{"value":"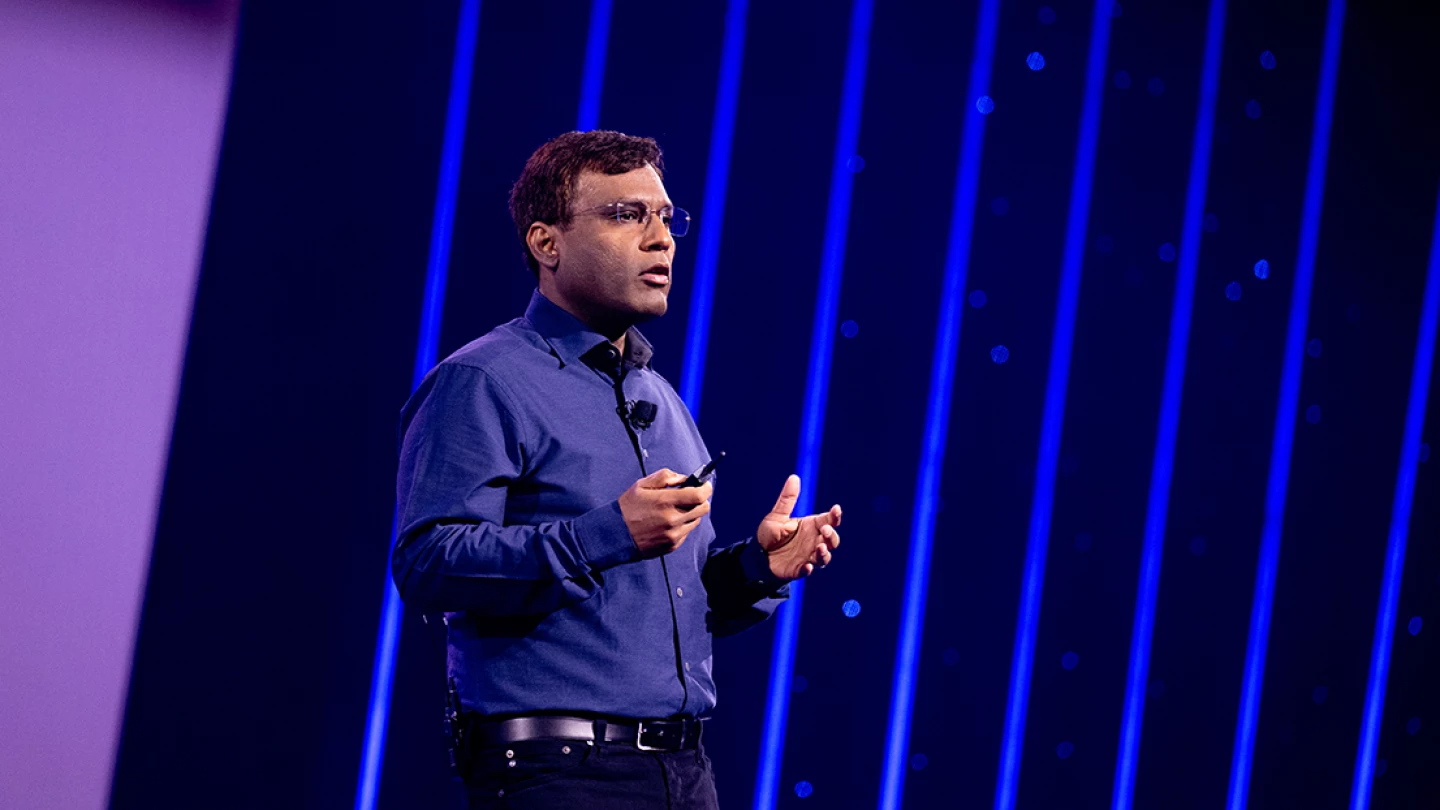\n\nAlexa AI senior vice president and head scientist Rohit Prasad onstage at re:MARS 2022.\n\nIn a talk today at re:MARS — Amazon’s conference on machine learning, automation, robotics, and space — ++[Rohit Prasad](https://www.amazon.science/author/rohit-prasad)++, Alexa AI senior vice president and head scientist, discussed the emerging paradigm of ambient intelligence, in which artificial intelligence is everywhere around you, responding to requests and anticipating your needs, but fading into the background when you don’t need it. Ambient intelligence, Prasad argued, offers the most practical route to generalizable intelligence, and the best evidence for that is the difference that Alexa is already making in customers’ lives.\n\nAmazon Science caught up with Prasad to ask him a few questions about his talk.\n\n#### **Q. What is ambient intelligence?**\n\n**A.** Ambient intelligence is artificial intelligence [AI] that is embedded everywhere in our environment. It is both reactive, responding to explicit customer requests, and proactive, anticipating customer needs. It uses a broad range of sensing technologies, like sound, vision, ultrasound, atmospheric sensing like temperature and humidity, depth sensors, and mechanical sensors, and it takes actions, playing your favorite tune, looking up information, buying products you need, or controlling thermostats, lights, or blinds in your smart home.\n\nAmbient intelligence is best exemplified by AI services like Alexa, which we use on a daily basis. Customers interact with Alexa billions of times each week. And thanks to predictive and proactive features like Hunches and Routines, more than 30% of smart-home interactions are initiated by Alexa.\n\n#### **Q. Why does ambient intelligence offer the most practical route to generalizable intelligence?**\n\n**A.** Alexa is made up of more than 30 machine learning systems that can each process different sensory signals. The real-time orchestration of these sophisticated machine learning systems makes Alexa one of the most complex applications of AI in the world.\n\n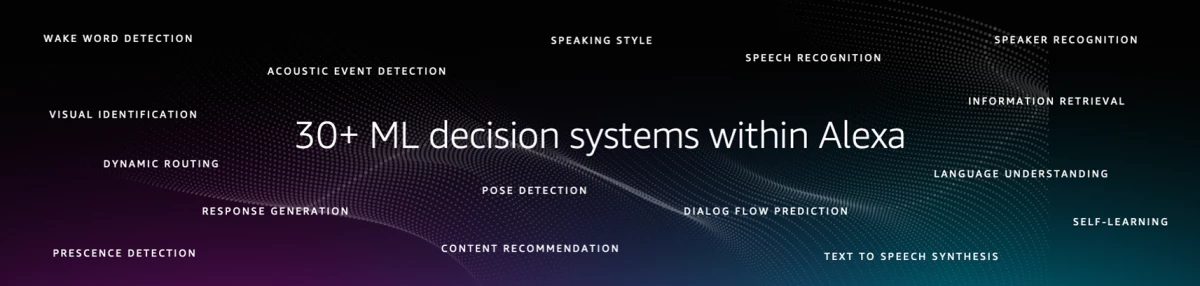\n\nAlexa is made up of more than 30 machine learning systems that process different sensory signals.\n\nStill, our customers demand even more from Alexa as their personal assistant, advisor, and companion. To continue to meet customer expectations, Alexa can’t just be a collection of special-purpose AI modules. Instead, it needs to be able to learn on its own and to generalize what it learns to new contexts. That’s why the ambient-intelligence path leads to generalizable intelligence.\n\nGeneralizable intelligence [GI] doesn’t imply an all-knowing, all-capable, über AI that can accomplish any task in the world. Our definition is more pragmatic, with three key attributes: a GI agent can (1) accomplish multiple tasks; (2) rapidly evolve to ever-changing environments; and (3) learn new concepts and actions with minimal external human input. For inspiration for such intelligence, we don’t need to look far: we humans are still the best example of generalization and the standard for AI to aspire to.\n\nWe’re already seeing some of this today, with AI generalizing much better than ever before. Foundational Transformer-based large language models trained with self-supervision are powering many tasks with significantly less manually labeled data than was required before. For example, our large language model pretrained on Alexa interactions — the Alexa Teacher Model — captures knowledge that is used in language understanding, dialogue prediction, speech recognition, and even visual-scene understanding. We have also proven that models trained on multiple languages often outperform single-language models.