{"value":"Graphs are an information-rich way to represent data. A graph consists of nodes — typically represented by circles — and edges — typically represented as line segments between nodes. In a knowledge graph, for instance, the nodes represent entities, and the edges represent relationships between them. In a social graph, the nodes represent people, and an edge indicates that two of those people know each other.\n\nAt Amazon Web Services, the use of machine learning (ML) to make the information encoded in graphs more useful to our customers has been a major research focus. In this post, we’ll showcase a variety of graph ML applications that customers have developed in collaboration with Amazon Web Services scientists, from malicious-account detection and automated document processing to knowledge-graph-assisted drug discovery and protein property prediction.\n\n#### **Introduction to graph learning**\n\nGraphs can be homogenous, meaning the nodes represent a single type of entity (say, airports), and the edges represent a single type of relationship (say, scheduled flights). Or they can be heterogeneous, meaning they integrate multiple types of relationships among different entities, such as a graph of customers and products connected by both purchase histories and interests, or a knowledge graph of drugs, diseases, genes, and biological pathways connected by relationships such as indication and regulation. Nodes are often associated with data features, such as a product’s price or text description.\n\n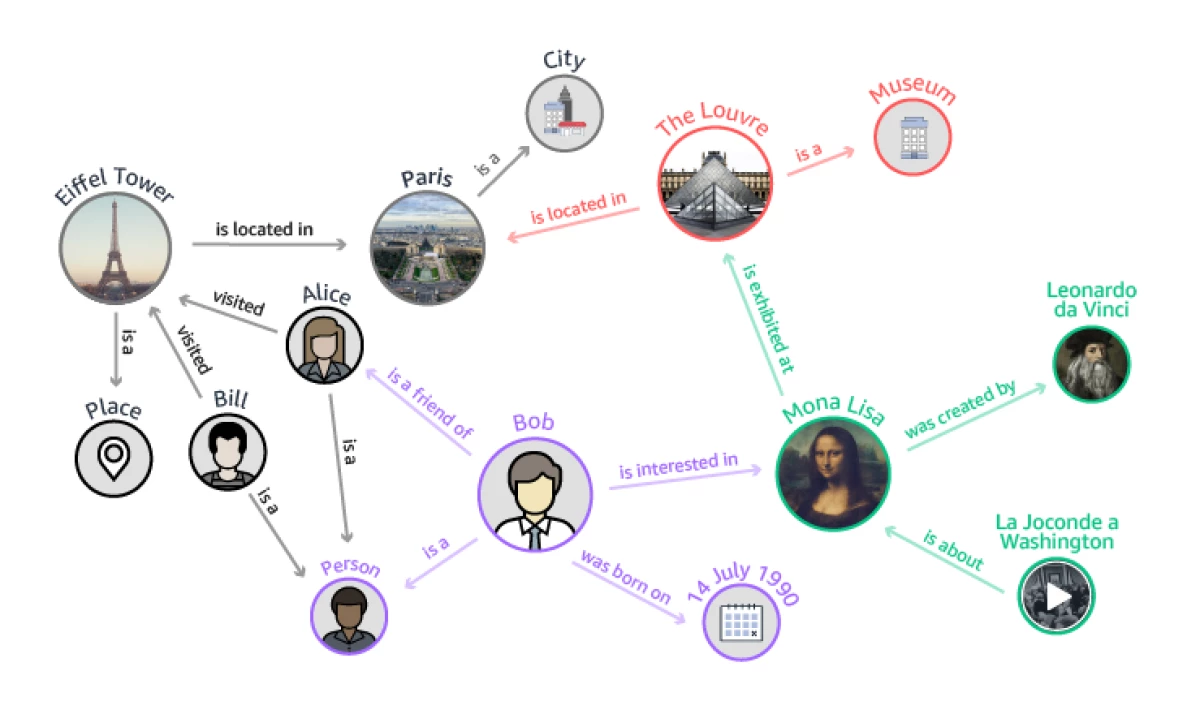\n\nIn a heterogenous knowledge graph, nodes can represent different classes of objects.\n\n#### **Graph neural networks**\n\nIn the past 10 years, deep learning has revolutionized a host of AI applications, from natural-language processing to speech synthesis to computer vision.\n\nGraph neural networks (GNNs) extend the performance benefits of deep learning to graph data. Like other popular neural networks, a GNN model has a series of layers, which progress toward higher levels of abstraction.\n\nFor instance, the first layer of a GNN computes a representation — or embedding — of the data represented by each node in the graph, while the second layer computes a representation of each node based on the prior embedding and the embeddings of the node’s nearest neighbors. In this way, every layer expands the scope of a node’s embedding, from one-hop neighbors, to two-hop neighbors, and for some applications, even further.\n\n\n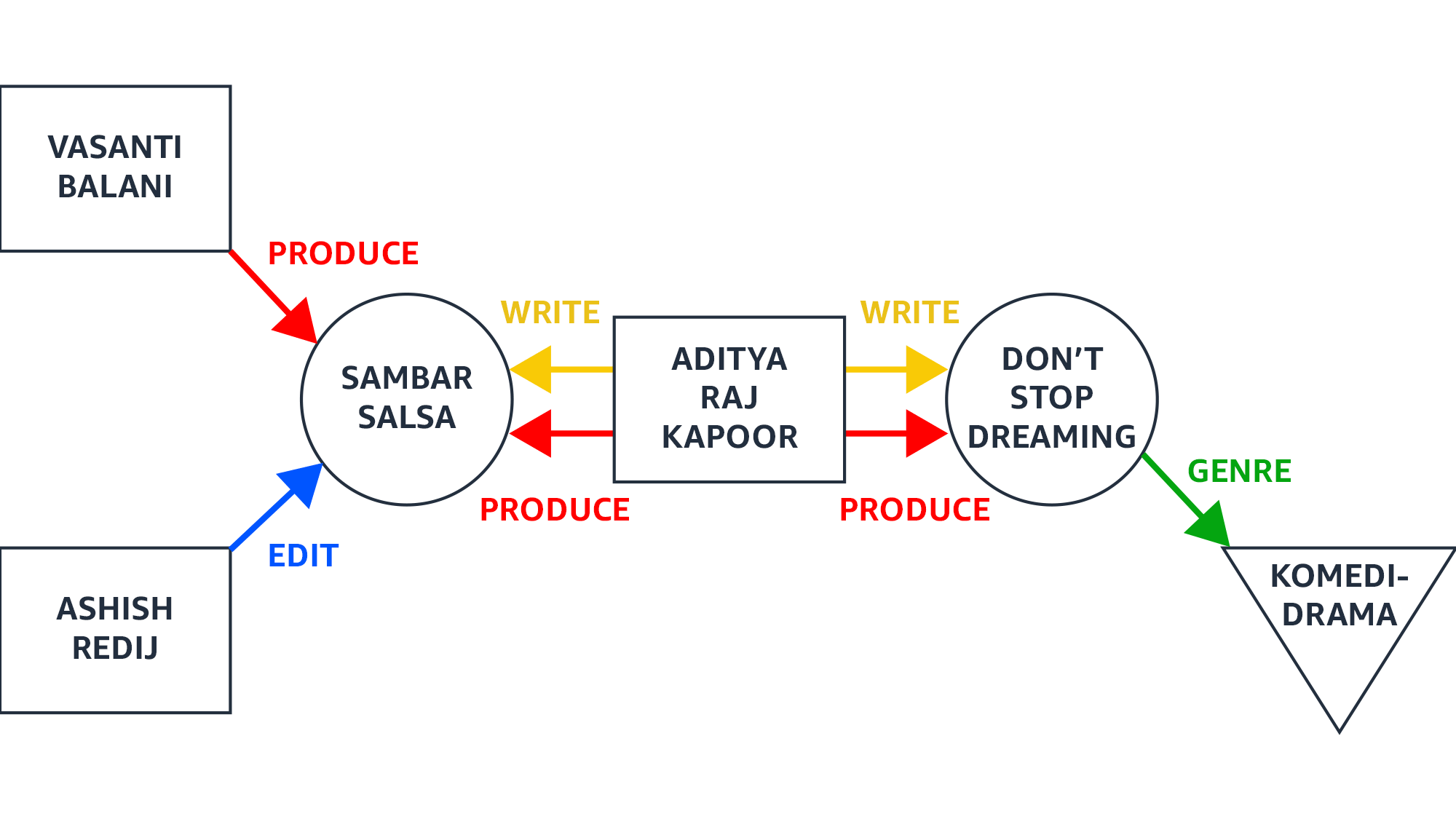\n\nA demonstration of how graph neural networks use recursive embedding to condense all the information in a two-hop graph into a single vector. Relationships between entities — such as \"produce\" and \"write\" in a movie database (red and yellow arrows, respectively) — are encoded in the level-0 embeddings of the entities themselves (red and orange blocks).\n\nSTACY REILLY\n\n#### **GNN tasks**\n\nThe individual node embeddings can then be used for node-level tasks, such as predicting properties of a node. The embeddings can also be used for higher-level inferences. For instance, using representations across a pair of nodes or across all nodes from the graph, GNNs can perform link-level or graph-level tasks, respectively.\n\nIn this section, we demonstrate the versatility of GNNs across all three levels of tasks and examine how our customers are using GNNs to tackle a variety of problems.\n\n#### **Node-level tasks**\n\nUsing GNNs, we can infer the behavior of an individual node in the graph based on the relationships it has to other nodes. One common task is node classification, where the objective is to infer nodes’ missing labels by looking at their neighbors’ labels and features. This method is used in applications such as financial-fraud detection, publication categorization, and disease classification.