{"value":"Recent algorithmic advances and hardware innovations have made it possible to train deep neural networks with billions of parameters. The networks’ performance, however, depends in part on hyperparameters such as the learning rate and the number and width of network layers.\n\nTuning hyperparameters is difficult and time-consuming, even for experts, and criteria like latency or cost often play a role in deciding the winning hyperparameter configuration. To make latest deep-learning technology practical for nonexperts, it is essential to automate hyperparameter tuning.\n\n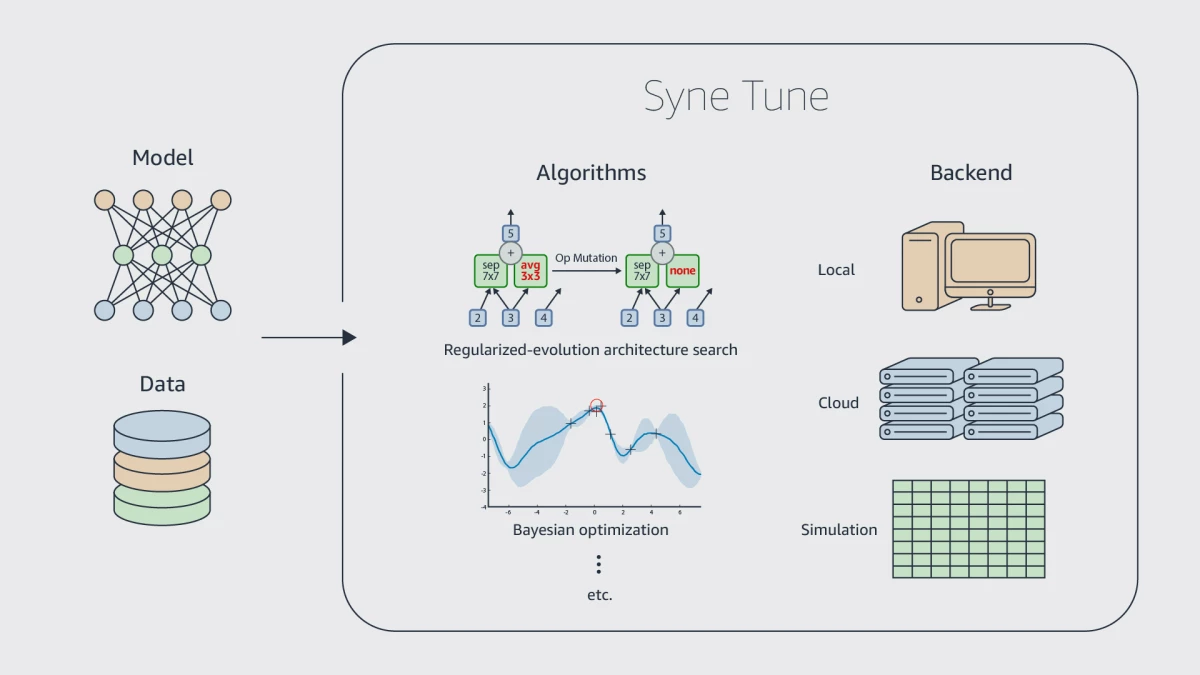\n\nSyne Tune provides implementations of a broad range of synchronous and asynchronous HPO algorithms and three execution backends with a general interface.\n\nTuning hyperparameters is difficult and time-consuming, even for experts, and criteria like latency or cost often play a role in deciding the winning hyperparameter configuration. To make latest deep-learning technology practical for nonexperts, it is essential to automate hyperparameter tuning.\n\nAt the first [International Conference on Automated Machine Learning](https://automl.cc/) (AutoML), we presented [Syne Tune](https://www.amazon.science/publications/syne-tune-a-library-for-large-scale-hyperparameter-tuning-and-reproducible-research), an [open-source library](https://github.com/awslabs/syne-tune) for large-scale hyperparameter optimization (HPO) with an emphasis on enabling reproducible machine learning research. It simplifies, standardizes, and accelerates the evaluation of a wide variety of HPO algorithms.\n\nThese algorithms are implemented on top of common modules and aim to remove implementation bias to enable fair comparisons. By supporting different execution backends, the library also enables researchers and engineers to effortlessly move from simulation and small-scale experimentation to large-scale distributed tuning on the cloud.\n\nIn this post, we will give an overview of the execution backends supported in Syne Tune and benchmark state-of-the-art asynchronous HPO algorithms, including transfer learning baselines.\n\n##### **Supported execution backends**\nSyne Tune provides a general interface for backends and three implementations: one to evaluate trials on a local machine, one to evaluate trials in the cloud, and one to simulate tuning with tabulated benchmarks to reduce run time. Switching between different backends is a matter of simply passing a different trial_backend parameter to the tuner, as shown in the code examples below. The backend API has been kept lean on purpose, and adding new backends requires little effort.