\n\nAnother element of better generalization is learning with little or no human involvement. Alexa’s self-learning mechanism is automatically correcting tens of millions of defects — both customer errors and errors in Alexa’s language-understanding models — each week. Customers can teach Alexa new behaviors, and Alexa can automatically generalize them across contexts — learning, for instance, that terms used to describe lighting settings can also be applied to speaker settings.\n\n#### **Q. Generalizing across contexts and reliably predicting customer needs will require more common sense than most AI systems exhibit today. How does common sense fit in to this picture?**\n\n**A.** To begin with, Alexa already exhibits common sense in a number of areas. For example, if you say to Alexa, “Set a reminder for the Super Bowl”, Alexa not only identifies the Super Bowl date and time but converts it into the customer’s time zone and reminds the customer 10 minutes before the start of the game, so they can wrap up what they are doing and get ready to watch the game.\n\nAnother example is suggested Routines, where Alexa detects frequent customer interaction patterns and proactively suggests automating them via a Routine. So if someone frequently asks Alexa to turn on the lights and turn up the heat at 7:00 a.m., Alexa might suggest a Routine that does that automatically.\n\nEven if the customer didn’t set up a Routine, Alexa can detect anomalies as part of its Hunches feature. For example, Alexa can alert you about the garage door being left open at 9:00 p.m., if it's usually closed at that time.\n\nMoving forward, we are aspiring to take automated reasoning to a whole new level. Our first goal is the pervasive use of commonsense knowledge in conversational AI. As part of that effort, we have collected and publicly released the largest dataset for ++[social common sense in an interactive setting](https://www.amazon.science/blog/amazon-releases-new-dataset-for-commonsense-dialogue)++.\n\nWe have also invented a generative approach that we call ++[think-before-you-speak](https://www.amazon.science/publications/think-before-you-speak-explicitly-generating-implicit-commonsenseknowledge-for-response-generation)++. In this approach, the AI learns to first externalize implicit commonsense knowledge — that is, “think” — using a large language model combined with a commonsense knowledge graph such as ConceptNet. Then it uses this knowledge to generate responses — that is, to “speak”.\n\n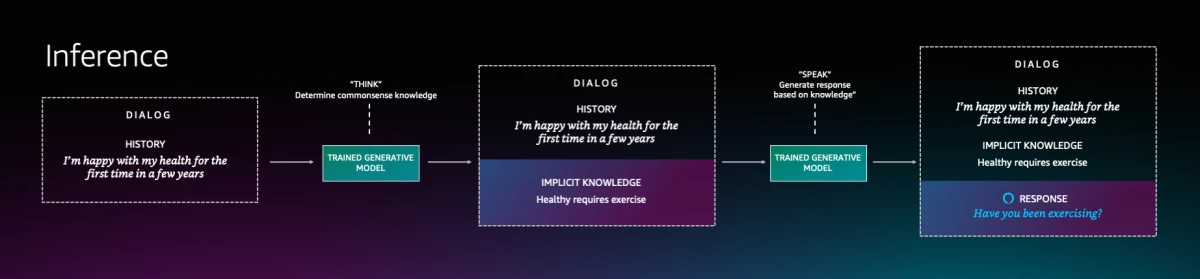\n\nAn overview of the think-before-you-speak approach.\n\nFor example, if during a social conversation on Valentine’s day a customer says, “Alexa, I want to buy flowers for my wife”, Alexa can leverage world knowledge and temporal context to respond with “Perhaps you should get her red roses”.\n\nWe’re also working to enable Alexa to answer complex queries that require multiple inference steps. For example, if a customer asks, \"Has Austria won more skiing medals than Norway?\", Alexa needs to combine the mention of skiing medals with temporal context to infer that the customer is asking about the Winter Olympics. Then Alexa needs to resolve “skiing” to the set of Winter Olympics events that involve skiing, which is not trivial, since those events can have names like “Nordic combined” and “biathlon”. Next, Alexa needs to retrieve and aggregate medal counts for each country and, finally, compare results.\n\n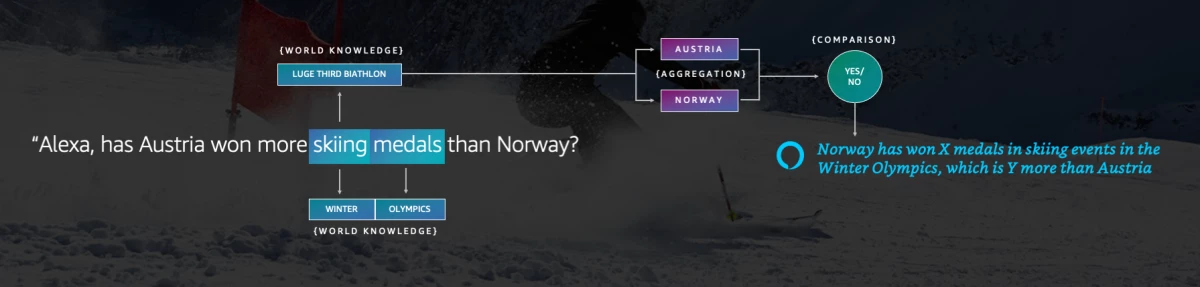\n\nThe Alexa AI team is working to enable Alexa to answer complex queries that require multiple inference steps.