\n\nIn Amazon Web Services, we have successfully used ++[Amazon Neptune](https://aws.amazon.com/neptune/machine-learning/)++ and ++[Deep Graph Library (DGL)](https://www.dgl.ai/)++ to apply GNN node representation learning to customers’ fraud detection use cases. For a large e-commerce sports gadgets customer, for instance, scientists in the ++[Amazon Machine Learning Solutions Lab](https://aws.amazon.com/ml-solutions-lab/)++ successfully used GNN models implemented in DGL to detect malicious accounts among billions of registered accounts.\n\nThese malicious accounts were created in large quantities to abuse usage of promotional codes and block general public access to the vendor’s best-selling items. Using data from e-commerce sites, we built a massive heterogenous graph in which the nodes represented accounts and other entities, such as products purchased, and the edges connected nodes based on usage histories. To identify malicious accounts, we trained a GNN model to propagate labels from accounts that were known to be malicious to unlabeled accounts.\n\n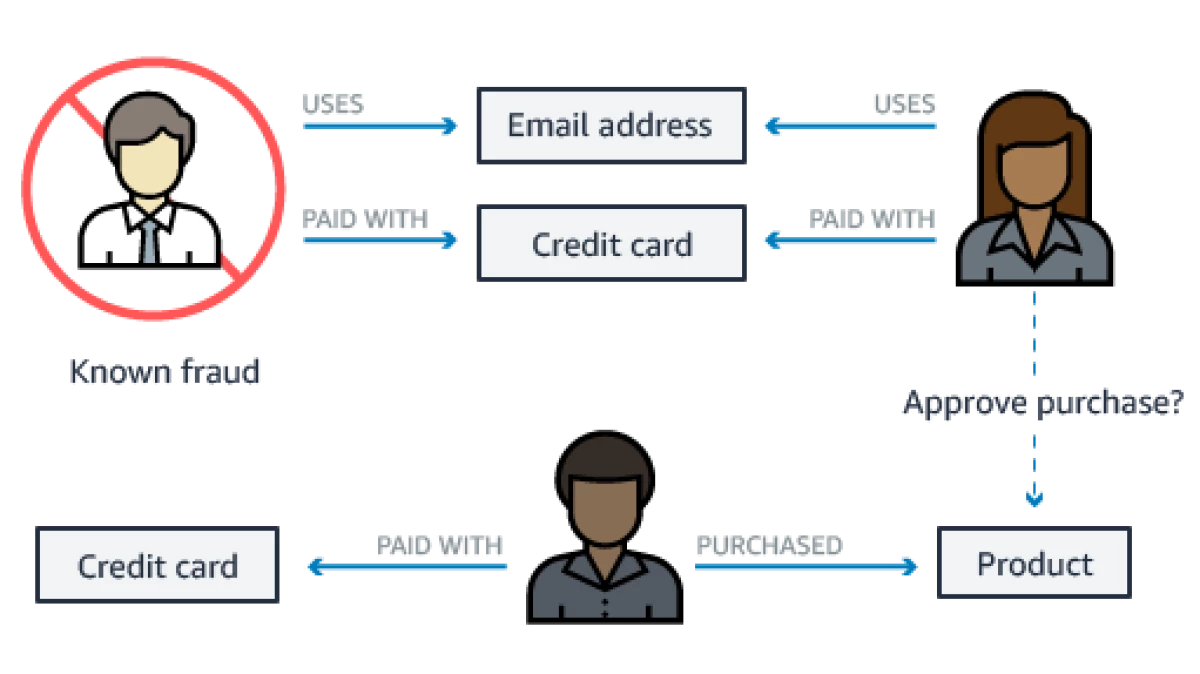\n\nAn example of how a graph representation can be used to detect fraud.\n\nWith this method, we were able to detect 10 times as many malicious accounts as a previous rule-based detection method could. Such performance improvements could not be achieved by traditional methods for doing machine learning on tabular datasets, such as CatBoost, which take only account features as inputs, without considering the relationships between accounts captured by the graph.\n\nBesides applications for inherently relational, graph-structured data, such as social-network and citation-network data, there have been extensions of GNNs for data normally presented in Euclidean space, such as images and texts. By transforming data in Euclidean space to graphs based on spatial proximity, GNNs can solve problems that are typically solved by convolutional neural networks (CNNs) and recurrent neural networks (RNNs), which were designed to handle visual data and sequential data.\n\nFor example, researchers have explored GNN models to improve the accuracy of information extraction, a task typically handled by RNNs. GNNs turn out to be better at incorporating the nonlocal and nonsequential relationships captured by graph representations of word dependencies.\n\nIn a recent ++[collaboration](https://aws.amazon.com/solutions/case-studies/united-airlines-2021-reinvent-video/)++, the Amazon Machine Learning Solutions Lab and United Airlines developed a customized GNN model (DocGCN) to improve the accuracy of automatic information extraction from self-uploaded passenger documents, including travel documents, COVID-19 test results, and vaccine cards. The team built a graph for each scanned travel document that connected textual units based on their spatial proximities and orientations in the document.\n\nThen, the DocGCN model reasoned over the relationships among textual units (nodes of the graph) to improve the identification of relevant textual information. DocGCN also generalized to complex forms with different formats by leveraging graphs to capture relationships between texts in tables, key-value pairs, and paragraphs. This improvement expedited the automation of international travel readiness verification.\n\n#### **Link-level tasks**\n\nAnother important learning task in graphs is link prediction, which is central to applications such as product or ad recommendation and friendship suggestion. Given two nodes and a relation, the goal is to determine whether the nodes are connected by the relation.\n\nTypically, the prediction is provided by a decoder that consumes the embeddings of the source and destination nodes, as in the work on ++[knowledge graph embedding at scale](https://www.amazon.science/blog/amazons-open-source-tools-make-embedding-knowledge-graphs-much-more-efficient)++ that members of our team presented at SIGIR 2020. The decoder is trained to correctly predict existing edges in the graph.