{"value":"The Causal Learning and Reasoning ([CLeaR](https://www.cclear.cc/2022)) conference — the first international conference on causal learning and reasoning — will launch next week in Eureka, CA, with Amazon as one of its three gold (top-tier) sponsors. [Bernhard Schölkopf](https://www.amazon.science/author/bernhard-scholkopf), an Amazon vice president and distinguished scientist and a l[eading researcher in the field of causal inference](https://scholar.google.com/citations?view_op=search_authors&hl=en&mauthors=label:causal_inference), is one of the conference organizers.\n\nIn the past few decades, some of the most influential developments in the study of causal discovery, causal inference, and the causal treatment of machine learning have resulted from cross-disciplinary efforts. In particular, a number of machine learning and[ statistical-analysis techniques](https://www.amazon.science/blog/determining-causality-in-correlated-time-series) have been developed to tackle classical causal-discovery and -inference problems. On the other hand, the causal view has proved useful for formulating, understanding, and tackling problems in [transfer learning](https://ieeexplore.ieee.org/abstract/document/9363924), reinforcement learning, and deep learning.\n\n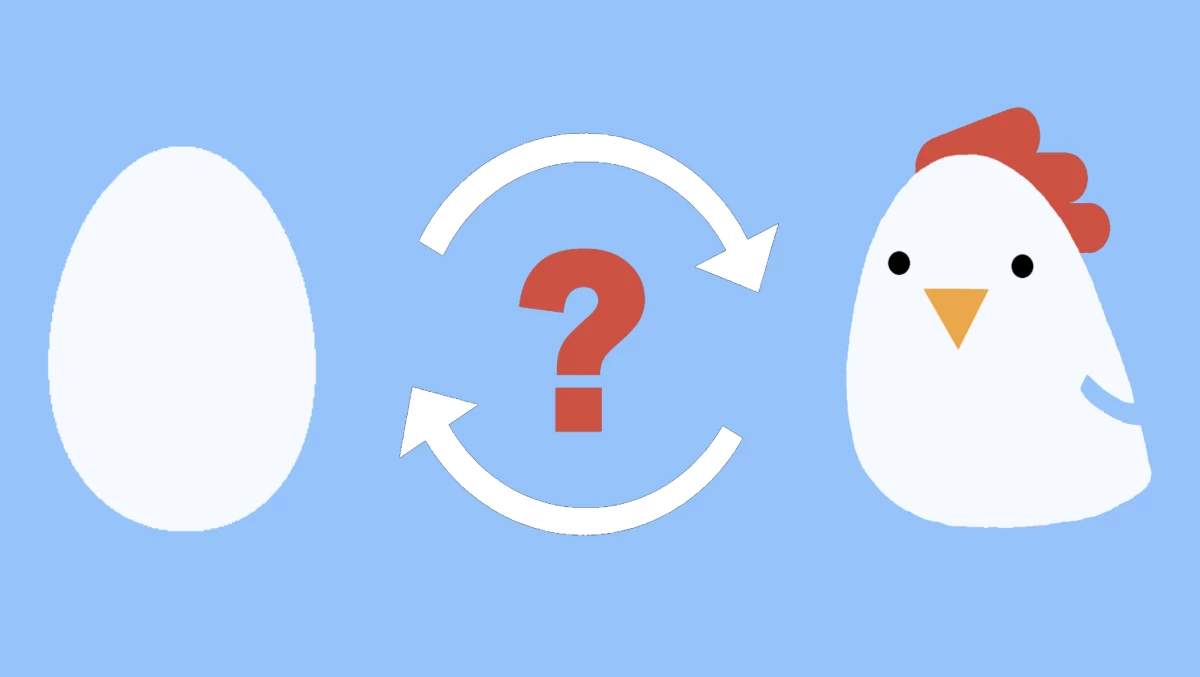\n\nThe CLeaR logo.\n\n“Machine learning ultimately is based on statistical dependencies,” Schölkopf[recently told Amazon Science](https://www.amazon.science/videos-webinars/neurips-luminaries-on-the-future-of-ai). “Causality is a concept that describes the dependencies in the system on a more fundamental level that produces statistical dependencies on the surface. Oftentimes, it's enough if we work at the surface and just learn from these dependencies. But it's only enough as long as we're in this setting where nothing changes. Once things start changing, it's helpful to think about the causality.”\n\nThe conference will feature [nine oral presentations and 40 poster presentations](https://www.cclear.cc/AcceptedPapers), covering topics that range from causal fairness and non-parametric inference to causal Markov decision processes and social-influence estimation.\n\nCLeaR will be held from April 11 to 13. [Registration is still open](https://www.cclear.cc/VenueandRegistration), with virtual-attendance options.\n\n\nABOUT THE AUTHOR\n\n#### Staff writer","render":"<p>The Causal Learning and Reasoning (<a href=\"https://www.cclear.cc/2022\" target=\"_blank\">CLeaR</a>) conference — the first international conference on causal learning and reasoning — will launch next week in Eureka, CA, with Amazon as one of its three gold (top-tier) sponsors. <a href=\"https://www.amazon.science/author/bernhard-scholkopf\" target=\"_blank\">Bernhard Schölkopf</a>, an Amazon vice president and distinguished scientist and a l<a href=\"https://scholar.google.com/citations?view_op=search_authors&hl=en&mauthors=label:causal_inference\" target=\"_blank\">eading researcher in the field of causal inference</a>, is one of the conference organizers.</p>\n<p>In the past few decades, some of the most influential developments in the study of causal discovery, causal inference, and the causal treatment of machine learning have resulted from cross-disciplinary efforts. In particular, a number of machine learning and<a href=\"https://www.amazon.science/blog/determining-causality-in-correlated-time-series\" target=\"_blank\"> statistical-analysis techniques</a> have been developed to tackle classical causal-discovery and -inference problems. On the other hand, the causal view has proved useful for formulating, understanding, and tackling problems in <a href=\"https://ieeexplore.ieee.org/abstract/document/9363924\" target=\"_blank\">transfer learning</a>, reinforcement learning, and deep learning.</p>\n<p><img src=\"https://dev-media.amazoncloud.cn/0629b2ebf54c4ca0b9dcb4572f538f7a_%E4%B8%8B%E8%BD%BD.jpg\" alt=\"下载.jpg\" /></p>\n<p>The CLeaR logo.</p>\n<p>“Machine learning ultimately is based on statistical dependencies,” Schölkopf<a href=\"https://www.amazon.science/videos-webinars/neurips-luminaries-on-the-future-of-ai\" target=\"_blank\">recently told Amazon Science</a>. “Causality is a concept that describes the dependencies in the system on a more fundamental level that produces statistical dependencies on the surface. Oftentimes, it’s enough if we work at the surface and just learn from these dependencies. But it’s only enough as long as we’re in this setting where nothing changes. Once things start changing, it’s helpful to think about the causality.”</p>\n<p>The conference will feature <a href=\"https://www.cclear.cc/AcceptedPapers\" target=\"_blank\">nine oral presentations and 40 poster presentations</a>, covering topics that range from causal fairness and non-parametric inference to causal Markov decision processes and social-influence estimation.</p>\n<p>CLeaR will be held from April 11 to 13. <a href=\"https://www.cclear.cc/VenueandRegistration\" target=\"_blank\">Registration is still open</a>, with virtual-attendance options.</p>\n<p>ABOUT THE AUTHOR</p>\n<h4><a id=\"Staff_writer_17\"></a>Staff writer</h4>\n"}