\n\nA key requirement for responding to such questions is explainability. Alexa shouldn't just reply \"yes\" but provide a response that summarizes Alexa's inference steps, such as \"Norway has won X medals in skiing events in the Winter Olympics, which is Y more than Austria\".\n\n#### **Q. What’s the one thing you are most excited about from your re:MARS keynote?**\n\n**A.** If I had to pick one thing among the suite of capabilities we showed at re:MARS, I’d say it is conversational explorations. Through the years, we have made Alexa far more knowledgeable, and it has gained expertise in many domains of information to answer natural-language queries from customers.\n\nNow, we are taking such question answering to the next level. We are enabling conversational explorations on ambient devices, so you don’t have to pull out your phone or go to your laptop to explore information on the web. Instead, Alexa guides you on your topic of interest, distilling a wide variety of information available on the web and shifting the heavy lifting of researching content from you to Alexa.\n\nThe idea is that when you ask Alexa a question — about a news story you’re following, a product you’re interested in, or, say, where to hike — the response includes specific information to help you make a decision, such as an excerpt from a product review. If that initial response gives you enough information to make a decision, great. But if it doesn’t — if, for instance, you ask for other options — that’s information that Alexa can use to sharpen its answer to your question or provide helpful suggestions.\n\nMaking this possible required three different types of advances. One is in dialogue flow prediction through deep learning in Alexa Conversations. The second is web-scale neural information retrieval to match relevant information to customer queries. And the third is automated summarization, to distill information from one or multiple sources.\n\n++[Alexa Conversations](https://www.amazon.science/blog/science-innovations-power-alexa-conversations-dialogue-management)++ is a dialogue manager that decides what actions Alexa should take based on customer interactions, dialogue history, and the current query or input. It lets users navigate and select information on-screen in a natural way — say, searching by topics or partial titles. And it uses query-guided attention and self-attention mechanisms to incorporate on-screen context into dialogue management, to understand how users are referencing entities on-screen.\n\nWeb-scale neural information retrieval retrieves information in different modalities and in different languages, at the scale of billions of data points. Conversational explorations uses Transformer-based models to semantically match customer queries with relevant information. The models are trained using a multistage training paradigm optimized for diverse data sources.\n\nAnd finally, conversational explorations uses deep-learning models to summarize information in bite-sized snippets, while keeping crucial information.\n\nCustomers will soon be able to experience such explorations, and we’re excited to get their feedback, to help us expand and enhance this capability in the months ahead.\n\nABOUT THE AUTHOR\n\n**Staff writer**","render":"<p><img src=\\"https://dev-media.amazoncloud.cn/8bc3a2961fec4495a0b8718f779d371a_%E4%B8%8B%E8%BD%BD.jpg\\" alt=\\"下载.jpg\\" /></p>\n<p>Alexa AI senior vice president and head scientist Rohit Prasad onstage at re:MARS 2022.</p>\n<p>In a talk today at re:MARS — Amazon’s conference on machine learning, automation, robotics, and space — <ins><a href=\\"https://www.amazon.science/author/rohit-prasad\\" target=\\"_blank\\">Rohit Prasad</a></ins>, Alexa AI senior vice president and head scientist, discussed the emerging paradigm of ambient intelligence, in which artificial intelligence is everywhere around you, responding to requests and anticipating your needs, but fading into the background when you don’t need it. Ambient intelligence, Prasad argued, offers the most practical route to generalizable intelligence, and the best evidence for that is the difference that Alexa is already making in customers’ lives.