\n\n##### **Local backend**\nThis backend evaluates trials concurrently on a single machine by using subprocesses. We support rotating multiple GPUs on the machine, assigning the next trial to the least busy GPU (e.g., the GPU with the fewest number of trials currently running). Trial checkpoints and logs are stored to local files.\n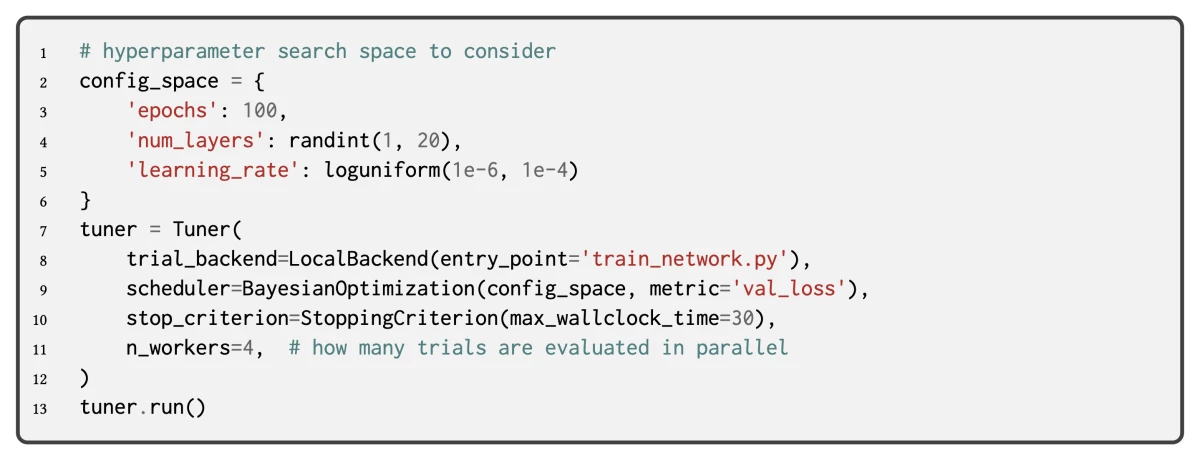\nHow to tune a training script with Bayesian optimization in Syne Tune.\n##### **Cloud backend**\nRunning on a single machine limits the number of trials that can run concurrently. Moreover, neural-network training may require many GPUs, even distributed across several nodes, or multi-GPU devices. For those use cases, we provide an [Amazon SageMaker](https://aws.amazon.com/cn/sagemaker/?trk=cndc-detail) backend that can run multiple trials in parallel.\n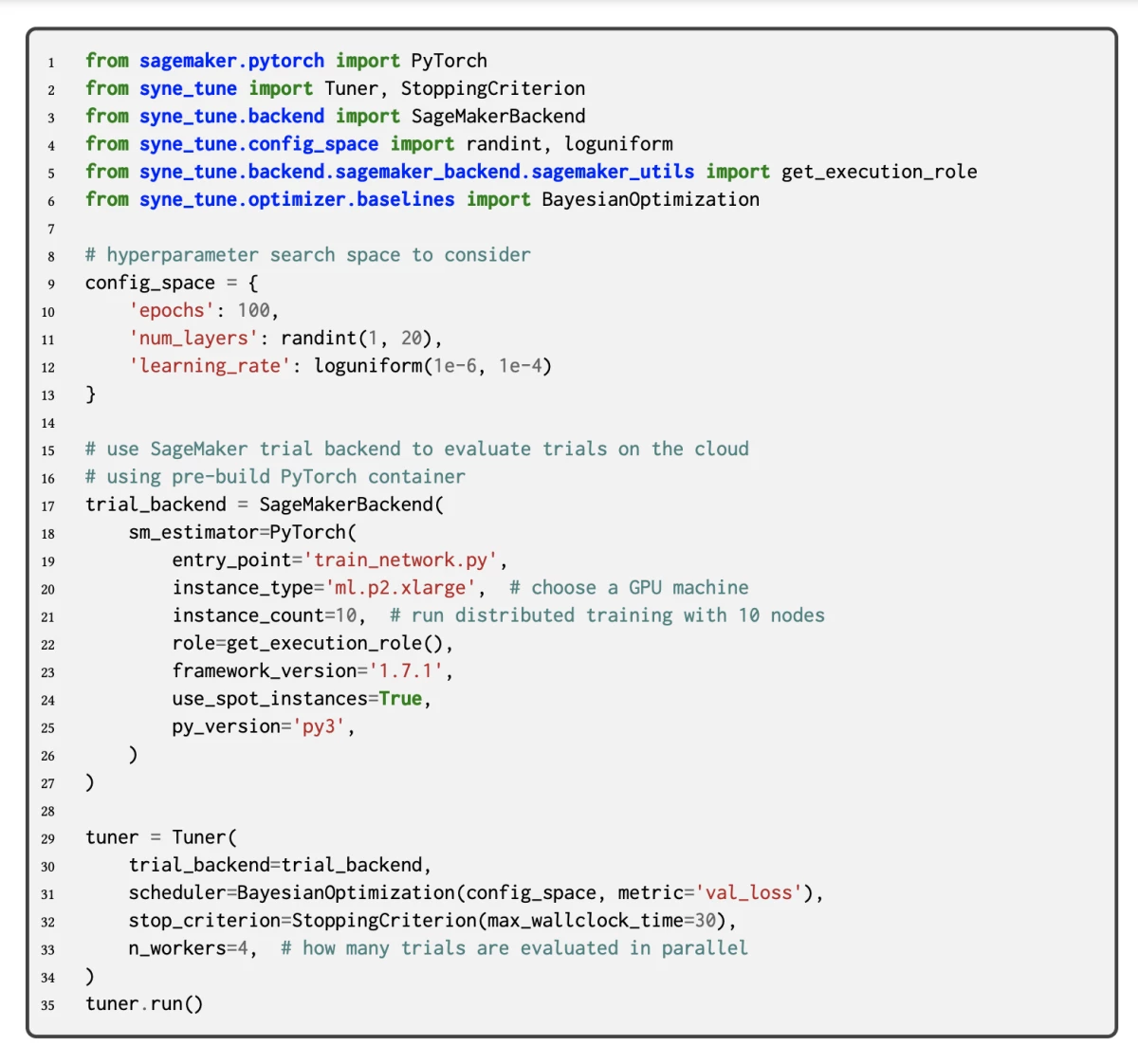\nChanging the trial backend to run on cloud machines.\n##### **Simulation backend**\nA growing number of tabulated benchmarks are available for HPO and neural-architecture-search (NAS) research. The simulation backend allows the execution of realistic experiments with such benchmarks on a single CPU instance, paying real time for the decision-making only.\n\nTo this end, we use a timekeeper to manage simulated time and a priority queue of time-stamped events (e.g., reporting-metric values for running trials), which work together to ensure that interactions between trials and the scheduler happen in the right ordering, whatever the experimental setup may be. The simulator correctly handles any number of workers, and delay due to model-based decision-making is taken into account.\n\n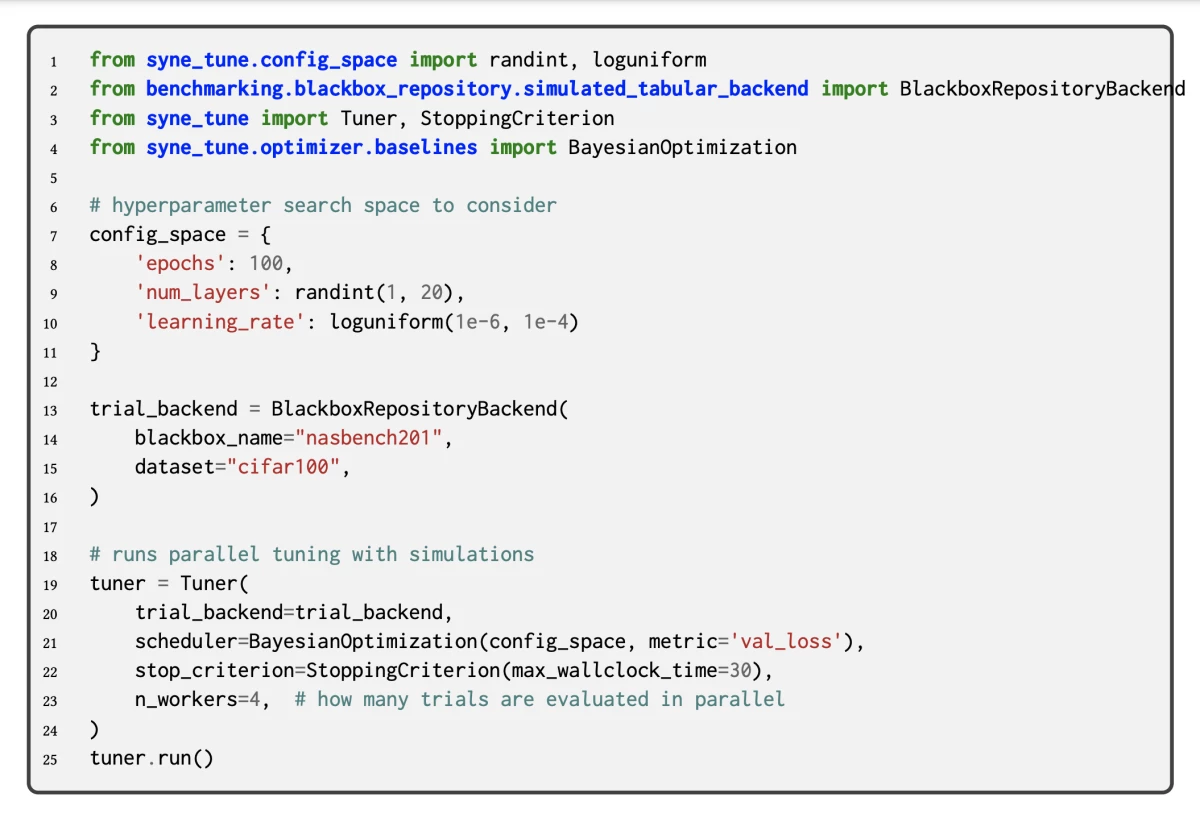\nChanging the trial backend to run on parallel and asynchronous simulations based on tabulated benchmarks.\n ##### **Comparing asynchronous tuning algorithms**\nSyne Tune provides implementations of a broad range of synchronous and asynchronous HPO algorithms. In our experiments, we consider single-fidelity HPO algorithms, which require entire training runs to evaluate a candidate hyperparameter configuration. Random search ([RS](https://www.jmlr.org/papers/v13/bergstra12a.html)), regularized evolution for