\n\n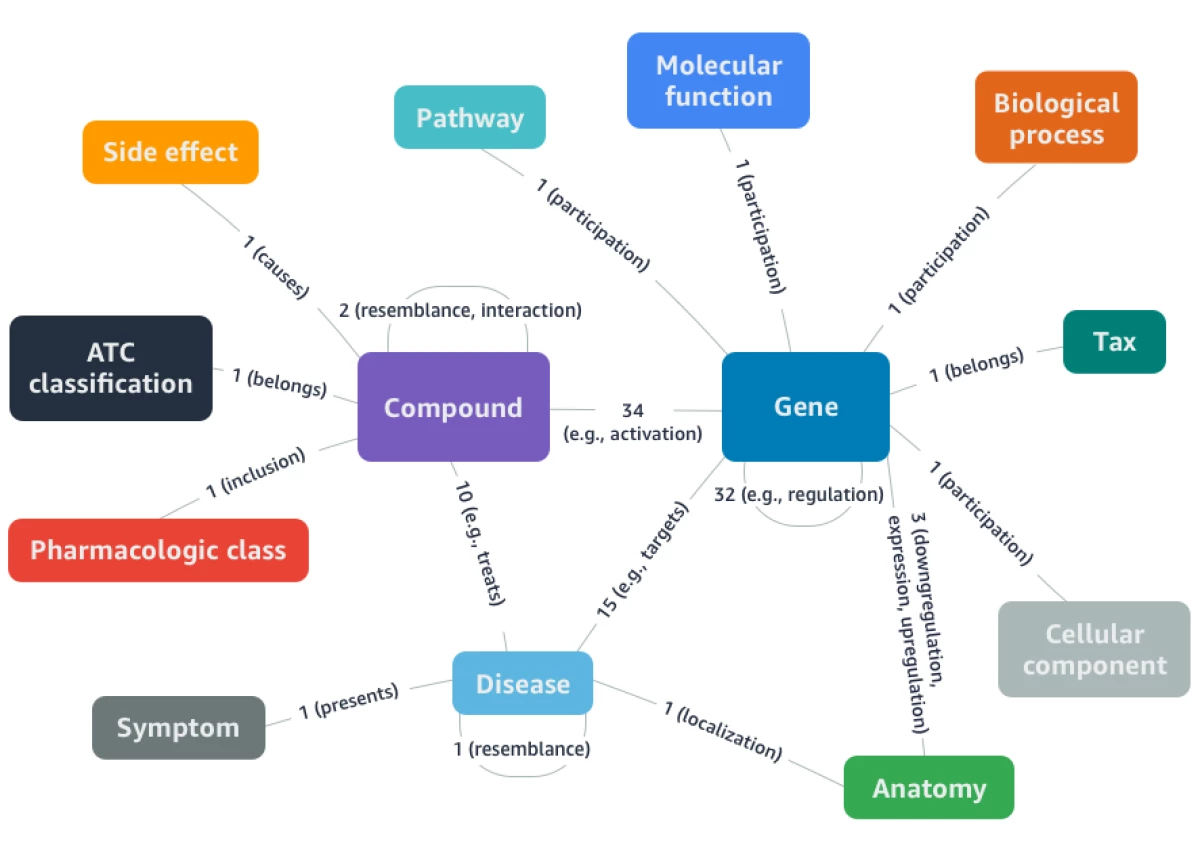\n\nThe high-level structure of DRKG. Numerals indicate the number of different types of relationships between classes of entities; terms between parentheses are examples of those relationships.\nCREDIT: GLYNIS CONDON\n\nAn exciting opportunity area in this context is drug discovery. Amazon Web Services has recently provided a drug-repurposing knowledge graph (++[DRKG](https://www.amazon.science/blog/amazon-web-services-open-sources-biological-knowledge-graph-to-fight-covid-19)++) that employs link prediction to identify new targets for existing drugs. Built by scientists at Amazon Web Services, DRKG is a comprehensive biological knowledge graph that relates human genes, chemical compounds, biological processes, drug side effects, diseases, and symptoms. By performing link prediction around COVID-19 in DRKG, researchers were able to identify 41 drugs that were potentially effective against COVID-19 — 11 of which were already in clinical trials.\n\nAmazon Web Services also publicly released this solution, built by leveraging DRKG, as the COVID-19 Knowledge Graph (CKG). CKG organizes and represents the information in the COVID-19 Open Research Dataset (++[CORD-19](https://www.semanticscholar.org/cord19)++), enabling fast discovery and prioritization of drug candidates. It can also be employed to identify ++[papers relevant to COVID-19](https://www.amazon.science/blog/using-knowledge-graphs-to-streamline-covid-19-research)++, thereby reducing the scale of human effort required to study, summarize, and interpret findings relevant to the pandemic.\n\n#### **Graph-level tasks**\n\nGraph-level tasks involve the analysis of large collections of small and independent graphs. A chemical library of organic compounds is a common example of a graph-level application, where each organic compound is represented as a graph of atoms connected by chemical bonds. Graph-level analyses of chemical libraries are often vital for drug development and discovery use cases; applications include predicting organic compounds’ chemical properties and predicting biological activities such as binding affinity to protein targets.\n\nAnother example of data that can benefit from graph-level representation is code snippets in programming languages. A piece of code can be represented by a program dependence graph (PDG), where variables, operators, and statements are nodes connected by their dependencies (links).\n\nAt PAKDD 2021, we presented a new method for ++[using GNNs to represent code snippets](https://www.amazon.science/publications/universal-representation-for-code)++. Recently, we have been using that method to identify similar code snippets, to find opportunities to make code more modular and easier to maintain.\n\nGNNs can also be used to encode global properties of the underlying systems and incorporate them into graph embeddings, in a way that is difficult with other deep-learning methods. We recently worked with scientists from Janssen Biopharmaceuticals to ++[predict the function of proteins](https://www.biorxiv.org/content/10.1101/2021.09.21.460852v1)++from their 3-D structure, which is useful for research and development in the pharmaceutical and biotech industries.\n\n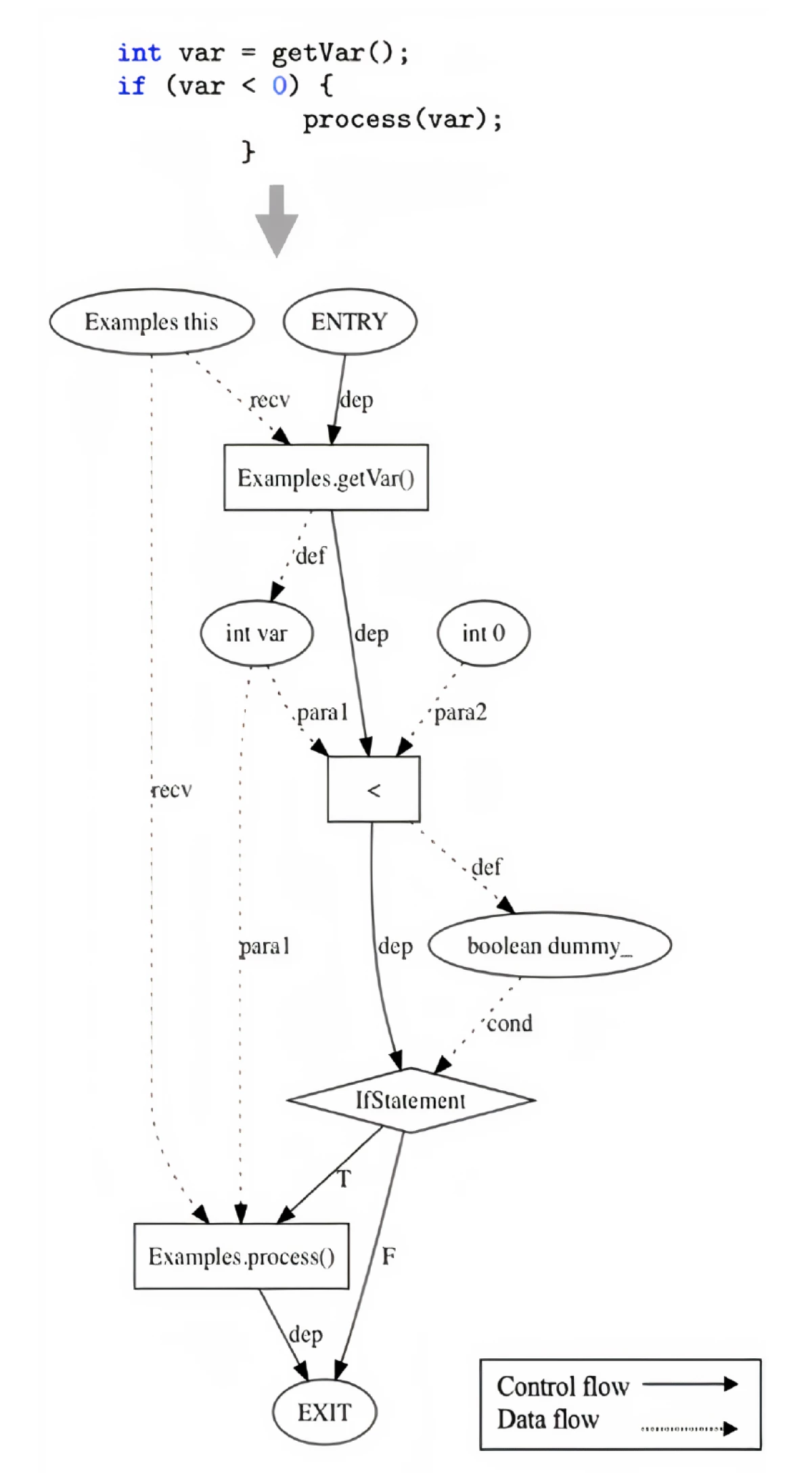\n\nAn example of a program dependence graph.\n\nA protein is composed of a sequence of amino acids folded in a particular way. We developed a graph representation of proteins in which each node was an amino acid, and the interactions between amino acids in the folded protein structure determined whether two nodes were linked or not.\n\n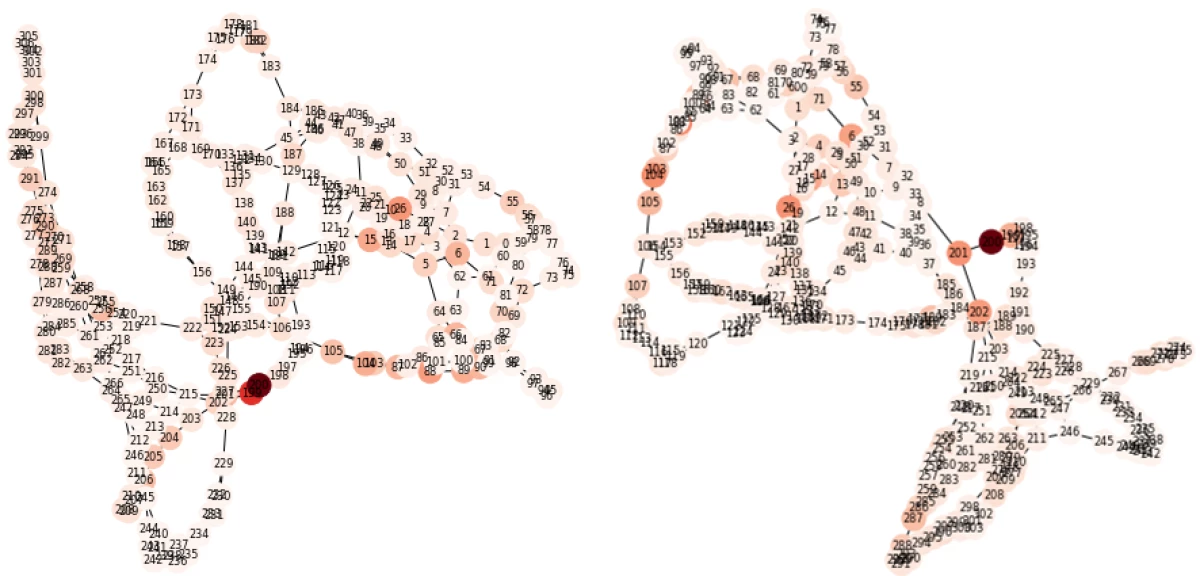\n\nExamples of graph representations of proteins.\n\nThis allowed us to encode fine-grained biological information, including the distance, angle, and direction of contact between neighboring amino acid residues. When we combined a GNN trained on these graph representations with a model trained to parse billions of protein sequences, we improved performance on various protein function prediction tasks of real-world importance.