</p>\n<p>Amazon Science caught up with Prasad to ask him a few questions about his talk.</p>\n<h4><a id=\\"Q_What_is_ambient_intelligence_8\\"></a><strong>Q. What is ambient intelligence?</strong></h4>\\n<p><strong>A.</strong> Ambient intelligence is artificial intelligence [AI] that is embedded everywhere in our environment. It is both reactive, responding to explicit customer requests, and proactive, anticipating customer needs. It uses a broad range of sensing technologies, like sound, vision, ultrasound, atmospheric sensing like temperature and humidity, depth sensors, and mechanical sensors, and it takes actions, playing your favorite tune, looking up information, buying products you need, or controlling thermostats, lights, or blinds in your smart home.</p>\\n<p>Ambient intelligence is best exemplified by AI services like Alexa, which we use on a daily basis. Customers interact with Alexa billions of times each week. And thanks to predictive and proactive features like Hunches and Routines, more than 30% of smart-home interactions are initiated by Alexa.</p>\n<h4><a id=\\"Q_Why_does_ambient_intelligence_offer_the_most_practical_route_to_generalizable_intelligence_14\\"></a><strong>Q. Why does ambient intelligence offer the most practical route to generalizable intelligence?</strong></h4>\\n<p><strong>A.</strong> Alexa is made up of more than 30 machine learning systems that can each process different sensory signals. The real-time orchestration of these sophisticated machine learning systems makes Alexa one of the most complex applications of AI in the world.</p>\\n<p><img src=\\"https://dev-media.amazoncloud.cn/eedcfd61ae0742dea391355e71b6507b_%E4%B8%8B%E8%BD%BD%20%281%29.jpg\\" alt=\\"下载 1.jpg\\" /></p>\n<p>Alexa is made up of more than 30 machine learning systems that process different sensory signals.</p>\n<p>Still, our customers demand even more from Alexa as their personal assistant, advisor, and companion. To continue to meet customer expectations, Alexa can’t just be a collection of special-purpose AI modules. Instead, it needs to be able to learn on its own and to generalize what it learns to new contexts. That’s why the ambient-intelligence path leads to generalizable intelligence.</p>\n<p>Generalizable intelligence [GI] doesn’t imply an all-knowing, all-capable, über AI that can accomplish any task in the world. Our definition is more pragmatic, with three key attributes: a GI agent can (1) accomplish multiple tasks; (2) rapidly evolve to ever-changing environments; and (3) learn new concepts and actions with minimal external human input. For inspiration for such intelligence, we don’t need to look far: we humans are still the best example of generalization and the standard for AI to aspire to.</p>\n<p>We’re already seeing some of this today, with AI generalizing much better than ever before. Foundational Transformer-based large language models trained with self-supervision are powering many tasks with significantly less manually labeled data than was required before. For example, our large language model pretrained on Alexa interactions — the Alexa Teacher Model — captures knowledge that is used in language understanding, dialogue prediction, speech recognition, and even visual-scene understanding. We have also proven that models trained on multiple languages often outperform single-language models.