not always easy,” Poblete says. “For example, the hate speech detection problem varies from country to country. Even between my country, Chile, and Argentina, the hate speech vocabularies are different. It’s not just linguistic adaptation; there’s cultural adaptation as well.”</p>\n<h4><a id=\\"Even_between_my_country_Chile_and_Argentina_the_hate_speech_vocabularies_are_different_Its_not_just_linguistic_adaptation_theres_cultural_adaptation_as_well_20\\"></a><strong>"Even between my country, Chile, and Argentina, the hate speech vocabularies are different. It’s not just linguistic adaptation; there’s cultural adaptation as well."</strong></h4>\\n<p>The natural way to try to adapt English-language models to other languages, Poblete explains, is to use multilingual embeddings, in which related words in different languages are mapped to the same regions of a representational space. But, she says, “they don’t usually work that well for this kind of problem.”</p>\n<p>“One thing we do is dataset enrichment,” Poblete says. “So, for example, I have my dataset in Spanish, and I will add labeled English data to that to see if I can improve my classifier by adding the multilingual data. Or we try to create specific embeddings for certain domains, such as hate speech. Or we try to train embeddings that are biased towards a particular kind of problem.”</p>\n<h4><a id=\\"Disaster_detection_26\\"></a><strong>Disaster detection</strong></h4>\\n<p>Poblete is well known for her work on social-media analysis: at <a href=\\"https://www.amazon.science/conferences-and-events/the-web-conference-2021\\" target=\\"_blank\\">the Web Conference 2021</a>, in April, for instance, she and her colleagues won a test-of-time award for their 2011 paper “<a href=\\"https://dl.acm.org/doi/10.1145/1963405.1963500\\" target=\\"_blank\\">Information credibility on Twitter</a>”. In more recent work, her group at the University of Chile has begun to apply techniques of social-media analysis to problems in the field of crisis informatics</p>\\n<p>“We use social-media data to try to improve tools for disaster detection and collection of information,” Poblete explains. “Earthquakes and floods happen a lot in Chile, so there’s a lot of interest in that. And it’s kind of a similar problem: how can we use resources from other languages for our language? How can we create universal tools that anybody could use, that don’t require a lot of resources?”</p>\n<p>Poblete’s group has developed a website, called twicalli.cl, that uses machine learning models to automatically process tweets in order to gauge the perceived intensity of earthquakes.</p>\n<p>“This is used by the National Seismology Center here in Chile,” Poblete says. “It’s used also by the navy, and a lot of emergency offices depend on this. We have a lot of seismographs in Chile — this is a very advanced field in Chile — but they cannot really tell how people felt the earthquake. This is important information because you could have two earthquakes with the same magnitude in different places, but they will be felt differently depending on how deep the earthquake was or the kind of terrain.</p>\n<p>“For areas where you have a large population, and they’re tweeting, we can estimate that in 30 minutes. And that used to sometimes take days and require experts to be in the place where the earthquake happened. When you’re in crisis management, the first minutes are super important. The information you gather in these first minutes will change how you respond to the emergency and how fast help will arrive.”</p>\n<p>Poblete is speaking from her home in Santiago, using Amazon’s Chime videoconferencing service, and suddenly, the onscreen image of her home office begins to jitter.</p>\n<p><img src=\\"https://dev-media.amazoncloud.cn/e68bc3433d7c4e52b432e1e70615bb62_image.png\\" alt=\\"image.png\\" /></p>\n<p>Barbara Poblete’s real-time screen share, using Amazon Chime, of the twicalli.cl dashboard. The top timeline indicates the frequency of tweets that use earthquake-related language; the lower timeline indicates a zoom-in of the most recent activity.</p>\n<p>“Oh look, there’s an earthquake here right now,” she says. “Let me just find twicalli to see if it actually detected this.” She shares her screen through Chime. “This is the seismograph, and these are earthquakes we had before. In this portion of the graph, you can see tweets spike with people talking about this. That way, people at the seismology center know that people actually felt this earthquake.”</p>\n<p>“The problem we’re working on right now,” Poblete says, “is to detect messages that are relevant to a crisis versus those that are noise. You want to separate the messages that are coming from the place of the event from other people who are just mentioning these things. When you have a hashtag that is popular, like the Nepal earthquake, you get a lot of messages that have nothing to do with it that are just mentioning the same hashtag. To tell those two things apart, we train disaster-specific word embeddings for that problem. And we’re testing if we can enhance the information that we have in Spanish for earthquakes in Chile with earthquakes from other countries. And not only cross-language learning but also cross-domain. Can I can learn from earthquakes to detect hurricanes or floods or something new that never happened before? Because that is also part of emergency preparedness.”</p>\n"}