\n\nGraph-level tasks for GNNs have different data-engineering requirements than the previous tasks. Node-level and link-level tasks usually operate on a single giant graph, whereas graph-level tasks operate on a large number of independent small graphs.\n\nTo help customers scale GNNs up for graph-level tasks, we developed ++[a cloud-based architecture](https://aws.amazon.com/blogs/machine-learning/train-graph-neural-nets-for-millions-of-proteins-on-amazon-sagemaker-and-amazon-documentdb-with-mongodb-compatibility/)++ that leverages the highly performant open-source GNN library ++[DGL](https://www.dgl.ai/)++, the ML resource orchestration tool SageMaker, and ++[Amazon DocumentDB](https://aws.amazon.com/documentdb/)++ for managing graph data.\n\n#### **Getting started on your GNN journey**\n\n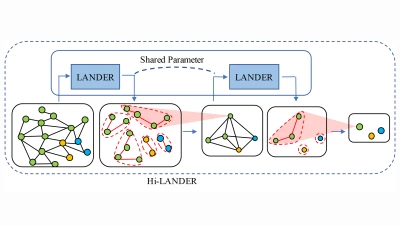\n\n**Related content**\n[Using supervised learning to train models for image clustering](https://www.amazon.science/blog/using-supervised-learning-to-train-models-for-image-clustering)\n\nIn this article, we presented a few examples of GNN applications at all three levels of graph-related tasks to showcase the value of GNNs to various enterprise and research problems. Amazon Web Services provides several options for customers looking to build and deploy GNN-powered ML solutions. Customers looking to get started quickly can use [Amazon Neptune](https://aws.amazon.com/cn/neptune/?trk=cndc-detail) ML to build GNN models directly on graph data stored in [Amazon Neptune](https://aws.amazon.com/cn/neptune/?trk=cndc-detail) without writing any code. [Amazon Neptune](https://aws.amazon.com/cn/neptune/?trk=cndc-detail) ML can train models to tackle node-level and link-level tasks like those described above. Customers looking to get more hands-on can implement GNN models using ++[DGL](https://www.dgl.ai/)++ on ++[Amazon SageMaker](https://docs.aws.amazon.com/sagemaker/latest/dg/deep-graph-library.html)++. In the meantime, we will continue to advance the science of GNNs to build more products and solutions to make GNNs more accessible to all our customers.\n\n**Acknowledgments**: ++[Guang Yang](https://www.amazon.science/author/guang-yang)++, Soji Adeshina, Jasleen Grewal, ++[Miguel Romero Calvo](https://www.amazon.science/author/miguel-calvo)++, ++[Suchitra Sathyanarayana](https://www.amazon.science/author/suchitra-sathyanarayana)++\n\nABOUT THE AUTHOR\n\n#### **[Zichen Wang](https://www.amazon.science/author/zichen-wang)**\nZichen Wang is an applied scientist with the Amazon Machine Learning Solutions Lab.\n\n#### **[Vassilis N. Ioannidis](https://www.amazon.science/author/vassilis-n-ioannidis)**\nVassilis N. Ioannidis is an applied scientist with Amazon Web Services.","render":"<p>Graphs are an information-rich way to represent data. A graph consists of nodes — typically represented by circles — and edges — typically represented as line segments between nodes. In a knowledge graph, for instance, the nodes represent entities, and the edges represent relationships between them. In a social graph, the nodes represent people, and an edge indicates that two of those people know each other.</p>\n<p>At Amazon Web Services, the use of machine learning (ML) to make the information encoded in graphs more useful to our customers has been a major research focus. In this post, we’ll showcase a variety of graph ML applications that customers have developed in collaboration with Amazon Web Services scientists, from malicious-account detection and automated document processing to knowledge-graph-assisted drug discovery and protein property prediction.