</p>\n<p>Another element of better generalization is learning with little or no human involvement. Alexa’s self-learning mechanism is automatically correcting tens of millions of defects — both customer errors and errors in Alexa’s language-understanding models — each week. Customers can teach Alexa new behaviors, and Alexa can automatically generalize them across contexts — learning, for instance, that terms used to describe lighting settings can also be applied to speaker settings.</p>\n<h4><a id=\\"Q_Generalizing_across_contexts_and_reliably_predicting_customer_needs_will_require_more_common_sense_than_most_AI_systems_exhibit_today_How_does_common_sense_fit_in_to_this_picture_30\\"></a><strong>Q. Generalizing across contexts and reliably predicting customer needs will require more common sense than most AI systems exhibit today. How does common sense fit in to this picture?</strong></h4>\\n<p><strong>A.</strong> To begin with, Alexa already exhibits common sense in a number of areas. For example, if you say to Alexa, “Set a reminder for the Super Bowl”, Alexa not only identifies the Super Bowl date and time but converts it into the customer’s time zone and reminds the customer 10 minutes before the start of the game, so they can wrap up what they are doing and get ready to watch the game.</p>\\n<p>Another example is suggested Routines, where Alexa detects frequent customer interaction patterns and proactively suggests automating them via a Routine. So if someone frequently asks Alexa to turn on the lights and turn up the heat at 7:00 a.m., Alexa might suggest a Routine that does that automatically.</p>\n<p>Even if the customer didn’t set up a Routine, Alexa can detect anomalies as part of its Hunches feature. For example, Alexa can alert you about the garage door being left open at 9:00 p.m., if it’s usually closed at that time.</p>\n<p>Moving forward, we are aspiring to take automated reasoning to a whole new level. Our first goal is the pervasive use of commonsense knowledge in conversational AI. As part of that effort, we have collected and publicly released the largest dataset for <ins><a href=\\"https://www.amazon.science/blog/amazon-releases-new-dataset-for-commonsense-dialogue\\" target=\\"_blank\\">social common sense in an interactive setting</a></ins>.</p>\n<p>We have also invented a generative approach that we call <ins><a href=\\"https://www.amazon.science/publications/think-before-you-speak-explicitly-generating-implicit-commonsenseknowledge-for-response-generation\\" target=\\"_blank\\">think-before-you-speak</a></ins>. In this approach, the AI learns to first externalize implicit commonsense knowledge — that is, “think” — using a large language model combined with a commonsense knowledge graph such as ConceptNet. Then it uses this knowledge to generate responses — that is, to “speak”.</p>\n<p><img src=\\"https://dev-media.amazoncloud.cn/715a29f6a3984f31854197c48137e2ef_%E4%B8%8B%E8%BD%BD%20%282%29.jpg\\" alt=\\"下载 2.jpg\\" /></p>\n<p>An overview of the think-before-you-speak approach.</p>\n<p>For example, if during a social conversation on Valentine’s day a customer says, “Alexa, I want to buy flowers for my wife”, Alexa can leverage world knowledge and temporal context to respond with “Perhaps you should get her red roses”.</p>\n<p>We’re also working to enable Alexa to answer complex queries that require multiple inference steps. For example, if a customer asks, “Has Austria won more skiing medals than Norway?”, Alexa needs to combine the mention of skiing medals with temporal context to infer that the customer is asking about the Winter Olympics. Then Alexa needs to resolve “skiing” to the set of Winter Olympics events that involve skiing, which is not trivial, since those events can have names like “Nordic combined” and “biathlon”. Next, Alexa needs to retrieve and aggregate medal counts for each country and, finally, compare results.