architecture search ([REA](https://arxiv.org/abs/1802.01548)), and Bayesian-optimization variants (e.g., Gaussian-process-based ([GP](https://papers.nips.cc/paper/2012/hash/05311655a15b75fab86956663e1819cd-Abstract.html)) and density-ratio-based ([BORE](http://proceedings.mlr.press/v139/tiao21a.html)), of which [TPE](https://papers.nips.cc/paper/2011/hash/86e8f7ab32cfd12577bc2619bc635690-Abstract.html) is a special case) fall in this category.\n\nWe also consider multi-fidelity HPO algorithms, which stop unpromising training runs early. The median stopping rule ([MSR](https://static.googleusercontent.com/media/research.google.com/en/pubs/archive/46180.pdf)), asynchronous successive halving ([ASHA](https://arxiv.org/abs/1810.05934)), and asynchronous Bayesian-optimization variants (e.g., [BOHB](https://proceedings.mlr.press/v80/falkner18a.html) and [MOB](https://arxiv.org/abs/1905.04970)) are prominent examples.\n\nThe table below shows the normalized rank, averaged over wall-clock time, of these single- and multi-fidelity optimizers on three publicly available neural-architecture-search benchmarks: FCNet, from [Klein and Hutter (2019)](https://arxiv.org/abs/1905.04970); NAS201, from [Dong and Yang (2020)](https://arxiv.org/abs/2001.00326); and LCBench, from [Zimmer et al. (2021)](https://arxiv.org/abs/2006.13799).\n\nMulti-fidelity algorithms are in general superior to single-fidelity algorithms, which is expected, as they make more efficient use of the computational resources available to them. These results are also consistent with previous results reported in the literature. It should be noted that among the multi-fidelity algorithms, MSR is the only one not using successive halving, and it performs worst.\nThe table also shows the average normalized rank of transfer learning approaches. Hyperparameter transfer learning uses evaluation data from past HPO tasks in order to warmstart the current HPO task, which can result in significant speed-ups in practice.\n\nSyne Tune supports transfer-learning-based HPO via an abstraction that maps a scheduler and transfer learning data to a warmstarted instance of the former. We consider the bounding-box and quantile-based ASHA, respectively referred to as [ASHA-BB](https://dl.acm.org/doi/10.5555/3454287.3455431) and [ASHA-CTS](https://proceedings.mlr.press/v119/salinas20a.html). We also consider a zero-shot approach ([ZS](https://ieeexplore.ieee.org/document/7373431)), which greedily selects hyperparameter configurations that complement previously considered ones, based on historical performances; and [RUSH](https://arxiv.org/abs/2103.16111), which warmstarts ASHA with the best configurations found for previous tasks. As expected, we find that transfer learning approaches accelerate HPO.