</p>\n<h4><a id=\\"Introduction_to_graph_learning_4\\"></a><strong>Introduction to graph learning</strong></h4>\\n<p>Graphs can be homogenous, meaning the nodes represent a single type of entity (say, airports), and the edges represent a single type of relationship (say, scheduled flights). Or they can be heterogeneous, meaning they integrate multiple types of relationships among different entities, such as a graph of customers and products connected by both purchase histories and interests, or a knowledge graph of drugs, diseases, genes, and biological pathways connected by relationships such as indication and regulation. Nodes are often associated with data features, such as a product’s price or text description.</p>\n<p><img src=\\"https://dev-media.amazoncloud.cn/4753c52cc8a4432688c90515f1c616bb_image.png\\" alt=\\"image.png\\" /></p>\n<p>In a heterogenous knowledge graph, nodes can represent different classes of objects.</p>\n<h4><a id=\\"Graph_neural_networks_12\\"></a><strong>Graph neural networks</strong></h4>\\n<p>In the past 10 years, deep learning has revolutionized a host of AI applications, from natural-language processing to speech synthesis to computer vision.</p>\n<p>Graph neural networks (GNNs) extend the performance benefits of deep learning to graph data. Like other popular neural networks, a GNN model has a series of layers, which progress toward higher levels of abstraction.</p>\n<p>For instance, the first layer of a GNN computes a representation — or embedding — of the data represented by each node in the graph, while the second layer computes a representation of each node based on the prior embedding and the embeddings of the node’s nearest neighbors. In this way, every layer expands the scope of a node’s embedding, from one-hop neighbors, to two-hop neighbors, and for some applications, even further.</p>\n<p><img src=\\"https://dev-media.amazoncloud.cn/9fc090498b8845949bdd2bc7df37b3d4_%E4%B8%8B%E8%BD%BD.gif\\" alt=\\"下载.gif\\" /></p>\n<p>A demonstration of how graph neural networks use recursive embedding to condense all the information in a two-hop graph into a single vector. Relationships between entities — such as “produce” and “write” in a movie database (red and yellow arrows, respectively) — are encoded in the level-0 embeddings of the entities themselves (red and orange blocks).</p>\n<p>STACY REILLY</p>\n<h4><a id=\\"GNN_tasks_27\\"></a><strong>GNN tasks</strong></h4>\\n<p>The individual node embeddings can then be used for node-level tasks, such as predicting properties of a node. The embeddings can also be used for higher-level inferences. For instance, using representations across a pair of nodes or across all nodes from the graph, GNNs can perform link-level or graph-level tasks, respectively.</p>\n<p>In this section, we demonstrate the versatility of GNNs across all three levels of tasks and examine how our customers are using GNNs to tackle a variety of problems.</p>\n<h4><a id=\\"Nodelevel_tasks_33\\"></a><strong>Node-level tasks</strong></h4>\\n<p>Using GNNs, we can infer the behavior of an individual node in the graph based on the relationships it has to other nodes. One common task is node classification, where the objective is to infer nodes’ missing labels by looking at their neighbors’ labels and features. This method is used in applications such as financial-fraud detection, publication categorization, and disease classification.</p>\n<p>In Amazon Web Services, we have successfully used <ins><a href=\\"https://aws.amazon.com/neptune/machine-learning/\\" target=\\"_blank\\">Amazon Neptune</a></ins> and <ins><a href=\\"https://www.dgl.ai/\\" target=\\"_blank\\">Deep Graph Library (DGL)</a></ins> to apply GNN node representation learning to customers’ fraud detection use cases. For a large e-commerce sports gadgets customer, for instance, scientists in the <ins><a href=\\"https://aws.amazon.com/ml-solutions-lab/\\" target=\\"_blank\\">Amazon Machine Learning Solutions Lab</a></ins> successfully used GNN models implemented in DGL to detect malicious accounts among billions of registered accounts.