</p>\n<p><img src=\\"https://dev-media.amazoncloud.cn/13645c7fc6c9423aaacb1b397fb29507_%E4%B8%8B%E8%BD%BD%20%283%29.jpg\\" alt=\\"下载 3.jpg\\" /></p>\n<p>The Alexa AI team is working to enable Alexa to answer complex queries that require multiple inference steps.</p>\n<p>A key requirement for responding to such questions is explainability. Alexa shouldn’t just reply “yes” but provide a response that summarizes Alexa’s inference steps, such as “Norway has won X medals in skiing events in the Winter Olympics, which is Y more than Austria”.</p>\n<h4><a id=\\"Q_Whats_the_one_thing_you_are_most_excited_about_from_your_reMARS_keynote_56\\"></a><strong>Q. What’s the one thing you are most excited about from your re:MARS keynote?</strong></h4>\\n<p><strong>A.</strong> If I had to pick one thing among the suite of capabilities we showed at re:MARS, I’d say it is conversational explorations. Through the years, we have made Alexa far more knowledgeable, and it has gained expertise in many domains of information to answer natural-language queries from customers.</p>\\n<p>Now, we are taking such question answering to the next level. We are enabling conversational explorations on ambient devices, so you don’t have to pull out your phone or go to your laptop to explore information on the web. Instead, Alexa guides you on your topic of interest, distilling a wide variety of information available on the web and shifting the heavy lifting of researching content from you to Alexa.</p>\n<p>The idea is that when you ask Alexa a question — about a news story you’re following, a product you’re interested in, or, say, where to hike — the response includes specific information to help you make a decision, such as an excerpt from a product review. If that initial response gives you enough information to make a decision, great. But if it doesn’t — if, for instance, you ask for other options — that’s information that Alexa can use to sharpen its answer to your question or provide helpful suggestions.</p>\n<p>Making this possible required three different types of advances. One is in dialogue flow prediction through deep learning in Alexa Conversations. The second is web-scale neural information retrieval to match relevant information to customer queries. And the third is automated summarization, to distill information from one or multiple sources.</p>\n<p><ins><a href=\\"https://www.amazon.science/blog/science-innovations-power-alexa-conversations-dialogue-management\\" target=\\"_blank\\">Alexa Conversations</a></ins> is a dialogue manager that decides what actions Alexa should take based on customer interactions, dialogue history, and the current query or input. It lets users navigate and select information on-screen in a natural way — say, searching by topics or partial titles. And it uses query-guided attention and self-attention mechanisms to incorporate on-screen context into dialogue management, to understand how users are referencing entities on-screen.</p>\n<p>Web-scale neural information retrieval retrieves information in different modalities and in different languages, at the scale of billions of data points. Conversational explorations uses Transformer-based models to semantically match customer queries with relevant information. The models are trained using a multistage training paradigm optimized for diverse data sources.</p>\n<p>And finally, conversational explorations uses deep-learning models to summarize information in bite-sized snippets, while keeping crucial information.</p>\n<p>Customers will soon be able to experience such explorations, and we’re excited to get their feedback, to help us expand and enhance this capability in the months ahead.</p>\n<p>ABOUT THE AUTHOR</p>\n<p><strong>Staff writer</strong></p>\n"}