\n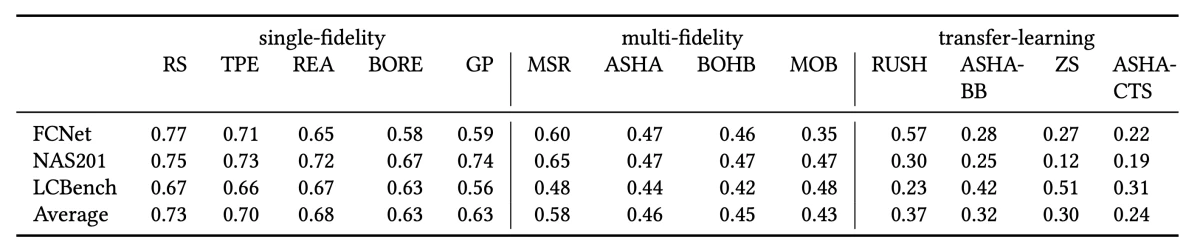\nAverage normalized rank (lower is better) of algorithms across time and benchmarks. Best results per category are indicated in bold.\nOur experiments show that [Syne Tune](https://github.com/awslabs/syne-tune) makes research on automated machine learning more efficient, reliable, and trustworthy. By making simulation on tabulated benchmarks a first-class citizen, it makes hyperparameter optimization accessible to researchers without massive computation budgets. By supporting advanced use cases, such as hyperparameter transfer learning, it allows better problem solving in practice.\n\nTo learn more about the library and contribute to it, please check out the [paper](https://www.amazon.science/publications/syne-tune-a-library-for-large-scale-hyperparameter-tuning-and-reproducible-research) and our GitHub repo for [documentation](https://github.com/awslabs/sagemaker-tune). We just released the 0.3 version, with new HPO algorithms, new benchmarks, tensorboard visualization, and more.\n\nABOUT THE AUTHOR\n#### **[Cedric Archambeau](https://www.amazon.science/author/cedric-archambeau)**\nCédric Archambeau is a principal applied scientist with Amazon Web Services.","render":"<p>Recent algorithmic advances and hardware innovations have made it possible to train deep neural networks with billions of parameters. The networks’ performance, however, depends in part on hyperparameters such as the learning rate and the number and width of network layers.</p>\n<p>Tuning hyperparameters is difficult and time-consuming, even for experts, and criteria like latency or cost often play a role in deciding the winning hyperparameter configuration. To make latest deep-learning technology practical for nonexperts, it is essential to automate hyperparameter tuning.</p>\n<p><img src=\\"https://awsdevweb.s3.cn-north-1.amazonaws.com.cn/e78b8ddbcbbd4f3abcf80de6ae52eae0_image.png\\" alt=\\"image.png\\" /></p>\n<p>Syne Tune provides implementations of a broad range of synchronous and asynchronous HPO algorithms and three execution backends with a general interface.