</p>\n<p>These malicious accounts were created in large quantities to abuse usage of promotional codes and block general public access to the vendor’s best-selling items. Using data from e-commerce sites, we built a massive heterogenous graph in which the nodes represented accounts and other entities, such as products purchased, and the edges connected nodes based on usage histories. To identify malicious accounts, we trained a GNN model to propagate labels from accounts that were known to be malicious to unlabeled accounts.</p>\n<p><img src=\\"https://dev-media.amazoncloud.cn/fe0fe09760944a07b3b2a9c19b6034f0_image.png\\" alt=\\"image.png\\" /></p>\n<p>An example of how a graph representation can be used to detect fraud.</p>\n<p>With this method, we were able to detect 10 times as many malicious accounts as a previous rule-based detection method could. Such performance improvements could not be achieved by traditional methods for doing machine learning on tabular datasets, such as CatBoost, which take only account features as inputs, without considering the relationships between accounts captured by the graph.</p>\n<p>Besides applications for inherently relational, graph-structured data, such as social-network and citation-network data, there have been extensions of GNNs for data normally presented in Euclidean space, such as images and texts. By transforming data in Euclidean space to graphs based on spatial proximity, GNNs can solve problems that are typically solved by convolutional neural networks (CNNs) and recurrent neural networks (RNNs), which were designed to handle visual data and sequential data.</p>\n<p>For example, researchers have explored GNN models to improve the accuracy of information extraction, a task typically handled by RNNs. GNNs turn out to be better at incorporating the nonlocal and nonsequential relationships captured by graph representations of word dependencies.</p>\n<p>In a recent <ins><a href=\\"https://aws.amazon.com/solutions/case-studies/united-airlines-2021-reinvent-video/\\" target=\\"_blank\\">collaboration</a></ins>, the Amazon Machine Learning Solutions Lab and United Airlines developed a customized GNN model (DocGCN) to improve the accuracy of automatic information extraction from self-uploaded passenger documents, including travel documents, COVID-19 test results, and vaccine cards. The team built a graph for each scanned travel document that connected textual units based on their spatial proximities and orientations in the document.</p>\n<p>Then, the DocGCN model reasoned over the relationships among textual units (nodes of the graph) to improve the identification of relevant textual information. DocGCN also generalized to complex forms with different formats by leveraging graphs to capture relationships between texts in tables, key-value pairs, and paragraphs. This improvement expedited the automation of international travel readiness verification.</p>\n<h4><a id=\\"Linklevel_tasks_55\\"></a><strong>Link-level tasks</strong></h4>\\n<p>Another important learning task in graphs is link prediction, which is central to applications such as product or ad recommendation and friendship suggestion. Given two nodes and a relation, the goal is to determine whether the nodes are connected by the relation.</p>\n<p>Typically, the prediction is provided by a decoder that consumes the embeddings of the source and destination nodes, as in the work on <ins><a href=\\"https://www.amazon.science/blog/amazons-open-source-tools-make-embedding-knowledge-graphs-much-more-efficient\\" target=\\"_blank\\">knowledge graph embedding at scale</a></ins> that members of our team presented at SIGIR 2020. The decoder is trained to correctly predict existing edges in the graph.</p>\n<p><img src=\\"https://dev-media.amazoncloud.cn/ac798a5f28ba45dcb0f35811a2f29002_image.png\\" alt=\\"image.png\\" /></p>\n<p>The high-level structure of DRKG. Numerals indicate the number of different types of relationships between classes of entities; terms between parentheses are examples of those relationships.