</p>\n<p>Tuning hyperparameters is difficult and time-consuming, even for experts, and criteria like latency or cost often play a role in deciding the winning hyperparameter configuration. To make latest deep-learning technology practical for nonexperts, it is essential to automate hyperparameter tuning.</p>\n<p>At the first <a href=\\"https://automl.cc/\\" target=\\"_blank\\">International Conference on Automated Machine Learning</a> (AutoML), we presented <a href=\\"https://www.amazon.science/publications/syne-tune-a-library-for-large-scale-hyperparameter-tuning-and-reproducible-research\\" target=\\"_blank\\">Syne Tune</a>, an <a href=\\"https://github.com/awslabs/syne-tune\\" target=\\"_blank\\">open-source library</a> for large-scale hyperparameter optimization (HPO) with an emphasis on enabling reproducible machine learning research. It simplifies, standardizes, and accelerates the evaluation of a wide variety of HPO algorithms.</p>\\n<p>These algorithms are implemented on top of common modules and aim to remove implementation bias to enable fair comparisons. By supporting different execution backends, the library also enables researchers and engineers to effortlessly move from simulation and small-scale experimentation to large-scale distributed tuning on the cloud.</p>\n<p>In this post, we will give an overview of the execution backends supported in Syne Tune and benchmark state-of-the-art asynchronous HPO algorithms, including transfer learning baselines.</p>\n<h5><a id=\\"Supported_execution_backends_16\\"></a><strong>Supported execution backends</strong></h5>\\n<p>Syne Tune provides a general interface for backends and three implementations: one to evaluate trials on a local machine, one to evaluate trials in the cloud, and one to simulate tuning with tabulated benchmarks to reduce run time. Switching between different backends is a matter of simply passing a different trial_backend parameter to the tuner, as shown in the code examples below. The backend API has been kept lean on purpose, and adding new backends requires little effort.</p>\n<h5><a id=\\"Local_backend_19\\"></a><strong>Local backend</strong></h5>\\n<p>This backend evaluates trials concurrently on a single machine by using subprocesses. We support rotating multiple GPUs on the machine, assigning the next trial to the least busy GPU (e.g., the GPU with the fewest number of trials currently running). Trial checkpoints and logs are stored to local files.<br />\\n<img src=\\"https://awsdevweb.s3.cn-north-1.amazonaws.com.cn/b98adb00eb284127907d41d86c7737b8_image.png\\" alt=\\"image.png\\" /><br />\\nHow to tune a training script with Bayesian optimization in Syne Tune.</p>\n<h5><a id=\\"Cloud_backend_23\\"></a><strong>Cloud backend</strong></h5>\\n<p>Running on a single machine limits the number of trials that can run concurrently. Moreover, neural-network training may require many GPUs, even distributed across several nodes, or multi-GPU devices. For those use cases, we provide an Amazon SageMaker backend that can run multiple trials in parallel.<br />\\n<img src=\\"https://awsdevweb.s3.cn-north-1.amazonaws.com.cn/b5e2ac9c4f084f55b1678b01f52ca48c_image.png\\" alt=\\"image.png\\" /><br />\\nChanging the trial backend to run on cloud machines.</p>\n<h5><a id=\\"Simulation_backend_27\\"></a><strong>Simulation backend</strong></h5>\\n<p>A growing number of tabulated benchmarks are available for HPO and neural-architecture-search (NAS) research. The simulation backend allows the execution of realistic experiments with such benchmarks on a single CPU instance, paying real time for the decision-making only.</p>\n<p>To this end, we use a timekeeper to manage simulated time and a priority queue of time-stamped events (e.g., reporting-metric values for running trials), which work together to ensure that interactions between trials and the scheduler happen in the right ordering, whatever the experimental setup may be. The simulator correctly handles any number of workers, and delay due to model-based decision-making is taken into account.</p>\n<p><img src=\\"https://awsdevweb.s3.cn-north-1.amazonaws.com.cn/29bd9feddf314adfb0ed460b09b9a3ea_image.png\\" alt=\\"image.png\\" /><br />\\nChanging the trial backend to run on parallel and asynchronous simulations based on tabulated benchmarks.</p>\n<h5><a id=\\"Comparing_asynchronous_tuning_algorithms_34\\"></a><strong>Comparing asynchronous tuning algorithms</strong></h5>\\n<p>Syne Tune provides implementations of a broad range of synchronous and asynchronous HPO algorithms. In our experiments, we consider single-fidelity HPO algorithms, which require entire training runs to evaluate a candidate hyperparameter configuration. Random search (<a href=\\"https://www.jmlr.org/papers/v13/bergstra12a.html\\" target=\\"_blank\\">RS</a>), regularized evolution for architecture search (<a