<br />\\nCREDIT: GLYNIS CONDON</p>\n<p>An exciting opportunity area in this context is drug discovery. Amazon Web Services has recently provided a drug-repurposing knowledge graph (<ins><a href=\\"https://www.amazon.science/blog/amazon-web-services-open-sources-biological-knowledge-graph-to-fight-covid-19\\" target=\\"_blank\\">DRKG</a></ins>) that employs link prediction to identify new targets for existing drugs. Built by scientists at Amazon Web Services, DRKG is a comprehensive biological knowledge graph that relates human genes, chemical compounds, biological processes, drug side effects, diseases, and symptoms. By performing link prediction around COVID-19 in DRKG, researchers were able to identify 41 drugs that were potentially effective against COVID-19 — 11 of which were already in clinical trials.</p>\n<p>Amazon Web Services also publicly released this solution, built by leveraging DRKG, as the COVID-19 Knowledge Graph (CKG). CKG organizes and represents the information in the COVID-19 Open Research Dataset (<ins><a href=\\"https://www.semanticscholar.org/cord19\\" target=\\"_blank\\">CORD-19</a></ins>), enabling fast discovery and prioritization of drug candidates. It can also be employed to identify <ins><a href=\\"https://www.amazon.science/blog/using-knowledge-graphs-to-streamline-covid-19-research\\" target=\\"_blank\\">papers relevant to COVID-19</a></ins>, thereby reducing the scale of human effort required to study, summarize, and interpret findings relevant to the pandemic.</p>\n<h4><a id=\\"Graphlevel_tasks_70\\"></a><strong>Graph-level tasks</strong></h4>\\n<p>Graph-level tasks involve the analysis of large collections of small and independent graphs. A chemical library of organic compounds is a common example of a graph-level application, where each organic compound is represented as a graph of atoms connected by chemical bonds. Graph-level analyses of chemical libraries are often vital for drug development and discovery use cases; applications include predicting organic compounds’ chemical properties and predicting biological activities such as binding affinity to protein targets.</p>\n<p>Another example of data that can benefit from graph-level representation is code snippets in programming languages. A piece of code can be represented by a program dependence graph (PDG), where variables, operators, and statements are nodes connected by their dependencies (links).</p>\n<p>At PAKDD 2021, we presented a new method for <ins><a href=\\"https://www.amazon.science/publications/universal-representation-for-code\\" target=\\"_blank\\">using GNNs to represent code snippets</a></ins>. Recently, we have been using that method to identify similar code snippets, to find opportunities to make code more modular and easier to maintain.</p>\n<p>GNNs can also be used to encode global properties of the underlying systems and incorporate them into graph embeddings, in a way that is difficult with other deep-learning methods. We recently worked with scientists from Janssen Biopharmaceuticals to ++<a href=\\"https://www.biorxiv.org/content/10.1101/2021.09.21.460852v1\\" target=\\"_blank\\">predict the function of proteins</a>++from their 3-D structure, which is useful for research and development in the pharmaceutical and biotech industries.</p>\\n<p><img src=\\"https://dev-media.amazoncloud.cn/a00c608ad0e343c6aae72a1851894ca7_image.png\\" alt=\\"image.png\\" /></p>\n<p>An example of a program dependence graph.</p>\n<p>A protein is composed of a sequence of amino acids folded in a particular way. We developed a graph representation of proteins in which each node was an amino acid, and the interactions between amino acids in the folded protein structure determined whether two nodes were linked or not.