href=\\"https://arxiv.org/abs/1802.01548\\" target=\\"_blank\\">REA</a>), and Bayesian-optimization variants (e.g., Gaussian-process-based (<a href=\\"https://papers.nips.cc/paper/2012/hash/05311655a15b75fab86956663e1819cd-Abstract.html\\" target=\\"_blank\\">GP</a>) and density-ratio-based (<a href=\\"http://proceedings.mlr.press/v139/tiao21a.html\\" target=\\"_blank\\">BORE</a>), of which <a href=\\"https://papers.nips.cc/paper/2011/hash/86e8f7ab32cfd12577bc2619bc635690-Abstract.html\\" target=\\"_blank\\">TPE</a> is a special case) fall in this category.</p>\\n<p>We also consider multi-fidelity HPO algorithms, which stop unpromising training runs early. The median stopping rule (<a href=\\"https://static.googleusercontent.com/media/research.google.com/en/pubs/archive/46180.pdf\\" target=\\"_blank\\">MSR</a>), asynchronous successive halving (<a href=\\"https://arxiv.org/abs/1810.05934\\" target=\\"_blank\\">ASHA</a>), and asynchronous Bayesian-optimization variants (e.g., <a href=\\"https://proceedings.mlr.press/v80/falkner18a.html\\" target=\\"_blank\\">BOHB</a> and <a href=\\"https://arxiv.org/abs/1905.04970\\" target=\\"_blank\\">MOB</a>) are prominent examples.</p>\\n<p>The table below shows the normalized rank, averaged over wall-clock time, of these single- and multi-fidelity optimizers on three publicly available neural-architecture-search benchmarks: FCNet, from <a href=\\"https://arxiv.org/abs/1905.04970\\" target=\\"_blank\\">Klein and Hutter (2019)</a>; NAS201, from <a href=\\"https://arxiv.org/abs/2001.00326\\" target=\\"_blank\\">Dong and Yang (2020)</a>; and LCBench, from <a href=\\"https://arxiv.org/abs/2006.13799\\" target=\\"_blank\\">Zimmer et al. (2021)</a>.</p>\\n<p>Multi-fidelity algorithms are in general superior to single-fidelity algorithms, which is expected, as they make more efficient use of the computational resources available to them. These results are also consistent with previous results reported in the literature. It should be noted that among the multi-fidelity algorithms, MSR is the only one not using successive halving, and it performs worst.<br />\\nThe table also shows the average normalized rank of transfer learning approaches. Hyperparameter transfer learning uses evaluation data from past HPO tasks in order to warmstart the current HPO task, which can result in significant speed-ups in practice.</p>\n<p>Syne Tune supports transfer-learning-based HPO via an abstraction that maps a scheduler and transfer learning data to a warmstarted instance of the former. We consider the bounding-box and quantile-based ASHA, respectively referred to as <a href=\\"https://dl.acm.org/doi/10.5555/3454287.3455431\\" target=\\"_blank\\">ASHA-BB</a> and <a href=\\"https://proceedings.mlr.press/v119/salinas20a.html\\" target=\\"_blank\\">ASHA-CTS</a>. We also consider a zero-shot approach (<a href=\\"https://ieeexplore.ieee.org/document/7373431\\" target=\\"_blank\\">ZS</a>), which greedily selects hyperparameter configurations that complement previously considered ones, based on historical performances; and <a href=\\"https://arxiv.org/abs/2103.16111\\" target=\\"_blank\\">RUSH</a>, which warmstarts ASHA with the best configurations found for previous tasks. As expected, we find that transfer learning approaches accelerate HPO.<br />\\n<img src=\\"https://awsdevweb.s3.cn-north-1.amazonaws.com.cn/da6a661aedd14662a8c18ff7c4f48d21_image.png\\" alt=\\"image.png\\" /><br />\\nAverage normalized rank (lower is better) of algorithms across time and benchmarks. Best results per category are indicated in bold.<br />\\nOur experiments show that <a href=\\"https://github.com/awslabs/syne-tune\\" target=\\"_blank\\">Syne Tune</a> makes research on automated machine learning more efficient, reliable, and trustworthy. By making simulation on tabulated benchmarks a first-class citizen, it makes hyperparameter optimization accessible to researchers without massive computation budgets. By supporting advanced use cases, such as hyperparameter transfer learning, it allows better problem solving in practice.</p>\\n<p>To learn more about the library and contribute to it, please check out the <a href=\\"https://www.amazon.science/publications/syne-tune-a-library-for-large-scale-hyperparameter-tuning-and-reproducible-research\\" target=\\"_blank\\">paper</a> and our GitHub repo for <a href=\\"https://github.com/awslabs/sagemaker-tune\\" target=\\"_blank\\">documentation</a>. We just released the 0.3 version, with new HPO algorithms, new benchmarks, tensorboard visualization, and more.</p>\\n<p>ABOUT THE AUTHOR</p>\n<h4><a id=\\"Cedric_Archambeauhttpswwwamazonscienceauthorcedricarchambeau_52\\"></a><strong><a href=\\"https://www.amazon.science/author/cedric-archambeau\\" target=\\"_blank\\">Cedric Archambeau</a></strong></h4>\n<p>Cédric Archambeau is a principal applied scientist with Amazon Web Services.</p>\n"}