</p>\n<p><img src=\\"https://dev-media.amazoncloud.cn/4c9d4b673f42476489179b8da3b11d3a_image.png\\" alt=\\"image.png\\" /></p>\n<p>Examples of graph representations of proteins.</p>\n<p>This allowed us to encode fine-grained biological information, including the distance, angle, and direction of contact between neighboring amino acid residues. When we combined a GNN trained on these graph representations with a model trained to parse billions of protein sequences, we improved performance on various protein function prediction tasks of real-world importance.</p>\n<p>Graph-level tasks for GNNs have different data-engineering requirements than the previous tasks. Node-level and link-level tasks usually operate on a single giant graph, whereas graph-level tasks operate on a large number of independent small graphs.</p>\n<p>To help customers scale GNNs up for graph-level tasks, we developed <ins><a href=\\"https://aws.amazon.com/blogs/machine-learning/train-graph-neural-nets-for-millions-of-proteins-on-amazon-sagemaker-and-amazon-documentdb-with-mongodb-compatibility/\\" target=\\"_blank\\">a cloud-based architecture</a></ins> that leverages the highly performant open-source GNN library <ins><a href=\\"https://www.dgl.ai/\\" target=\\"_blank\\">DGL</a></ins>, the ML resource orchestration tool SageMaker, and <ins><a href=\\"https://aws.amazon.com/documentdb/\\" target=\\"_blank\\">Amazon DocumentDB</a></ins> for managing graph data.</p>\n<h4><a id=\\"Getting_started_on_your_GNN_journey_96\\"></a><strong>Getting started on your GNN journey</strong></h4>\\n<p><img src=\\"https://dev-media.amazoncloud.cn/c0f69de3c8a1424ca8ab2fc4f8659554_image.png\\" alt=\\"image.png\\" /></p>\n<p><strong>Related content</strong><br />\\n<a href=\\"https://www.amazon.science/blog/using-supervised-learning-to-train-models-for-image-clustering\\" target=\\"_blank\\">Using supervised learning to train models for image clustering</a></p>\\n<p>In this article, we presented a few examples of GNN applications at all three levels of graph-related tasks to showcase the value of GNNs to various enterprise and research problems. Amazon Web Services provides several options for customers looking to build and deploy GNN-powered ML solutions. Customers looking to get started quickly can use Amazon Neptune ML to build GNN models directly on graph data stored in Amazon Neptune without writing any code. Amazon Neptune ML can train models to tackle node-level and link-level tasks like those described above. Customers looking to get more hands-on can implement GNN models using <ins><a href=\\"https://www.dgl.ai/\\" target=\\"_blank\\">DGL</a></ins> on <ins><a href=\\"https://docs.aws.amazon.com/sagemaker/latest/dg/deep-graph-library.html\\" target=\\"_blank\\">Amazon SageMaker</a></ins>. In the meantime, we will continue to advance the science of GNNs to build more products and solutions to make GNNs more accessible to all our customers.</p>\n<p><strong>Acknowledgments</strong>: <ins><a href=\\"https://www.amazon.science/author/guang-yang\\" target=\\"_blank\\">Guang Yang</a></ins>, Soji Adeshina, Jasleen Grewal, <ins><a href=\\"https://www.amazon.science/author/miguel-calvo\\" target=\\"_blank\\">Miguel Romero Calvo</a></ins>, <ins><a href=\\"https://www.amazon.science/author/suchitra-sathyanarayana\\" target=\\"_blank\\">Suchitra Sathyanarayana</a></ins></p>\n<p>ABOUT THE AUTHOR</p>\n<h4><a id=\\"Zichen_Wanghttpswwwamazonscienceauthorzichenwang_109\\"></a><strong><a href=\\"https://www.amazon.science/author/zichen-wang\\" target=\\"_blank\\">Zichen Wang</a></strong></h4>\n<p>Zichen Wang is an applied scientist with the Amazon Machine Learning Solutions Lab.</p>\n<h4><a id=\\"Vassilis_N_Ioannidishttpswwwamazonscienceauthorvassilisnioannidis_112\\"></a><strong><a href=\\"https://www.amazon.science/author/vassilis-n-ioannidis\\" target=\\"_blank\\">Vassilis N. Ioannidis</a></strong></h4>\n<p>Vassilis N. Ioannidis is an applied scientist with Amazon Web Services.</p>\n"}